Best Poster Award for Joe Abbott
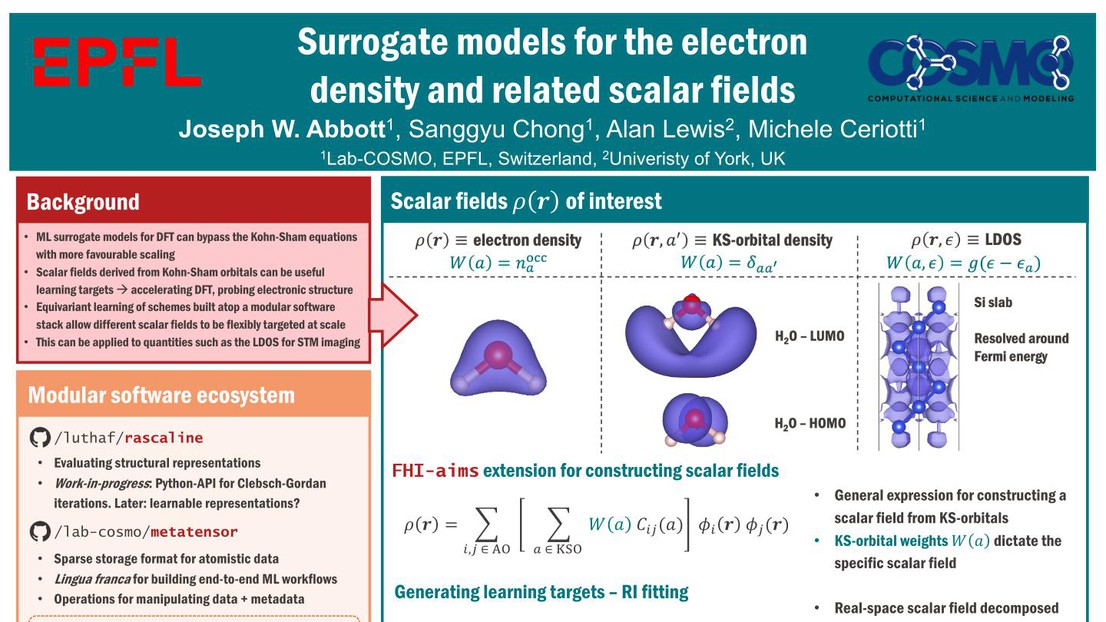
© 2023 EPFL
Congratulations to Joe Abbott from COSMO Lab for the best poster award at the Machine-Learning Interatomic Potentials School (MLIP-2023) that took place at Aalto University from 6th to 10th November!
At this years MLIP-2023 workshop Joe Abbott won the best poster award for his work on "Surrogate models for the electron density and related scalar fields".
The electron density is a central quantity in electronic structure calculations and a fundamental property of molecules and materials. With it, one can in principle access any ground state electronic property. Density-functional theory (DFT) is an ab initio method that calculates the electron density of a system by solving self- consistently the Kohn-Sham equations. However, the cubic scaling of this method in the number of electrons of a system makes the calculations for large systems intractable. In the field of atomistic machine learning (ML), there has been effort put into the development of surrogate models that are able to directly predict the self-consistent electron density at a fraction of the cost of DFT and with more favourable linear scaling. One framework in particular focuses on learning the coefficients of basis functions fitted to the real-space density, using a equivariant structural descriptors. Previous approaches within the "density-fitting framework" solved closed- form analytical solutions to train ML surrogate models. A move towards a gradient-based learning approach allows models of arbitrary complexity to be trained using gradient-descent and backpropagation.
An end-to-end pipeline for the training and prediction of the electron density under this new framework is presented here, built on top of a modular stack of scientific software. With this, access to the electron density of increasingly large systems is possible. This so-called "bypassing of the Kohn-Sham equations" may allow for acceleration of electronic structure calculations, or investigation of larger-scale electronic phenomena not possible with current approaches. Furthermore, this framework is in principle able to learn the coefficients of any scalar field decomposed onto a fitted atom-centered basis. With this, we explore learning targets beyond the total electron density, such as the local density of states. This opens the possibility of applying the infrastructure to interesting scientific problems, such as ML-driven imaging of scanning tunneling microscopy (STM).
