Zeno Karl Schindler Award - 2022 - Benjamin Kellenberger
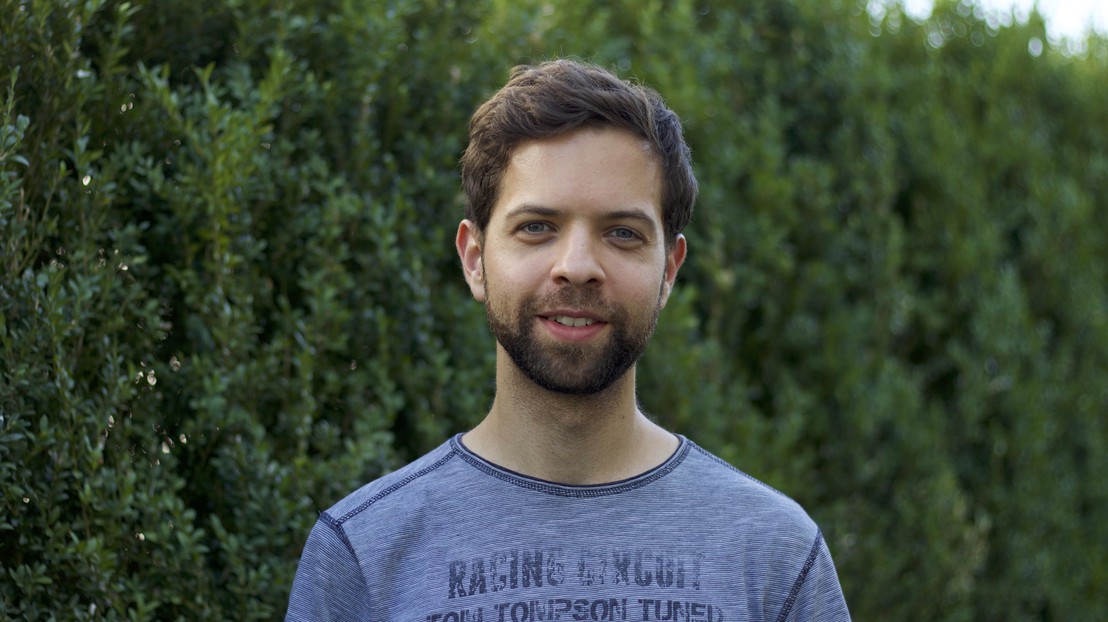
© 2022 EPFL
Interactive Machine Vision for Wildlife Conservation
For his important contributions in digital animal conservation, marrying advances in machine learning, aerial remote sensing and animal ecology. His efforts allowed building an interdisciplinary community at the interface between engineering technologies and the preservation of biodiversity.
The world is increasingly confronted with biodiversity-related issues, including species extinctions and habitat degradation due to climate change and other, human-induced causes. We need to monitor our ecosystem at scale to be able to protect species and understand their interactions within their environment. I propose to use image-based remote sensing and machine learning to do so. We can nowadays observe the Earth at large and detail, using imaging platforms like drones and satellites. Thanks to advances in machine learning, we can further make sense of this data at scale. Hence, I first developed methods that directly detect endangered animals in drone imagery, allowing censuses of individuals over large areas with minimal effort. I subsequently developed "AIDE", the Annotation Interface for Data-driven Ecology, which allows users to employ, optimise, and share such models to their own needs with no coding requirements. Both initiatives have significantly advanced wildlife censuses in accuracy, efficiency, and safety. Finally, I drew perspectives towards a fully data-science driven landscape of wildlife conservation, going beyond mere detections and into species and environmental characterisations, and encouraging ecology and machine learning communities to work together and protect wildlife at large.