What can Wikipedia tell us about human interaction?
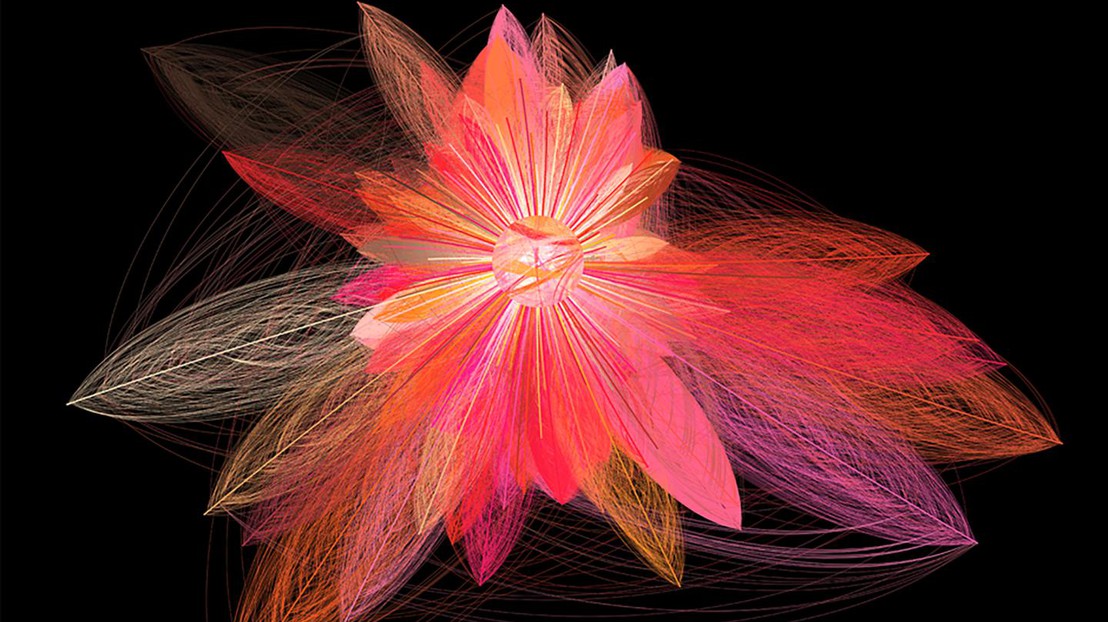
In this data visualization, each node represents a group of Wikipedia pages on a subject related to world events of 2015. Petals are formed by grouping nodes from a given topic © 2016 Kirell Benzi
EPFL researchers have studied the dynamics of network structures using one of the world’s most-visited websites: Wikipedia. In addition to a better understanding of online networks, their work brings exciting insights into human social behavior and collective memory.
Have you ever visited a Wikipedia page to answer a question, only to find yourself clicking from page to page, until you end up on a topic wildly different from the one you started with? If so, not only are you not alone, but chances are that other people have taken the same roundabout route from, say, “Game of Thrones” to “Dubrovnik” to “tourist attraction” to “world’s biggest ball of twine”.
Researchers in the Signal Processing Laboratory (LTS2) led by Professor Pierre Vandergheynst in the EPFL School of Engineering (STI) and School of Computer and Communication Sciences (IC) wanted to find out how this process works.
More specifically, they set out to study the dynamics of network structure using signal processing and network theory, developing an algorithm to automatically detect unusual activity in constantly changing, complex systems like Wikipedia.
“The brain of humanity”
The ability to detect and study anomalous events in online networks – for example, a sudden spike in the number of visits to a particular Wikipedia page over a certain period of time – could tell us a lot about human interaction, collective behavior, memory and information exchange, the researchers say.
“Our idea was to imagine Wikipedia as the brain of humanity, where page visits are comparable to spikes in brain activity,” says Volodymyr Miz, a researcher and PhD student in the LTS2. Miz is the lead author on an article about the new algorithm, which was recently presented at The Web Conference 2019 in San Francisco, California, USA.
Co-author Kirell Benzi, a former LTS2 researcher and EPFL data visualization lecturer now working as a data artist, added that what made Wikipedia so appealing as a data source was its accessibility and size.
“Wikipedia has some 5 billion visits per year for English alone. With this technique, we can identify groups of pages that belong together,” he said.
From collective memory to fake news
The researchers’ algorithm is unique because it can not only identify such anomalous events, but also provide insights into exactly where, how, and why they happened.
“The core difference is that we provide more context due to the network structure. For example, if we look at Wikipedia pages about the 2015 Paris terrorist attacks, we can see that the page about the attack is directly connected to the page about Charlie Hebdo magazine, and also to a cluster of pages representing terrorist organizations,” Miz explains.
Benzi and Miz call this kind of information-seeking “collective memory”, as it can reveal how current events trigger memories of the past.
“The Wikipedia research is about trying to explore new findings about human nature itself. Wikipedia is a very interesting dataset because it reflects more or less what we as humanity decide to remember. Collectively, we have the same train of thought and browse the same topics,” Benzi says.
So, what topics do people most care about, according to this research? In short: other people.
“Some 80% of visits are for entertainment or celebrities. In past research, we've found that 40% of all links that are clicked are about people and their relationships,” Benzi says, adding that fewer than 1% of visits are for topics related to science.
The LTS2 is currently collaborating with developers of the free offline web browser Kiwix, which aims to bring compressed versions of Wikipedia to those without free access to the internet.
“Our method could be very helpful to Kiwix to help identify and compress only relevant portions of Wikipedia, based on language and culture, for example,” Miz says.
Other applications of the algorithm could include studying the spread of fake news on Twitter by monitoring spikes in retweets, or understanding links between email network dynamics and real-world events. However, these topics are more challenging to study than Wikipedia due to smaller amounts of freely available data.
Case study: Game of Thrones
Miz, Benzi and their colleagues used their method to detect anomalous activity on Wikipedia pages related to the final season of the HBO hit show Game of Thrones as an example. The resulting open dataset allowed them to create data visualizations of pages relating to different aspects of the show, including actors, characters, seasons, episodes, and other topics.
The researchers were also able to use the method to determine character popularity based on the number of visits to their Wikipedia pages over time, and are currently trying to see which other pages were activated by the death of a particular character on the show. This work builds on a similar effort in 2016 to analyze the Star Wars universe.
Benzi notes that the research is an excellent example of digital humanities, in which data science methods and digital technologies are applied to sociology, literature, history and other humanities fields.
“Digital humanities is a really interesting as a field, but it only works when you have a combination of different skillsets from data science, engineering, psychology, sociology, art and so on. So, one of the perks is being able to collaborate between labs,” Benzi says.
Follow the development of this research on social networks: @mizvladimir, @KirellBenzi