Virginia Bordignon receives 2023 IEEE Best Dissertation Award
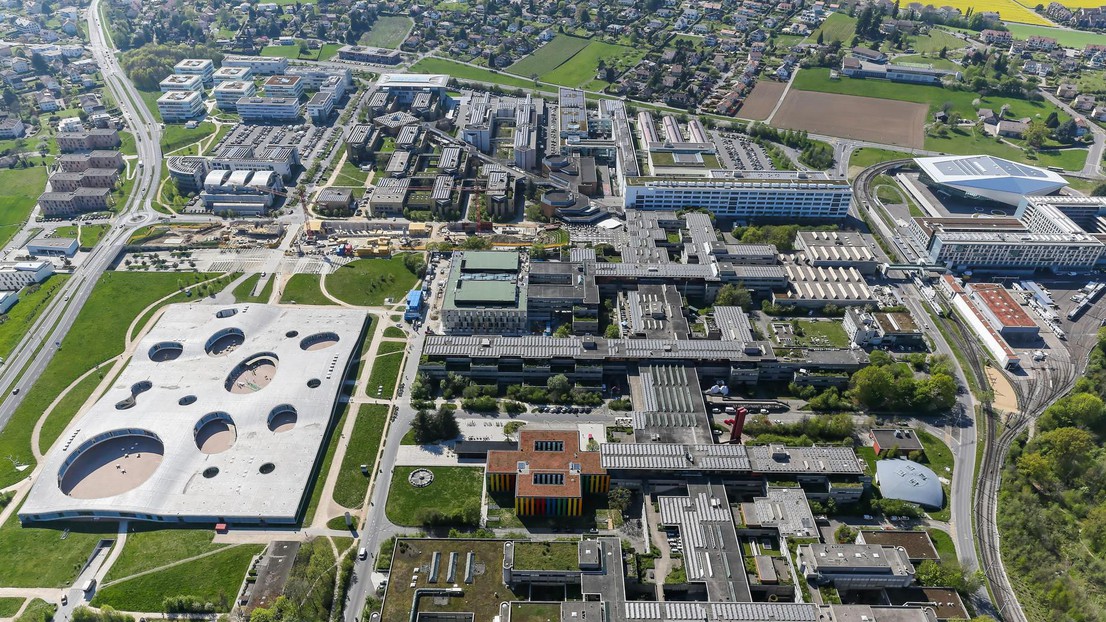
© 2023 EPFL
The Ph.D. dissertation of Virginia Bordignon, entitled Opinion Formation over Adaptive Networks, has been selected to receive the 2023 Best Dissertation Award from the IEEE Signal Processing Society.
Dr. Bordignon received her Ph.D. in Electrical Engineering in 2022 at EPFL under the supervision of Prof. Ali H. Sayed in the Adaptive Systems Laboratory. This award recognizes Ph.D. dissertations for their scientific impact on the discipline and for their overall quality in terms of creativity, novelty, rigor, and timeliness. Dr. Bordignon will receive the award at the IEEE Signal Processing Society Awards ceremony to be held in Seoul, South Korea, in April 2024.

According to her Ph.D. supervisor Prof. Sayed, “Virginia’s dissertation is of singular quality in terms of topic, quality, and depth. Her dissertation did not only solve important open problems in social learning in an elegant and mathematically deep manner, but it has also launched new directions of research that are motivating the works of several other Ph.D. students."
Dr. Bordignon’s dissertation deals with the topic of social learning over graphs. Social learning involves a collection of agents that are usually dispersed over some geographic region. The agents exchange opinion about a particular topic, or observe some state of nature. For example, the agents may be observing together some bird and wish to agree on its color, or they may be observing some machine and wish to decide whether it is operating safely or not. Since agents may be viewing the object from different perspectives or angles, the information available to each individual agent may not be sufficient for them to arrive at an informed decision on their own. By working together, and by sharing information among neighbors, agents are able to reach consensus and agree on a common characteristic (such as the color of the bird in this example or the safety condition of a machine). While this is a simple example, the state-of-nature can refer to any other scenario, such as deciding on the weather condition (rainy, sunny, cloudy), deciding on eminent danger, agreeing on an opinion, tracking opinion dynamics, tracking targets, etc. This formulation is key to the solution of decision-making problems by networked agents and is useful in many scenarios.
Dr. Bordignon’s work was able to show how to incorporate adaptation and learning into the popular Bayes’ rule in order to enable socially intelligent interactions over graphs. By doing so, she was able to propose a new class of social learning algorithms that are able to track changes in the underlying models, respond faster to drifts in opinions, counter the stubbornness of agents in social networks, and enable continuous learning. In her work, she had to rely on advanced probability and mathematical tools such as convergence of random series, unconventional central limit theorems, and large deviations analysis. She has published well from her work, including 5 journal articles in the IEEE Trans. Information Theory and 1 journal article in the IEEE Trans. Signal Processing.
Dr. Bordignon is currently a post-doctoral scholar at the Adaptive Systems Laboratory at EPFL School of Engineering.