Using a camera to spot and track drones
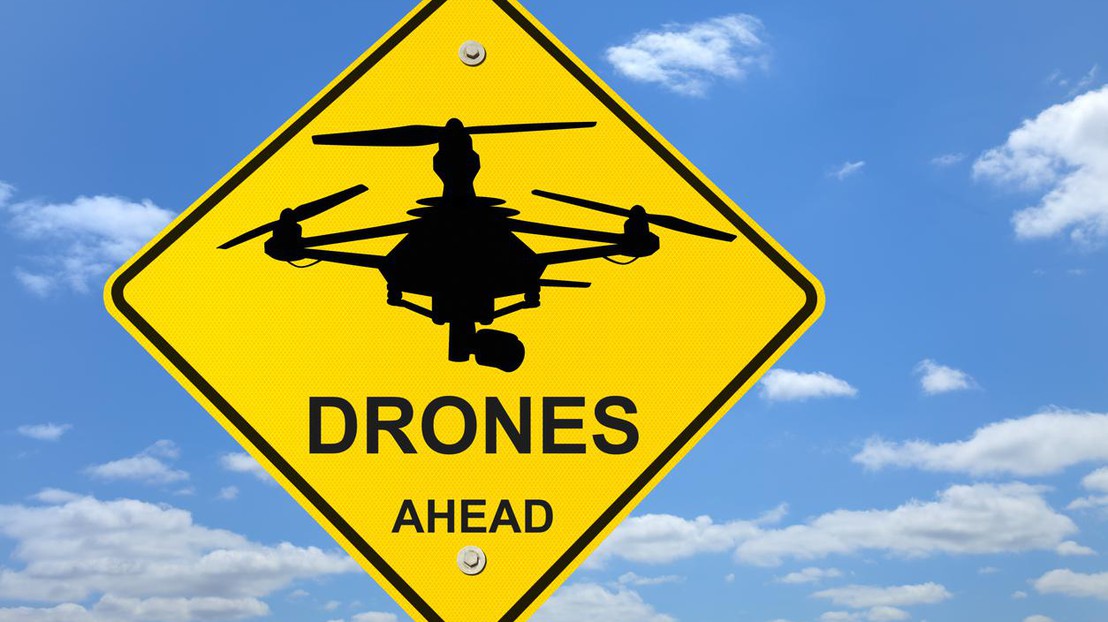
EPFL researchers have developed algorithms capable of detecting and tracking small flying objects using a simple camera. ©istock
EPFL researchers have shown that a simple camera can detect and track flying drones. Plus, the lightweight, energy-efficient and inexpensive technology could be installed directly on the drones themselves and enhance safety in the skies.
The rising number of drones in air space poses numerous challenges. Topping that list is our ability to simply detect these small unmanned aerial vehicles. Periodic near-misses between drones and large airplanes raise the specter of disaster, and the drones themselves often lack the necessary technology to locate other moving objects. To address these issues, EPFL researchers have developed algorithms capable of detecting and tracking small flying objects using a simple camera. The proof of concept was conducted as part of a PhD dissertation, and a real-time detection and collision avoidance system is now being developed in a project funded by the Commission for Technology and Innovation (CTI).
Today’s collision avoidance systems operate actively: an airplane in flight calculates its position, altitude and course, and communicates this information to other aircraft using the same technology. Those aircraft can then evaluate the risk of a collision based on their own positioning data and, if necessary, alert the pilot. But this system is only effective as long as all aircraft are equipped with the same technology. In reality, drones often lack such systems, which are costly and heavy and consume more power.
Artificial intelligence and Deep learning
A camera can thus be an effective, non-cooperative (i.e., not every aircraft must be equipped with it) addition to that system, provided the camera can successfully detect a flying drone. Therein lay the obstacle that researchers at EPFL's Computer Vision Laboratory (CVLAB) sought to overcome. The biggest challenge for a moving camera is to spot another moving object. This is much more difficult on a drone than it is on a car, which only moves in two dimensions. Drones move in three dimensions, and the camera is called on to detect objects against the sky or the ground, depending on the angle of sight. Plus, drones need to locate objects as quickly as possible, such as when they are still fuzzy black dots against a dark forest. And the fact that no two drones look alike anymore – new models are constantly being developed – meant that the researchers had to find a way to teach the camera to recognize all sorts of drones.
In his thesis, Artem Rozantsev showed that these challenges can be overcome. The first step consisted in using artificial intelligence and deep learning to teach the camera to recognize drones. His method combined information on both appearance (types of drones, position, etc.) and motion (movement in the camera's field of view), as neither alone was capable of achieving sufficiently reliable detection. He therefore proposed a machine-learning technique that operates on spatio-temporal cubes of image intensities where individual patches are aligned using a regression-based motion stabilization algorithm.
Real-time performance and accuracy
But the recognition algorithm on its own was not enough. To train a detector to recognize all types of drones in all kinds of positions, it has to have "seen" as many as possible. The existing database of images, however, is limited. So Rozantsev filled in the gaps by generating realistic synthetic images. The generated images, which are based only on a small set of real examples and a coarse 3D model of the object, are used together with the real examples to train the detector. A key ingredient to his method, the generated images are as close as possible to the real ones – not in terms of image quality, but according to the features used by the machine-learning algorithm.
The researchers managed to develop a reliable algorithm capable of detecting a drone using a lightweight camera similar to those found in smartphones. The aim of the project, now financed by the CTI, is to train a detector using an even larger data set to improve its real-time performance and accuracy. EPFL's CVLAB researchers are working on this in collaboration with FLARM Technology AG, the leading supplier of affordable collision avoidance technology for civil aviation. The first commercial models are expected to be released next year.