The random importance of clouds
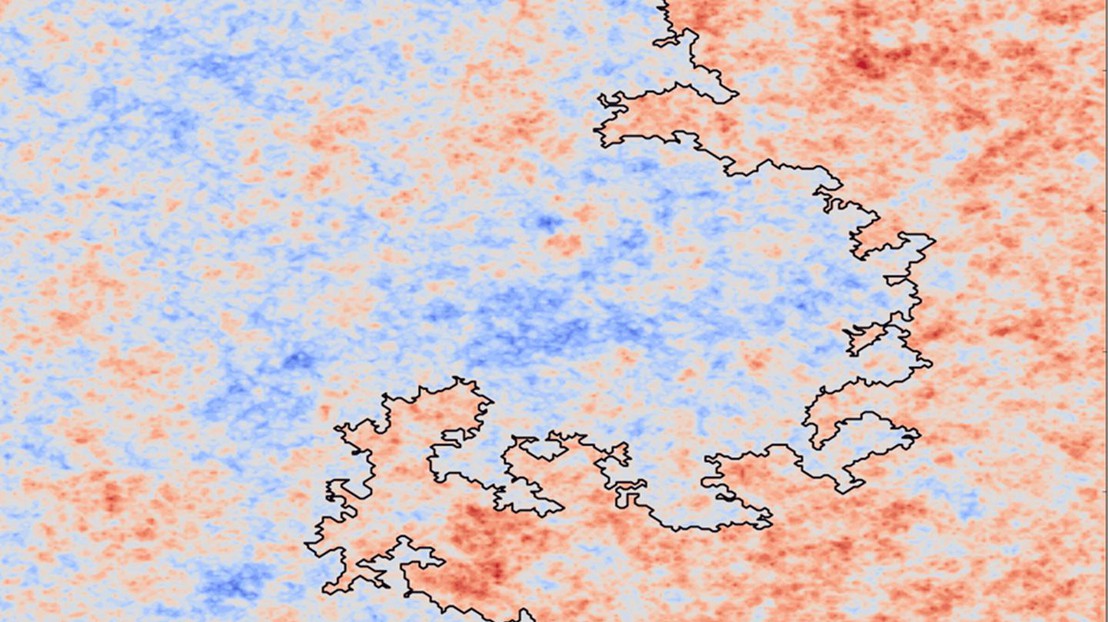
A heat map of the heights of an approximation of the 2D GFF (red is high, blue is low) together with a zero contour line, drawn in black. Credit: Juhan Aru, EPFL
Juhan Aru is a new professor in Mathematics at EPFL where he holds the Chair of Random Geometry. His work aims at understanding geometric properties of models where randomness and geometry meet.
Randomness and geometrical structures interact with one another in a plethora of real-life examples, such as bubbles in boiling water or snowflakes. But Professor Juhan Aru, EPFL’s new Chair of Random Geometry, found a way to link his hobbies with work in another everyday phenomenon: clouds.
“In the north, from where I come from, the summer skies are often filled with small puffy clouds – cumulus clouds,” he says. “You often see them in Switzerland too. One of my favourite pastimes has always been to lie down on a field, a beach – why not a mountaintop? – and to watch these clouds.” Aru considers himself lucky, as his current field of mathematics is actually linked to clouds.
The field Aru is working on is sometimes called "random geometry", where geometric structures and randomness interact. If one looks at cumulus clouds, then a certain structure – or geometry – is clear and apparent to the eye. But even so, randomness is present, hidden in the formation of clouds through the aggregation of water molecules, each flying around the sky independently, randomly, before ultimately “deciding” to contribute to the cloud itself.
Mathematicians don’t have a good, satisfactory mathematical description of cumulus clouds – or at least a model that can describe and understand their detailed and beautiful shapes. For now, we have to work on simpler things, e.g. restricting ourselves to two dimensions – or as mathematicians would say, to “planar structures” – some of them still resemble clouds, but two-dimensional clouds.
Aru and his group are trying to understand the mathematical properties of these cloud-like planar structures. Their work is similar to that of a biologist, who taxonomizes different species – classifying them, and describing their distinctive and common properties.
One of the models Aru works with is called the “2D Gaussian free field” (2D GFF; see an example in the figure) We can think of it as the height function of a mountainous landscape, like the Alps.
A surface plot of an approximation of the 2D Gaussian free field. Credit: Juhan Aru, EPFL.
“I try to understand the peaks, the valleys, and the level lines of this landscape,” says Aru. “It turns out that the 2D GFF has many connections to models of statistical physics, to quantum field theories, to simplified theories of quantum gravity, and also to what is called ‘Brownian motion’.” Brownian motion is a probabilistic model used in mathematics to describe the random motion of particles. “Errantly moving particles are like water molecules in the summer sky,” explains Aru. “So, at least for me, the GFF is also linked to clouds”.
The past few years have seen remarkable progress in the field, and now Aru and his colleagues have been able understand further aspects of the 2D GFF. “Yet, the work is by no means done,” says Aru. "Indeed, many connections of the GFF are still being uncovered. For example, at the moment we have no mathematical means to study geometric questions in three dimensions; and yet, clouds are three-dimensional. So the questions are challenging, and inspiration is important - so I often go for a walk, lie down, and take a look at the sky!”
The Gaussian free field (GFF) is a random height function. It is different every time you look at it, but even so there are some geometric properties that are (almost) always true. Therefore, when we study a 2D GFF, we are interested in describing its statistical properties. Together with co-authors, the Chair of Random Geometry has been studying such properties.
The difficulty is that the 2D GFF fluctuates so much that you cannot assign a value to it at any point – mathematically speaking, at any point the random height has infinite variance. One can only ask about averages of the height around any point. Mathematically, this means that the 2D GFF can be only be described as a random generalized function, as a random Schwartz distribution, which makes the study of geometric properties more difficult – how do you even give a sense to for example contour lines, when the height-function has no point-wise values?
Nevertheless, around 15 years ago Oded Schramm and Scott Sheffield found a way to describe the level lines of the 2D GFF and since then there has been tremendous progress in the subject. Part of the progress has been in giving sense to a wider variety of geometric notions, e.g. excursions off the level lines.
Another part of the progress has been related to the many applications of the GFF: it helps construct 2D toy models of quantum gravity, called Liouville quantum gravity, and to better understand Schramm–Loewner evolution processes – a family of random curves that describes the geometry of many statistical physics models, e.g. those of long random polymers.