Teasing out the structure of free polyphony
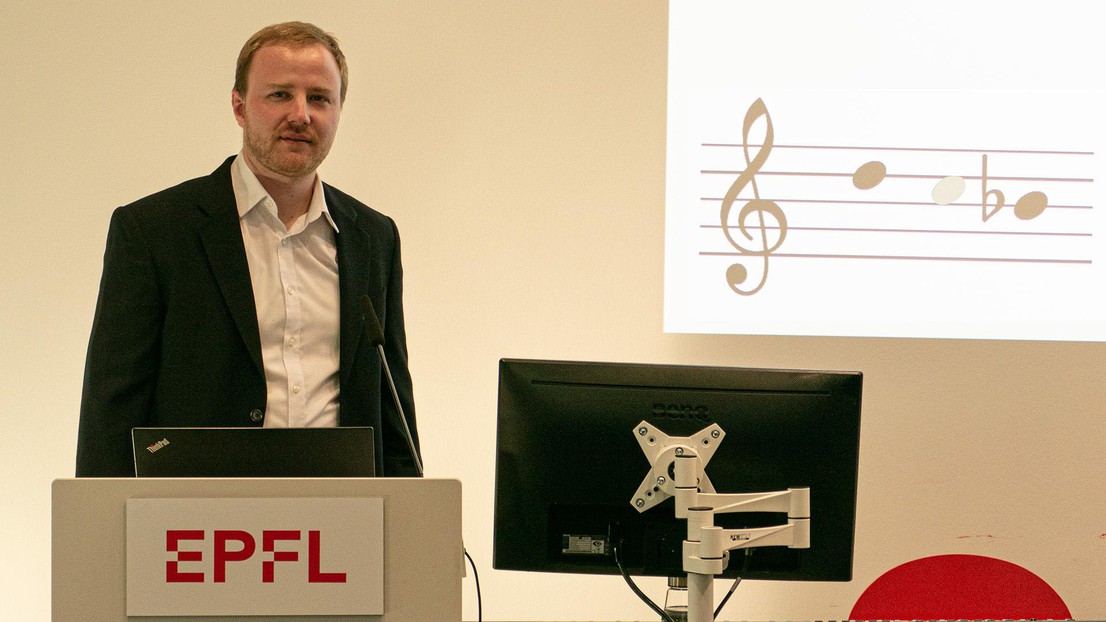
© 2023 EPFL
Christoph Finkensiep used a combination of music theory and computational modeling to shed light on the generative processes behind music.
First impressions can be deceiving. It’s a fact that applies not only to people, places, or paintings but also to music. We naturally try to impose a structure upon the music we listen to, identifying chords, a rhythm, or melodies. But closer observation can reveal hidden complexity that is often difficult to systematize, including independent voices, imperfect rhythms, and ambiguous relationships between neighboring notes.
For his PhD thesis at the Digital and Cognitive Musicology Lab in EPFL’s Digital Humanities Institute, Christoph Finkensiep made it his mission to tease out the structure of free polyphony, music in which groups of notes cannot be clearly classified as contributing to chords or melodies. Bridging the divide between the humanities and “hard science,” his research draws equally on classical music theory and computational analysis. We spoke with Christoph two weeks before his public thesis defense.
What is free polyphony, and how does it differ from the music we might hear on the radio?
(Christoph Finkensiep): - Free polyphony doesn’t necessarily differ from what we hear on the radio; you might hear it in the songs they play. Essentially, free polyphony is a specific way of combining notes not just as a melody over a sequence of chords, but more like several lines that play concurrently and can freely start, stop, or connect with each other. In a way, it’s the default case for music when you don’t have so many constraints.
Free polyphony sounds as if it was defined precisely by a lack of structure, but your PhD thesis is entitled: “The structure of free polyphony.”
- Exactly. The lack of structure makes it complicated, but it’s still a sequence of notes. They might overlap, start at the same time but end at different times, there might be many of them in parallel, or we might simply have a sequence of single notes. Representing the notes in a score is not straightforward, and the structures they form together can be even more complicated. Traditionally people address this by making simplifying assumptions, assuming, for example, that they are dealing with a sequence of chords. But the really interesting stuff happens when that’s not the case. The notes are still related to each other, but we need to figure out which notes are related and how.
What got you interested in this topic?
- I play the trombone and some piano, and I had a bit of music theory background when I started my thesis. When you learn music theory, you learn to apply it using common sense, extending the simple rules to more complex cases. Only that isn’t very explicit, is it? We don’t really know how to do it. That was something that kept bugging me, something that I wanted to address. I wanted to know: can we get to the point where we can teach a computer how to do it?
EPFL is known for many things, but music does not rank highly on that list. What brought you to EPFL?
- I had already written my master’s thesis with Martin Rohrmeier, who now heads EPFL’s Digital and Cognitive Musicology Lab, while he was still in Dresden. So I basically followed him here for my PhD thesis. What’s beautiful about EPFL is that we can combine the humanities side of things – music and cultural phenomena – with all this expertise in computation.
What types of tools do you use to study this type of problem in musicology?
- My work is based on generative models. The piece of music is essentially the end product of what the model generates, and the generative process can be thought of as an “explanation” of the piece. What I try to figure out is: what is a good explanation for the notes in a piece? Or in other words, what happens in the generation process? These models are typically recursive, in the same way that music is often about taking something simple and ornamenting it, making it more and more complex.
On the probabilistic side, it’s about quantifying how plausible a given explanation for a piece might be. Assigning probabilities to the individual steps gives us a probability for each derivation. Then, we can turn around and ask: given this piece, what is the most plausible explanation for it?
What are some of the key findings you uncovered while working on your thesis?
- One of the most interesting things I found was that we do not yet have a good understanding of voice leading, which describes the phenomenon of notes being connected in lines, with notes leading to the notes that follow them. But what do we mean by voices? They could refer to different instruments playing together or different singers singing together. In a famous example, the prelude of Bach’s G major cello suite, it sounds like there are several voices moving together even though it’s just a solo cello line. This is an example of implicit polyphony, where there are several intended voices. So, it’s still unclear what we mean by voices, especially when we don’t have a fixed voice structure.
Does your work tell us anything about how we, as music consumers, make sense of this complexity?
- In a way, yes. Most models I worked with considered ideal cases in which we have a complete derivation of a piece explaining every single note. We typically don’t look at pieces precisely enough to achieve this level of understanding. If an expert spent a lot of time with a piece, they could probably get there. If, however, you tried to use classical parsing mechanisms to find all possible derivations of a piece and pick the best one, well, it would be beyond what you could implement on a computer.
So, what is missing? There is probably some kind of heuristic approach that doesn’t try to find the best solution but simply tries to come up with a good guess. When we listen to a piece, we usually have a first impression of what’s going on. We might recognize familiar patterns or differentiate between styles. Our brains can do this quickly and intuitively.
But then, when you work with a piece and practice it, you again get a different understanding. So, there isn’t just the immediate perception, but there’s another level that requires spending time with a piece to develop a deeper and deeper understanding.
Where do you see your field of research heading over the next years?
- It could go many ways. Many people in my area work on things like automatic music generation, which would be one of the most obvious applications. But for me, that isn’t very important. My focus is on fundamental research.
So far, I’ve focused on a very specific type of phenomenon. Now I’d like to extend it to other styles to uncover principles that are shared across styles and others that change from one style to the other.
Another question that interests me is relating this to general cognitive phenomena. For example, why do we find these recursive structures in music? Are they exclusive to music? Probably not. We see them in language, vision, and other cognitive domains. They may be a fundamental element of how we think.
And then, there is the computational side, which involves a mix of recursive models, probabilistic models, and ideas related to deep learning and reinforcement learning. With its complex structures that need advanced computational tools to analyze, music is a perfect testbed for developing new computational tools, especially in the direction of explainable and interpretable artificial intelligence.