Talk by Sanjay Chandrasekaran on optimizing recommender systems
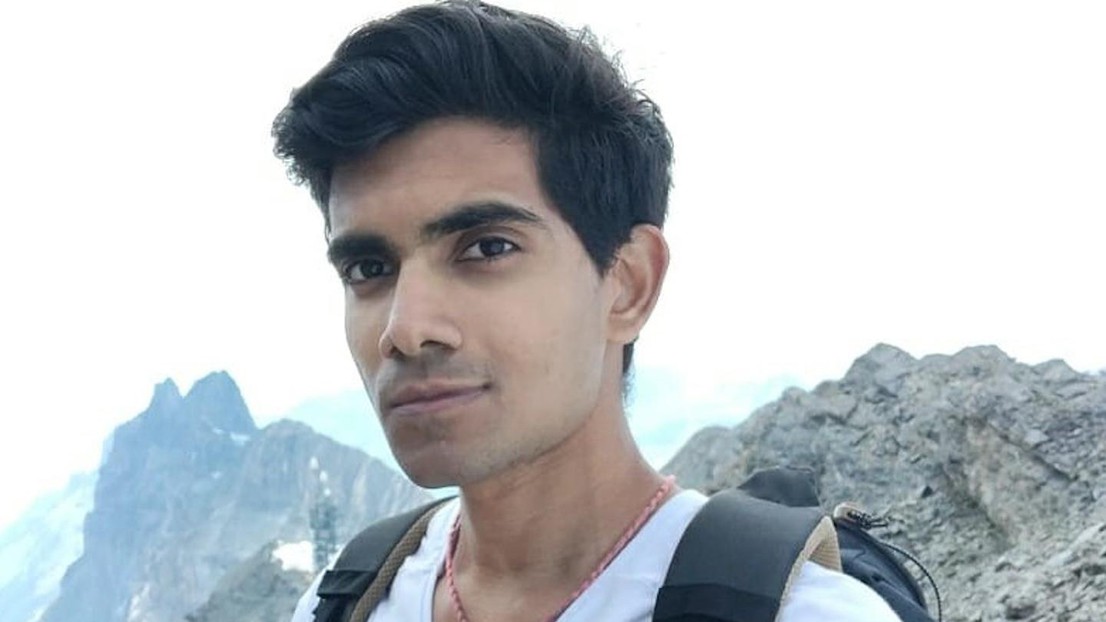
© 2023 EPFL
On 1/12/23, Sanjay Chandrasekaran will give the talk "Optimizing recommender systems for social networks: A
control-theoretic approach". The seminar will start at 11h00 in ME C2 405.
Abstract:
Social media platforms employ recommender systems to engage users with content. The stateof-
the-art methods use brute-force machine learning approaches that combine features
describing personalization, popularity and similarity of interests with other users on the social
platform to provide a set of media feed that the user is most likely to engage with. They provide
feed to the users based on their individual history of interests and similarities with peers.
Conventional recommenders do not take into account the social network explicitly, nor does it
consider a feedback loop between the user opinion evolution and the recommender.
In this talk, I would like to present a control-theoretic perspective of recommenders, wherein
we take the user’s social network into account and identify users with maximum node
centrality, thereby prioritizing advertisements towards them. User interactions, real-time
opinions of users, and user clicking behaviour are assumed unknown for online
recommendations. We address these three challenges from a feedback control point of view:
user interactions are determined using sensitivity estimation, real-time opinions are estimated
using perception-based techniques, and the unknown clicking behaviour is learned using a
combination of supervised learning and zero-order optimization. The proposed scheme allows
us to independently test the effect of users' clicking behaviour, social network topology and
objective function of the recommender over the opinion dynamics. We provide theoretical
performance guarantees and numerically verify the recommender’s efficacy for two tasks:
agenda-driving and engagement maximization. Our recommender shows promising results on
simulation environments and a real dataset, laying the groundwork for future systems-theoryb-ased
recommenders.
Bio: Sanjay Chandrasekaran recieved his B. Tech. (Honors) degree in Instrumentation & Control
Engineering from NIT-Trichy in 2021. He completed his M.Sc. degree in Information Technology
& Electrical Engineering, specializing in systems and control from ETH Zurich in 2023. He carried
out his master thesis at the Automatic Control Laboratory at ETH Zurich under the supervision
of Prof. Dr. Florian Dörfler. Broadly, his research interests are in feedback optimization, control
and estimation for networked systems and in the intersection of learning and control, with the
associated development of performance guarantees and stability bounds. Apart from work,
Sanjay enjoys being active and practices yoga, goes on hikes and via ferrata routes.