Stefan Vlaski receives 2024 Best PhD Dissertation Award from EURASIP
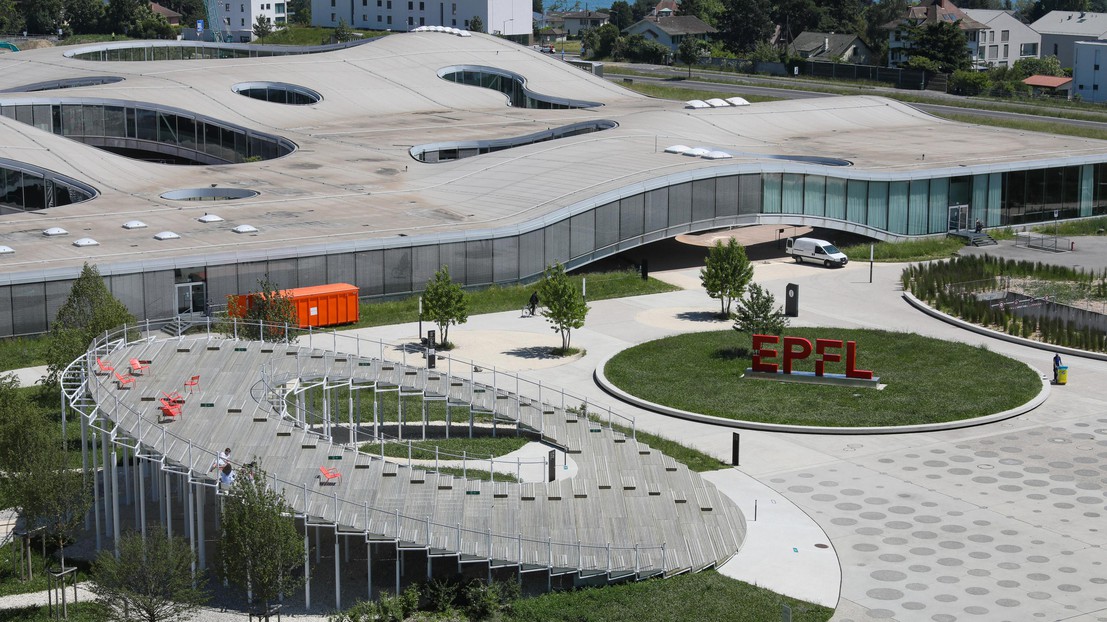
© 2024 EPFL
Stefan Vlaski had received the 2024 Best Ph.D. Dissertation Award from the European Association for Signal Processing (EURASIP) for his dissertation titled “Distributed Stochastic Optimization in Non-Differentiable and Non-Convex Environments.”
Stefan Vlaski started his Ph.D. studies at UCLA in 2014 under the supervision of Professor Ali H. Sayed. He moved with Dean Sayed to EPFL in 2017 as a visiting Ph.D. student where he concluded the work on his UCLA Ph.D. dissertation in 2019 with the title “Distributed Stochastic Optimization in Non-Differentiable and Non-Convex Environments.” Following his graduation, Stefan served for 2 years as a post-doctoral scholar at EPFL in Professor Sayed’s Adaptive Systems Laboratory during 2019-2021. In 2021 he joined Imperial College London as a faculty member.

According to Professor Sayed, “Stefan is a superlative researcher with a rounded and broad understanding of the field. He has made deep contributions to the science of multi-agent systems and adaptive networks.”
One key focus area for Dr. Vlaski’s research has been the study of how adaptive networks learn in nonconvex environments. These scenarios are common in practice, and they arise for example in the training of large and deep neural models. Such neural networks have nonconvex performance surfaces and yet they have been observed to deliver impressive performance in a wide range of applications. In their own way, they can succeed in finding local minima that deliver good performance metrics. Earlier approaches for optimization and learning in nonconvex environments have focused on infusing artificial noise into the update recursions of optimization algorithms to prod them to move away from undesirable saddle locations. However, neural models can sometimes do that without such artifacts.
Stefan had a powerful insight. Stochastic optimization algorithms are known to generate gradient noise by default, due to the random nature of their update relations. And this noise seeps into the dynamics of learning algorithms. Can the presence of such gradient noise alone be sufficient to ensure escape from saddle points without any additional artifacts? In a 2-part paper published in the IEEE Transactions on Signal Processing in 2021, with extensive theoretical analysis, Stefan was able to discover two original results that have pushed our understanding of nonconvex environments to new limits. First, he established conditions under which gradient noise is sufficient to ensure escape from saddle points and, second, he showed that the escape from saddle points occurs in polynomial time. These results form the core of his Ph.D. dissertation. According to his supervisor Sayed, “I consider these results to be so revealing that I have dedicated an entire chapter in my recent textbook on Inference and Learning from Data (Cambridge, 2022) to Stefan’s results.”
Stefan has contributed to many other important research topics as well, including effective algorithms for federated learning, decentralized algorithms for multi-task learning, and algorithms for learning over social graphs. He has published extensively in these domains, with articles appearing in several IEEE journals including IEEE Trans. Signal Processing, IEEE Trans. Information Theory, IEEE Trans. Automatic Control, and others.