SNSF Consolidator Grant pour Prof. Daniel Kuhn
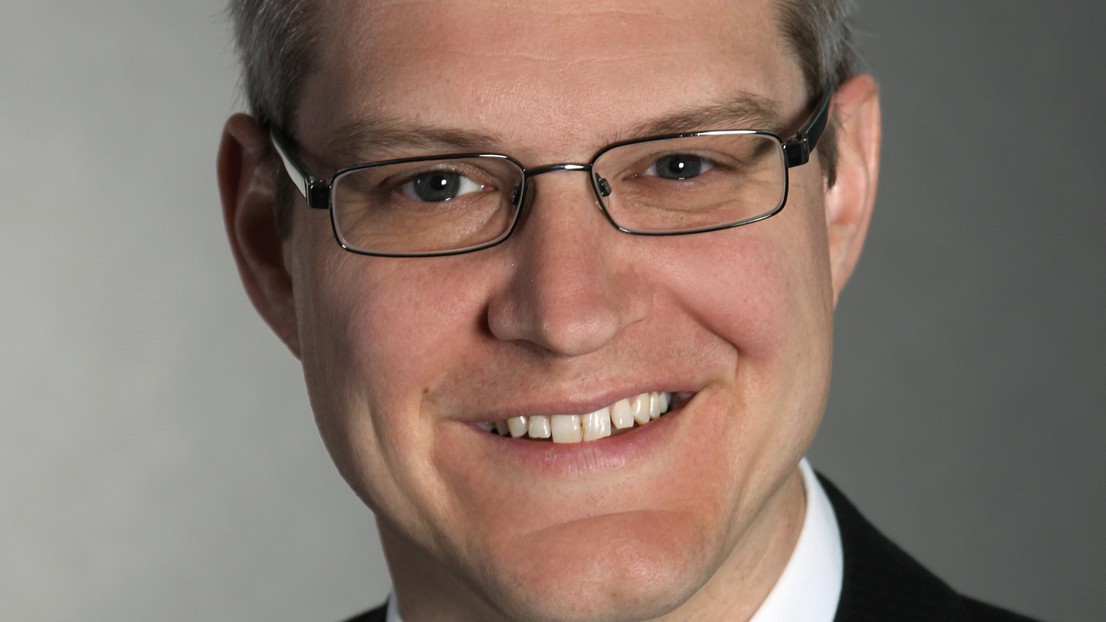
Prof. Daniel Kuhn © 2015 EPFL
Prof. Daniel Kuhn a obtenu un Consolidator Grant pour CHF 1Mio du Fonds National Suisse pour la Recherche Scientifique (FNS) pour son projet intitulé “Optimization under Unknown or Uncertain Uncertainty (Optimization4U)”. Cette bourse s'inscrit dans le cadre de la mesure transitoire "Temporary Backup Schemes" suite à l'exclusion des institutions de recherche suisses des programmes du Conseil européen de la recherche (CER).
Résumé du projet
Uncertainty is traditionally modeled via probability distributions. However, observable statistical data can often be explained by many strikingly different distributions. This "uncertainty about the uncertainty" poses a major challenge for optimization problems with uncertain parameters: estimation errors in the parameters' distribution are amplified through the optimization process and lead to biased (overly optimistic) optimization results as well as post-decision
disappointment in out-of-sample tests.
The emerging field of distributionally robust optimization (DRO) seeks new optimization models whose solutions are optimized against all
distributions consistent with the given prior information. Recent breakthrough work has shown that many DRO models can be solved in
polynomial time even when the corresponding stochastic models are intractable. DRO models also offer a more realistic account of
uncertainty and mitigate the post-decision disappointment characteristic of stochastic models.
This project will advance DRO as a dominant modeling paradigm for optimization under uncertainty and aims to lay the foundations for
industry-size applications. We endeavor to make progress along four directions. (i) Distributions: We will use Choquet theory to
significantly extend the class of distribution families that can be handled in a DRO framework. (ii) Dynamics: We will leverage modern
decision rule techniques to solve dynamic DRO models without incurring a curse of dimensionality. (iii) Decision Support: We plan to develop a
tailor-made modeling language and open-source software to make DRO methods accessible to decision-makers without expert knowledge in
optimization. (iv) Dimensionality: As a guiding principle, we will use tractable conservative approximations whenever necessary to guarantee
that all emerging DRO models remain polynomial-time solvable and thus have the potential to scale to industrially relevant problem sizes.