"SINDER: Repairing the Singular Defects of DINOv2" at ECCV 2024
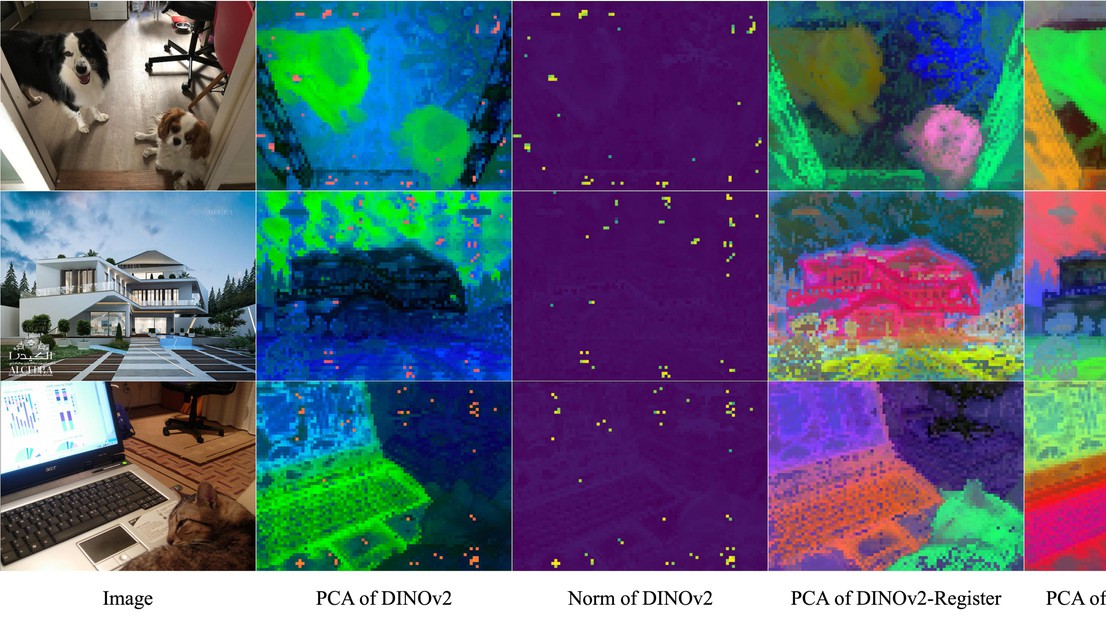
© 2024 EPFL
Our paper "SINDER: Repairing the Singular Defects of DINOv2" was accepted at the 2024 European Conference on Computer Vision (ECCC 2024)
Our paper "SINDER: Repairing the Singular Defects of DINOv2" was accepted at the 2024 European Conference on Computer Vision (ECCC 2024).
Authors: Haoqi Wang, Tong Zhang (IVRL), Mathieu Salzmann
Vision Transformer models trained on large-scale datasets, although effective, often exhibit artifacts in the patch token they extract. While such defects can be alleviated by re-training the entire model with additional classification tokens, the underlying reasons for the presence of these tokens remain unclear. In this paper, we conduct a thorough investigation of this phenomenon, combining theoretical analysis with empirical observations. Our findings reveal that these artifacts originate from the pre-trained network itself, specifically stemming from the leading left singular vector of the network's weights. Furthermore, to mitigate these defects, we propose a novel fine-tuning smooth regularization that rectifies structural deficiencies using only a small dataset, thereby avoiding the need for complete re-training. We validate our method on various downstream tasks, including unsupervised segmentation, classification, supervised segmentation, and depth estimation, demonstrating its effectiveness in improving model performance. Codes and checkpoints are available at https://github.com/haoqiwang/sinder.
arXiv link: https://arxiv.org/abs/2407.16826