Quantum Magnetism on the Pyrochlore Lattice
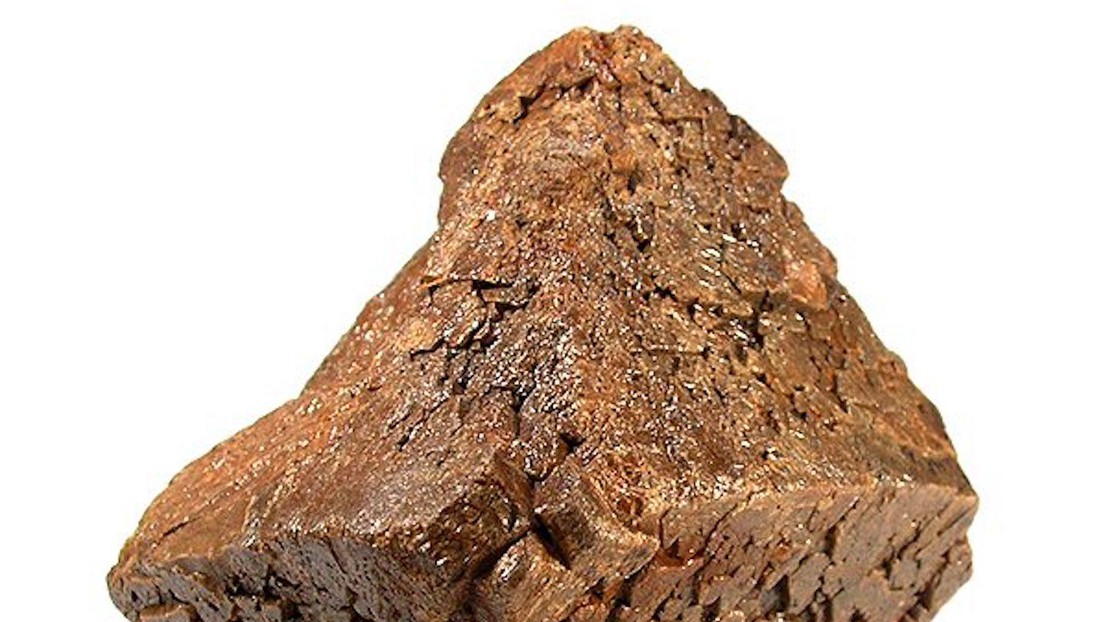
Rob Lavinsky, iRocks.com – CC-BY-SA-3.0, CC BY-SA 3.0 <https://creativecommons.org/licenses/by-sa/3.0>, via Wikimedia Commons
A collaboration between CQSL and the University of Zurich has studied the elusive properties of quantum magnets on the Pyrochlore Lattice. The work, now published in Physical Review X, is based on extensive numerical simulations relying on novel ways to represent complex quantum wave functions, including deep convolutional neural networks.
Quantum magnets are materials in which spins arranged in a crystal lattice interact with each other. At low temperature, these spins often assume some type of order, such as collinear ferromagnetic ordering. However, materials known as quantum spin liquids avoid such ordering even down to the lowest temperatures, retaining their quantum fluctuations. Such materials are highly sought after because of their potential applications in quantum computing. Promising candidates include materials in which the atoms are arranged in a so-called “pyrochlore lattice,” a 3D network of corner-sharing tetrahedra. Its most frustrated Heisenberg regime, where spins interact with only their nearest neighbors in a “very quantum” way, has been inaccessible to theoretical study to date, and whether this model can host a quantum spin liquid is not yet settled. The work by CQSL and University of Zurich, contrary to many expectations, gives a negative answer.
An important aspect of this research is that the conclusions are reached thanks to extensive numerical simulations based on novel ways to represent the complex quantum wave functions including deep convolutional neural networks. The neural-network based approaches are those developed at CQSL, and are publicly available through the NetKet open-source project. Overall, this combination of numerical techniques has allowed to simulate previously inaccessible system sizes and find an ordered ground state in which spins bond pairwise into dimers (local crystals) rather than forming a liquid.
Beyond this concrete physical result, this work is the first application of neural network quantum states to solve 3D quantum many-body systems. Such systems are a frontier for numerical simulations, as previously existing techniques were better suited for lower dimensions. This work, on the contrary, showcases a novel way of obtaining high-quality solutions of quantum problems in three dimensions.
"Broken-Symmetry Ground States of the Heisenberg Model on the Pyrochlore Lattice" by Nikita Astrakhantsev, Tom Westerhout, Apoorv Tiwari, Kenny Choo, Ao Chen, Mark H. Fischer, Giuseppe Carleo, and Titus Neupert, Phys. Rev. X 11, 041021 (2021)