Pioneering Algebraic Topology in the Blue Brain Project
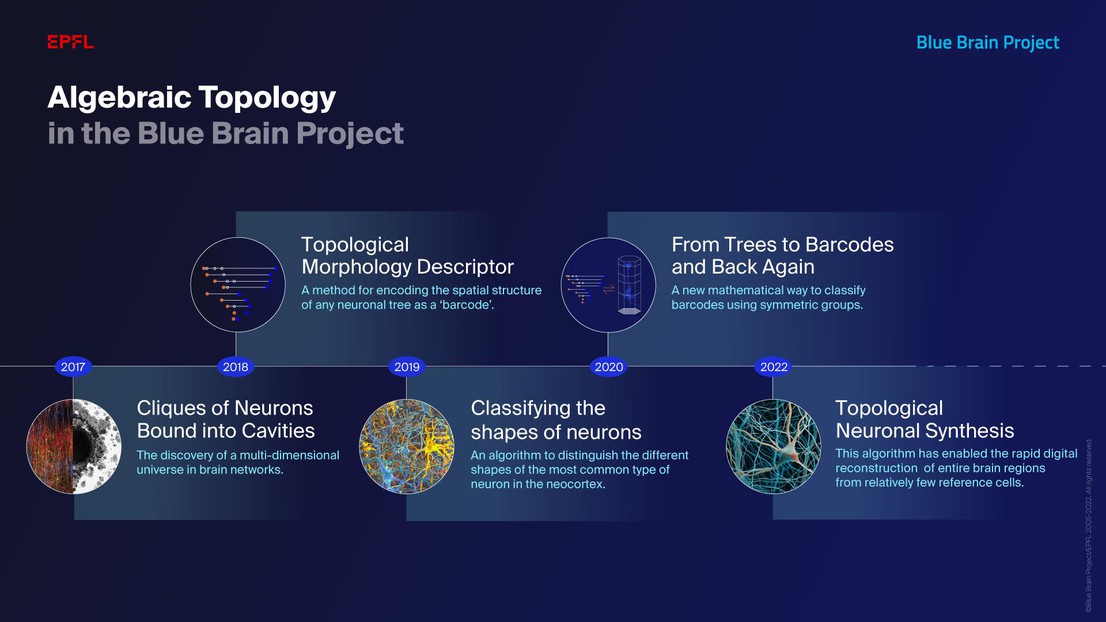
© 2022 EPFL
In the Spring of 2022, the EPFL Blue Brain Project announced in a paper published in Cell Reports that it had found a way to mathematically build the 3D tree-like geometries of neurons using algebraic topology. One of the main branches of pure mathematics, algebraic topology allowed Blue Brain to describe the geometrical shapes of neurons in a way that could be used to build their digital twins. This breakthrough opens the path to using computers to automatically build digital copies of any of the thousands of different types of neurons found in the brain. The study, led by Blue Brain’s Neuromathematics Leader Lida Kanari and EPFL Professor Kathryn Hess of the Laboratory for Topology and Neuroscience, was the latest in a series of Blue Brain studies where algebraic topology helped tackle and solve a wide range of previously intractable neuroscience problems.
Founded in 2005 by Henry Markram, Blue Brain has made significant progress in pursuit of its goal - to build biologically detailed digital twins of brain tissue, and ultimately the whole mouse brain. Once achieved for rodents, the approach can be gradually adapted and applied to other species, including, eventually, the Human brain.
The Blue Brain approach uses machine reading to draw from information published in hundreds of thousands of scientific papers, and data mining to extract values deposited in brain databases all around the world. It infers some of the missing data and gaps in our knowledge using knowledge graphs, and then develops algorithms that can create digital copies of neurons and brain tissue where the neurons are connected via synapses. A digital twin is only an approximation of real brain tissue, but its accuracy increases as more data and knowledge are incorporated and the inference algorithms improve. Simulating digital twins of rat and mouse brain tissue allows previously unimaginable experiments simply because every parameter of the brain tissue can be accessed. “If we can add simulation neuroscience as a third branch to the existing experimental and theoretical branches of neuroscience, we stand a chance of understanding the brain”, says Henry Markram.
Building a digital copy of brain tissue is a complex problem. New methods and ideas need to be invented and explored along the way. The solution to a specific problem at various points on this journey can come from virtually any discipline; from computer science, biology, chemistry, physics, or mathematics. In fact, Blue Brain found some powerful tools when it ventured far afield from neuroscience and brought in a branch of pure mathematics – algebraic topology.
Discussions on how algebraic topology could be relevant to neuroscience began between the topologist Ran Levi from Aberdeen University and the neuroscientist Henry Markram in the ‘90s. However, it was only when the first digital twins of brain tissue were developed by the Blue Brain, some 30 years later, that any of these ideas could be tested. Kathryn Hess, a topologist from the EPFL, joined the collaboration in 2005, and in 2015 established the neuroscience topology group to tackle some of the hardest problems that Blue Brain was facing.
In a nutshell, algebraic topology is a mathematical approach to study the properties of multi-dimensional geometric shapes. A tree has a structure and that structure has complex properties; a social network of people has a structure with complex properties, as does the internet. Similarly, a neuron has a structure with complex properties and so does a network of neurons. Algebraic topology has the advantage of not being limited by dimensions, such as the size of the elements and timeframes within which something must happen; it looks at the way the elements are connected. In the context of its use in neuroscience, “Algebraic topology is like a telescope and microscope at the same time,” explains Kathryn Hess. “It can zoom into networks, the trees in the forest, and look at the spaces between the trees at the same time. Algebraic topology is particularly well suited to describing and characterizing the emergence of global structures from local constraints”.
Discovering a multi-dimensional universe in brain networks
In 2017, the Blue Brain Project published a ground-breaking paper – Cliques of Neurons Bound into Cavities Provide a Missing Link between Structure and Function – that has been viewed over 350,000 times, triggered a flurry of topological studies into brain networks, and even ushered in a new topic in mathematics conferences.
Using algebraic topology to study the way neurons group together to form geometrical shapes, revealed a universe of complex multi-dimensional geometric structures never seen before. The building blocks of these geometric structures arise when a group of neurons become connected to each other in a specific way, called a directed clique. A directed clique forms when one neuron connects to all others in a group of neurons, the second neuron in the clique connects to all others except the first one, the third to all others except the first and second one, and so on, until all neurons in the clique are connected. In directed cliques, the information flows preferably into the group through one member (the source neuron) and out of the group through another (the sink neuron). In the language of algebraic topology, the more neurons there are in a clique, the higher the dimension of the topological structure. Groups with many neurons forming a directed clique - high dimensional directed cliques – have such an unusual structure that they are rarely found in completely random networks.
Two years earlier, Blue Brain had published the first digital copy of a piece of the neocortex — the most evolved part of the brain and the seat of our sensations, actions, and consciousness. Using this digital brain tissue, topological analyses revealed a universe of multi-dimensional directed cliques. This discovery could only have been made on digital brain tissue because it is impossible to map out how every neuron is connected to every other neuron in real brain tissue. An experiment on real brain tissue designed by Michael Reimann, who heads the simulation team in Blue Brain, with Rodrigo Perin, who leads Markram’s experimental laboratory, later indirectly confirmed that multi-dimensional topological structures do indeed exist in real brain tissue and that they cannot be there by chance; they have to be constructed specifically by some as yet unknown mechanism in the brain.
Guided by Kathryn Hess, Ran Levi, and their groups, the team asked how these directed cliques shape the activity that emerges in the brain. They found that how neurons are connected matters. Activity in groups of neurons connected in directed cliques was far more coordinated than if they were randomly connected, and the bigger the directed clique, the more coordinated the activity was.
This remarkable study did not stop there. They addressed the next conundrum: How can a brain make sense of the world when its neurons always react differently to the same stimulus? Having used the tools of algebraic topology to look at the fine structure – the trees – of the connectivity and activity, a new tool from the topology toolbox was used to simultaneously look at the global structure – the spaces between the trees. In other words, the cavities in the network of neurons that the connected neurons were leaving behind were examined.
Since the connectivity between neurons is not only structural, but can switch on and off as the brain reacts to a stimulus, the team tracked the evolution of the cavities while the digital tissue responded to a stimulus. They found something remarkable: while the digital brain tissue responded differently to the same stimulus, as was expected, the sequence of numbers of cavities that were created during the stimulus was the same. The activity was spreading from lower dimensional directed cliques creating small cavities, to higher dimensional cliques creating bigger cavities, somewhat like seeing bubbles in foam grow. The cavities reached a peak dimensionality before collapsing. It was the equivalent of watching structures repeatedly rise and fall. Could such structures be the basis of perception? The answer to this question will need to wait for larger, and perhaps even more accurate digital brain models.
Kathryn Hess, commented at the time, “When you look at the patterns in which neurons are firing through the filter of algebraic topology, different structures emerge. Moreover, when you look at the evolution of such a pattern through time, it gives us a mathematical signature that describes this pattern that was hidden in what seemed like random firing of the network.”
“Algebraic topology promises to become one of the most important keys to guide our attempts at understanding the complexity of the brain. For example, one of the hardest problems in neuroscience is to explain how wiring up all the brain’s neurons a particular way – the so-called connectome – dictates our response to the world, our cognitive capabilities, and our individuality. Answering how different connectomes shape brain activity can help explain what makes us human, and what makes each animal species so different” explains Prof. Henry Markram, “We have suspected for a long time that the reason we struggle to truly understand the brain is because it is so difficult to imagine beyond our 3- and 4-dimensional world. We will need algebraic topology to help us map multi-dimensional patterns of electrical activity that are created as we experience the world and help us begin to understand how the structure of the brain dictates its function”.
Since these discoveries, the Blue Brain has been testing where else algebraic topology can help solve the hard problems.
A barcode for neurons solves a century-old problem
A tree-like branching pattern, such as that of neurons, is a common morphology in biological systems. To tell different kinds of morphologies apart, it is mostly enough to just look and compare their branching patterns. However, if one wants to draw a classification of neurons, the differences between their morphologies must be quantified. Two problems have stopped neuroscience from doing this until now. Firstly, while neurons have a wide range of branching patterns, it is often much harder to tell one type of neuron apart from another, leading to debates between neuroscientists that can last for decades, blocking progress. Secondly, a variety of mathematical methods have been tried to measure the differences in the branching pattern of neurons, but none were good enough to get all neuroscientists to agree.
Blue Brain, led by Lida Kanari and Kathryn Hess, solved this century-old problem. Using yet another technique from the algebraic topology toolbox, called persistent homology, they could develop a barcode for neurons that reliably tells them apart. The key to the success of the method is to abstract the branching patterns in a way that allows simultaneous measurement of the global and local patterns. It’s the equivalent of asking, “what kind of branching pattern could explain the overall shape?” This method of fingerprinting the shape of neurons, called a Topological Morphology Descriptor (TMD), was published in the journal Neuroinformatics in early 2018.
In 2019, in a front-cover paper published in Cerebral Cortex, the team showed they could use the TMD method to solve the persistent problem of objectively classifying neurons. “We developed an algorithm to distinguish the different shapes of the most common type of neuron in the neocortex – the pyramidal cells”, explains Lida Kanari. “Our study proved for the first time that objective classification of these pyramidal cells is possible by applying tools from algebraic topology.”
This method has the potential to accelerate progress in neuroscience and benefit the whole community by allowing neuroscientists to start counting and classifying the different types of neurons in the brain, in different brain regions, and in different animal species.Neuroscientists can now follow neurons as they change during development and aging and eventually spot diseases in the clusters of neurons.
Scientists at Blue Brain can now correlate the different electrical behaviors of neurons to their morphologies and create algorithms that build neurons with a particular shape and electrical behavior. They can begin to understand how the shape of neurons depends on gene regulation allowing them to eventually build the brain by looking at which genes are activated. Naturally, a lot more work is needed to answer all these questions and enable all these approaches, but there now is a solid method to characterize and quantify different types of neurons.
Enabling the rapid digital reconstruction of entire brain regions from relatively few reference cells
What can be measured can be built; the team obtained the barcodes of neurons and developed an algorithm that can be guided by these topological descriptors to artificially build digital copies of neurons. This new algorithm, called the Topological Neuronal Synthesis (TNS), was published in Cell Reports. The TNS now allows Blue Brain to build digital copies of all the neurons found in entire brain regions from just a few barcodes. “Eventually we want to be able to feed the algorithm pictures of neurons in papers published by all neuroscientists since the famous Ramón y Cajal started photographing and drawing neurons with pen and paper over a century ago, which are lying “inanimate” in the literature, and turn them into digital copies and make them “come alive” by recreating their electrical activity. Imagine the value of actually being able to use a century of neuroscience data,” says Henry Markram.
Lida Kanari explains further, “The findings are already enabling us to build biologically detailed reconstructions and simulations of the mouse brain, by computationally reconstructing brain regions for simulations which replicate the anatomical properties of neuronal morphologies and include region-specific anatomy”. Blue Brain also addresses another major challenge in neuroscience: it is difficult and takes days behind a microscope to draw a single stained neuron in 3D. This has severely limited the number of 3D digital neurons that are available for neuroscientists to study. “Using the TNS algorithm, we can synthesize millions of unique neuronal morphologies, up to 10 million cells in a few hours,” she says.
From Trees to Barcodes and Back Again
Further work using the TMD can be seen in the 2020 publication, From Trees to Barcodes and Back Again: Theoretical and Statistical Perspectives.
The team developed a new mathematical way to classify barcodes using symmetric groups, enabling them to provide theoretical and computational insights into the relation between the TMD and TNS. The extent to which the TNS recovers a geometric tree from its TMD was investigated and the effect of different types of noise on the process of tree generation from persistence diagrams described. The investigations oncluded that the TNS algorithm is stable with respect to specific types of noise providing a further guarantee of the reliability of the algorithm.
The team has clear plans for their next steps. “We intend to continue exploring the distinguishing characteristics of the tree-like shapes of neurons,” confirms Lida Kanari. “With the goal of explaining the structural and functional implications for the observed geometric and combinatorial constraints. On the mathematical side, we are currently analyzing the structure on the space of barcodes revealed by the symmetric groups and determining what information can be extracted from the induced stratification of this space. This structure on the space of barcodes should also provide significant insights into the still somewhat mysterious space of geometric trees, which is of considerable interest to a wide range of mathematicians,” Lida concludes.
“Neurotopology is accelerating Blue Brain and can accelerate many areas of neuroscience” concludes Prof. Henry Markram.
Links
- Cliques of Neurons Bound into Cavities Provide a Missing Link between Structure and Function (2017)
- Reconstruction and Simulation of Neocortical Microcircuitry (2015)
- A Topological Representation of Branching Neuronal Morphologies (2017)
- Objective Morphological Classification of Neocortical Pyramidal Cells (2019)
- Computational synthesis of cortical dendritic morphologies (2022)
- From Trees to Barcodes and Back Again: Theoretical and Statistical Perspectives (2020)