PhD Defense of Ya-Ping Hsieh
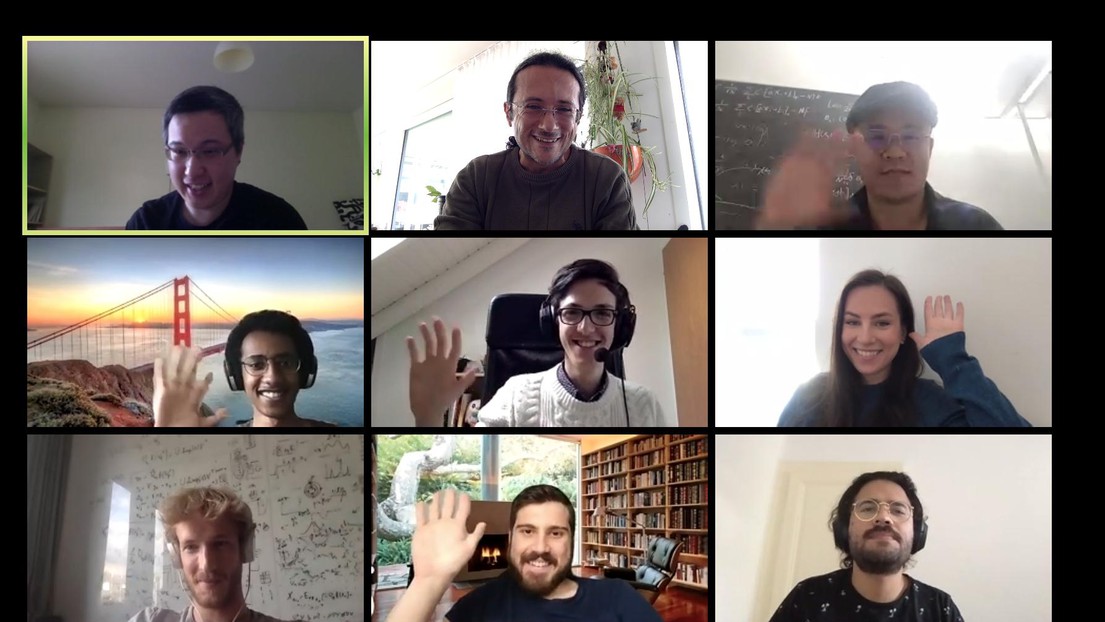
Zoom defence of Ya-Ping Hsieh © Volkan Cevher / 2020 EPFL
On 30th September 2020, Ya-Ping Hsieh, a PhD student at LIONS lab, successfully defended his PhD thesis. The thesis, entitled "Convergence without convexity: sampling, optimization, and games" was supervised by Prof. Volkan Cevher.
Due to Covid-19 the presentation was held remotely via Zoom.
Ya-Ping's thesis is about convergence theory without assuming convexity. We solved some important non-convex sampling problems, and rigorously characterized why non-convex/non-concave games are hard to train. To remedy the situation, we propose to consider the mixed Nash equilibrium, and by doing so achieve the state-of-the-art on training robust reinforcement learning agents.
Abstract:
Many important problems in contemporary machine learning involve solving highly non- convex problems in sampling, optimization, or games. The absence of convexity poses significant challenges to convergence analysis of most training algorithms, and in some cases (such as min-max games) it is not even known whether common training algorithms converge or not. In this thesis, we aim to partially bridge the gap by 1. Proposing a new sampling framework to transform non-convex problems in to convex ones. 2. Characterizing the convergent sets of a wide family of popular algorithms for min-max optimization. 3. Devising provably convergent algorithms for finding mixed Nash Equilibria of infinite-dimensional bi-affine games. Our theory has several important implications. First, we resolve a decade-old open problem in Bayesian learning via our non-convex sampling framework. Second, our algorithms for bi-affine games apply to the formidably difficult training of generative adversarial networks and robust reinforcement learning, and on both examples we demonstrate promising empirical performance. Finally, our results on min-max optimization lead to a series of negative results for state-of-the-art algorithms, suggesting that one requires fundamentally new tools to advance the theory.