Performance is Relative: Implications for Robust Decision-Making
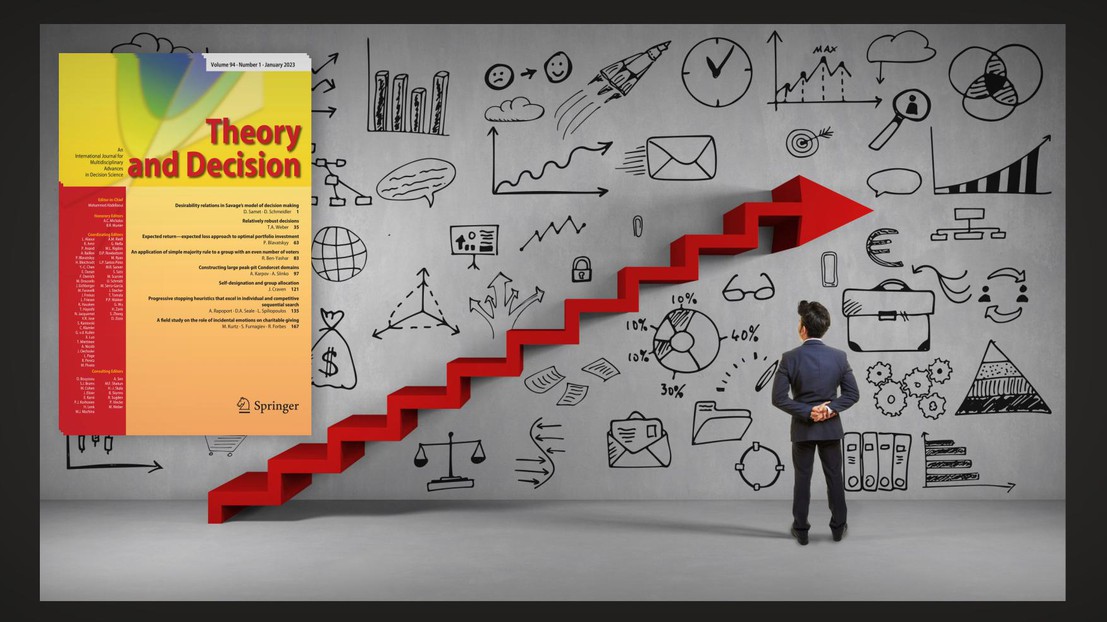
© 2023 EPFL
A new research paper by Prof. Weber on “Relatively Robust Decisions” examines decisions which attain the best relative performance across many random scenarios. The results, published in the January 2023 issue of Theory and Decision, a leading journal in the field of Decision Science, provide structural insights on this important decision-making problem.
When trying to find the best price for a new innovative product, it is difficult to put probabilities on possible demand scenarios. The reason for this is that the product has never existed before, and one may specify perhaps only an upper and lower bound for demand, but not how likely (conditional on a price) each potential demand volume might be. In a standard “absolute” robustness framework, the company should charge a price that maximizes the worst-case scenario, which in this example would simply mean to charge the price which is best for the lowest conceivable demand for the product. This amounts to a very bleak prospect indeed, and most likely would turn out to be very costly for the firm, since the price would probably be much too low for the actual observed demand. By contrast, the “relative performance” of a given pricing decision d, across a range of possible scenarios x, can be measured as follows. For any scenario x find the ratio of the payoff under the decision d and the best-possible payoff when taking a decision d*(x) that is perfectly adapted to the scenario x. For positive payoffs, this ratio is always a number between 0 and 1. Thus, the relative performance of the decision d must then be the worst of these ratios—with respect to all demand scenarios x. As a result, determining a “relatively robust decision” that maximizes relative performance, so as to attain, say, a value of 0.7, provides a guarantee to always reach at least 70 percent of the payoff that could have been achieved with perfect foresight. Professor Weber’s article formalizes this framework and links it to the theory of “monotone comparative statics” in Economics. This allows for simple closed-form solutions in practical applications, such as the choice of a service for which a decision does not yet know her utility, such as for an electric vehicle before having ever owned one, discussed in the paper.
The research in [1] is part of an ongoing project on relative robustness at the Chair of Operations, Economics and Strategy (OES) at EPFL. It also builds on the earlier work [2] on fair resource allocation by the author together with some of his colleagues at Stanford University, published in 2009.
Abstract
It is natural for humans to judge the outcome of a decision under uncertainty as a percentage of an ex-post optimal performance. We propose a robust decision-making framework based on a relative performance index. It is shown that if the decision maker’s preferences satisfy quasisupermodularity, single-crossing, and a nondecreasing log differences property, the worst-case relative performance index can be represented as the lower envelope of two extremal performance ratios. The latter is used to characterize the agent’s optimal robust decision, which has implications both computationally and for obtaining closed-form solutions. We illustrate our results in an application which compares the performance of relative robustness to solutions that optimize worst-case payoffs, maximum absolute regret, and expected payoffs under a Laplacian prior.
[1] Weber, T.A. (2023) “Relatively Robust Decisions,” Theory and Decision, Vol. 94, No. 1, pp. 35—62. [DOI: https://doi.org/10.1007/s11238-022-09866-z; open access]
[2] Goel, A., Meyerson, A., Weber, T.A. (2009) “Fair Welfare Maximization,” Economic Theory, Vol. 41, No. 3, pp. 465—494. [DOI: 10.1007/s00199-008-0406-0]