Our paper on self supervised learning (SSL) accepted to CVPR 2022
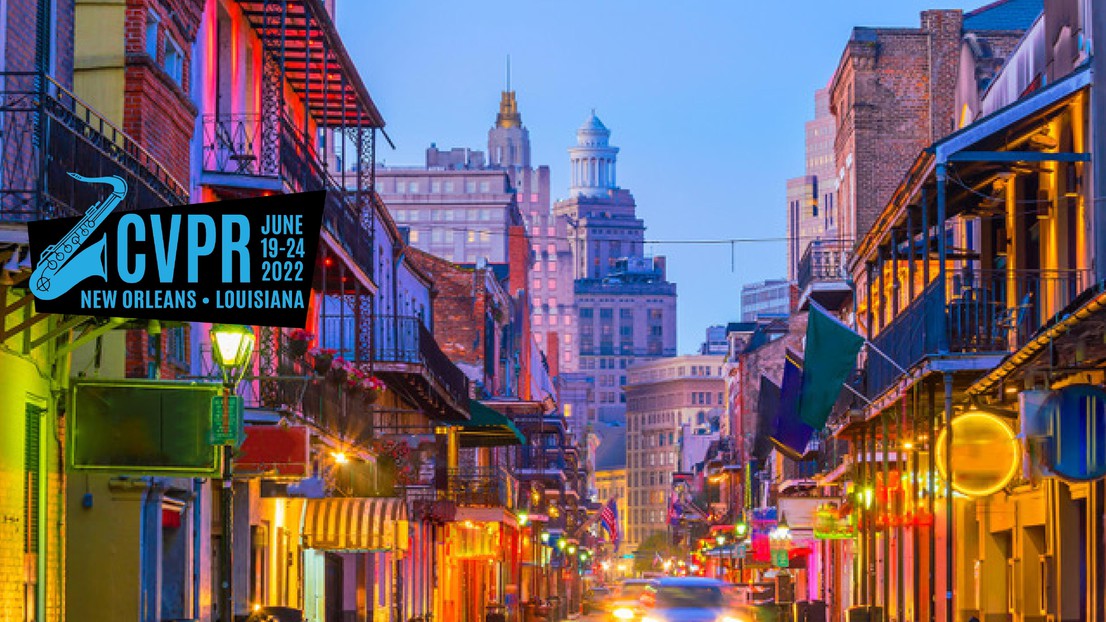
© 2022 CVPR
The paper "Leverage Your Local and Global Representations: A New Self-Supervised Learning Strategy" by Tong Zhang, Congpei Qiu, Wei Ke, Sabine Süsstrunk, Mathieu Salzmann is accepted to CVPR2022.
IVRL member Tong Zhang will present his work at the Computer Vision and Pattern Recognition conference taking place in New Orleans, Louisiana from June 19 to June 24, 2022.
Self-supervised learning (SSL) methods aim to learn view-invariant representations by maximizing the similarity between the features extracted from different crops of the same image regardless of cropping size and content. In essence, this strategy ignores the fact that two crops may truly contain different image information, e.g., background and small objects, and thus tends to restrain the diversity of the learned representations. In this work, we address this issue by introducing a new self-supervised learning strategy, LoGo, that explicitly reasons about Local and Global crops. To achieve view invariance, LoGo encourages similarity between global crops from the same image, as well as between a global and a local crop. However, to correctly encode the fact that the content of smaller crops may differ entirely, LoGo promotes two local crops to have dissimilar representations, while being close to global crops. Our LoGo strategy can easily be applied to existing SSL methods.