New publication in the Electric Power Systems Research journal
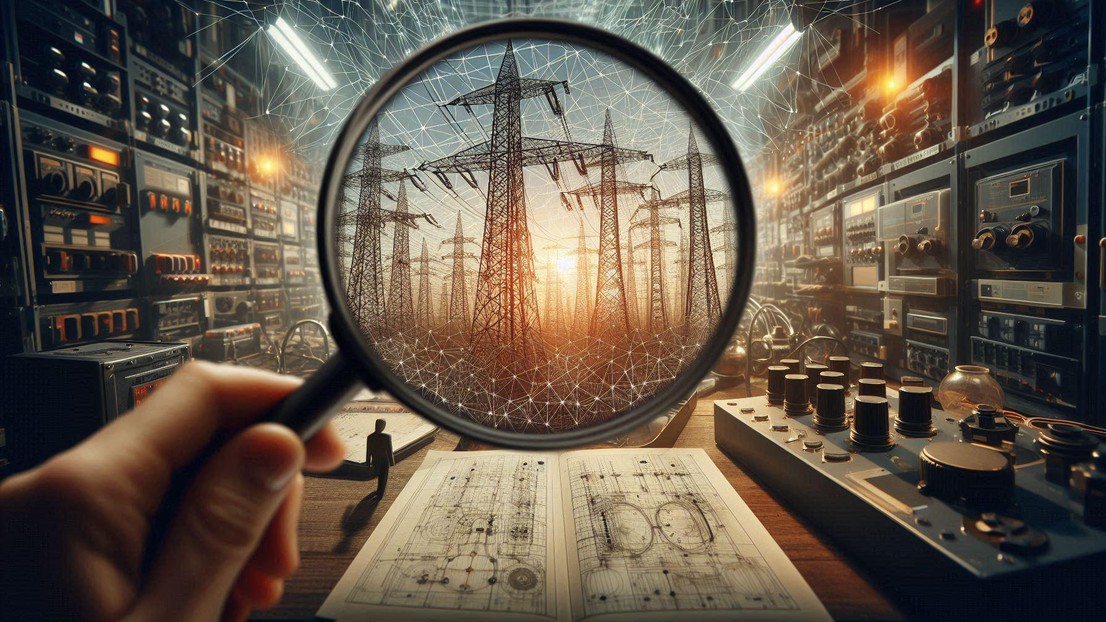
© 2024 EPFL
Modeling existing distribution grids is essential for implementing the advanced control techniques required for the energy transition. However, using machine learning for this purpose faces significant challenges due to the limitations of current sensing technology. The paper "Power Grid Parameter Estimation Without Phase Measurements: Theory and Empirical Validation" offers a statistical analysis of the errors in various state-of-the-art estimation methods. The key finding is that, until advanced sensors become more affordable, control engineers should prioritize using the impedance matrix over the admittance matrix wherever possible.
Reliable integration and operation of renewable distributed energy resources requires accurate distribution grid models. However, obtaining precise models is often prohibitively expensive, given their large scale and the ongoing nature of grid operations. To address this challenge, considerable efforts have been devoted to harnessing abundant consumption data for automatic model inference. The primary result of the paper is that, while the impedance of a line or a network can be estimated without synchronized phase angle measurements in a consistent way, the admittance cannot. Furthermore, a detailed statistical analysis is presented, quantifying the expected estimation errors of four prevalent admittance estimation methods. Such errors constitute fundamental model inference limitations that cannot be resolved with more data. These findings are empirically validated using synthetic data and real measurements from the town of Walenstadt, Switzerland, confirming the theory. The results contribute to our understanding of grid estimation limitations and uncertainties, offering guidance for both practitioners and researchers in the pursuit of more reliable and cost-effective solutions.