New PhD Dan Assouline: AI sees potential for renewables
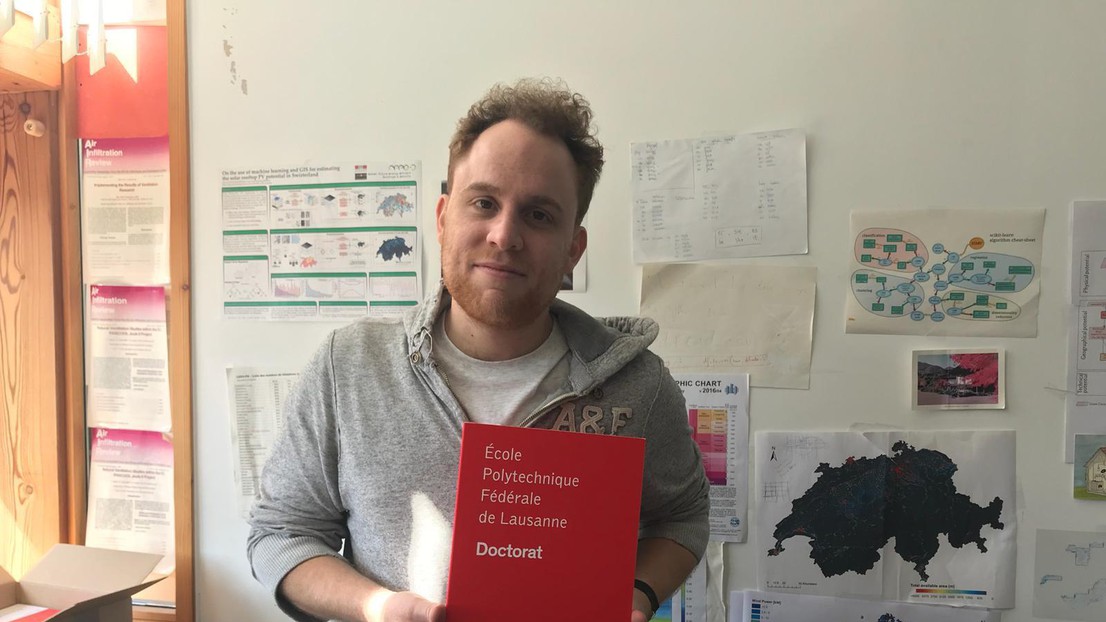
Dan Assouline © 2019 EPFL LESO-PB
Dan Assouline has obtained a PhD for his thesis "Machine Learning and Geographic Information Systems for large-scale mapping of renewable energy potential" written at the EPFL Solar Energy and Building Physics Lab under the supervision of Dr Nahid Mohajeri and Prof. Jean-Louis Scartezzini. Based on machine learning methods and GIS, his mapping of the Swiss renewable energy potential for different technologies yields valuable information for the definition of regional energy strategies as well as methods that are applicable in different regions of the World. Furthermore, his studies suggest that it is effectively possible to cover the energy needs of Switzerland solely with renewables.
Optimizing the use of renewable energy requires the assessment of their potential supply, along with the demand loads in locations of interest. In particular, large-scale supply estimation studies are needed in order to evaluate areas of high potential for each type of energy source for a particular region, and allow the elaboration of efficient global energy strategies. In Switzerland, the “Energy Strategy 2050”, initiated in 2011 by the Swiss Federal Council, sets an example with the ambitious goal of reaching a 50-80% reduction of CO2 emissions by the year 2050, with a clear course of action: phasing-out nuclear power, improving energy efficiency and greatly increasing the use of renewables.
This thesis develops a general data-driven strategy combining Geographic Information Systems and Machine Learning methods to map the large-scale energy potential for three very popular sources of decentralized energy systems: wind energy (using horizontal axis wind turbines), geothermal energy (using very shallow ground source heat pumps) and solar energy (using photovoltaic solar panels over rooftops). For each of the three considered energy sources, an adapted methodology is suggested to assess its large-scale potential, by estimating multiple variables of interest with a suitable time resolution, using widely available data, and combining these variables into potential values. The use of Machine Learning algorithms (notably Support Vector Machines and Random Forests) allows the prediction of the variables at unknown locations, along with the uncertainty attached to the predictions. In each case, the developed methodology is set up with an aim to be applied for Switzerland, meaning that it relies on Swiss available energy-related data. Such data, however, including meteorological, topographic, ground/soil-related and building-related data, is becoming progressively available for most countries, making it possible to widely generalize the proposed methodologies.
Results show that Machine Learning is adequate for energy potential estimation, as the multiple required predictions and spatial extrapolations are achieved with reasonable accuracy. Final values validated with other existing data or studies show general agreement. For Switzerland, the results show a significant potential for the considered renewables. In particular, there is a relatively high potential for Rooftop- Mounted solar PV panels, as it is estimated that they could generate a total electricity production of 16.3 TWh per year, which corresponds to 25.3% of the annual electricity demand in 2017.
This research was funded by the Swiss Innovation Agency Innosuisse through the Swiss Competence Center for Energy Research "Future Energy Efficient Buildings and Districts" SCCER FEEB&D.
Assouline, Dan; Mohajeri, Nahid; Scartezzini, Jean-Louis (dirs.), Machine Learning and Geographic Information Systems for large-scale mapping of renewable energy potential, EPFL Thesis no 9376, 2019