New networked operator parametrization allows unconstrained learning!
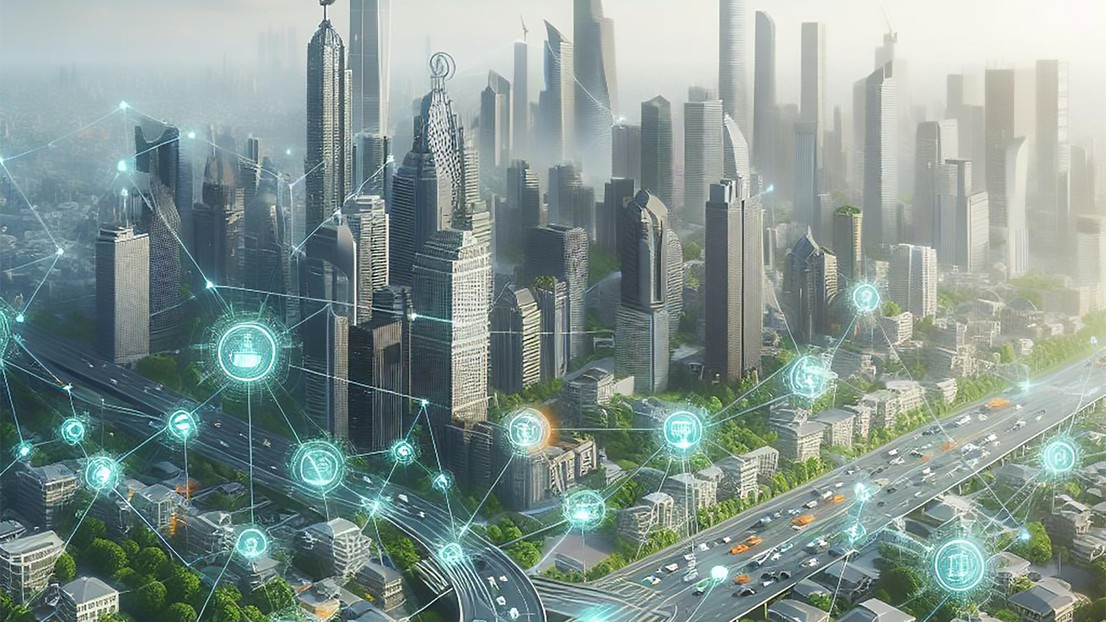
© 2024 EPFL
Our paper "Unconstrained learning of networked nonlinear systems via free parametrization of stable interconnected operators" got accepted for the European Control Conference 2024 (ECC24)!
This paper introduces a novel parametrization technique for nonlinear networked incrementally L2-bounded operators in discrete time. The key innovation is a "free" parametrization that yields a sparse large-scale operator with bounded incremental L2 gain regardless of parameter values. This enables efficient parameter optimization using unconstrained gradient descent, making it applicable in large-scale optimal control and system identification. Moreover, it allows embedding prior knowledge about system interconnection topology and stability directly into the designed distributed operator. The method can incorporate state-of-the-art Neural Network (NN) parametrizations seamlessly and is demonstrated through a simulation example, highlighting its superiority over standard NN-based identification methods without enforced priors on system topology and stability.
NCCR Automation