New benchmark helps solve the hardest quantum problems
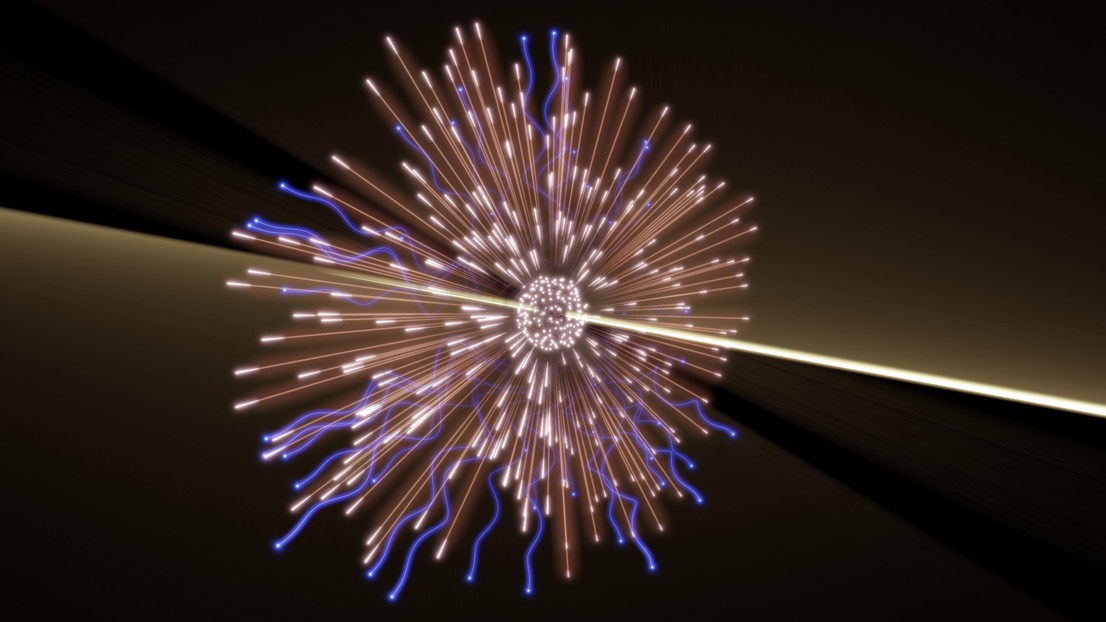
©EPFL/iStock photos (Peter Hansen)
Predicting the behavior of many interacting quantum particles is a complicated process but is key to harness quantum computing for real-world applications. A collaboration of researchers led by EPFL has developed a method for comparing quantum algorithms and identifying which quantum problems are the hardest to solve.
From subatomic particles to complex molecules, quantum systems hold the key to understanding how the universe works. But there's a catch: when you try to model these systems, that complexity quickly spirals out of control — just imagine trying to predict the behavior of a massive crowd of people where everyone is constantly influencing everyone else. Turn those people into quantum particles, and you are now facing a “quantum many-body problem”.
Quantum many-body problems are efforts to predict the behavior of a large number of interacting quantum particles. Solving them can unlock huge advances in fields like chemistry and materials science, and even push the development of new tech like quantum computers.
But the more particles you throw into the mix, the harder it gets to model their behavior, especially when you're looking for the ground state, or lowest energy state, of the system. This matters because the ground state tells scientists which materials will be stable and could even reveal exotic phases like superconductivity.
For every problem, a solution: but which one?
For years, scientists have relied on a mix of methods like quantum Monte Carlo simulations and tensor networks (variational wave functions) to approximate solutions to these problems. Each method has its strengths and weaknesses, but it’s hard to know which one works best for which problem. And until now, there hasn’t been a universal way to compare their accuracy.
A large collaboration of scientists, led by Giuseppe Carleo at EPFL has now developed a new benchmark called the “V-score” to tackle this issue. The V-score (“V” for “Variational Accuracy”) offers a consistent way to compare how well different quantum methods perform on the same problem. The V-score can be used to identify the hardest-to-solve quantum systems, where current computational methods struggle, and where future methods —such as quantum computing — might offer an advantage.
The breakthrough method is published in Science.
How the V-score works
The V-score is calculated using two key pieces of information: the energy of a quantum system and how much that energy fluctuates. Ideally, the lower the energy and the smaller the fluctuations, the more accurate the solution. The V-score combines these two factors into a single number, making it easier to rank different methods based on how close they come to the exact solution.
To create the V-score, the team compiled the most extensive dataset of quantum many-body problems to date. They ran simulations on a range of quantum systems, from simple chains of particles to complex, frustrated systems, which are notorious for their difficulty. The benchmark not only showed which methods worked best for specific problems, but also highlighted areas where quantum computing might make the biggest impact.
Solving the hardest quantum problems
Testing the V-score, the scientists found that some quantum systems are much easier to solve than others. For example, one-dimensional systems, such as chains of particles, can be tackled relatively easily using existing methods like tensor networks. But more complex, high-dimensional systems like frustrated quantum lattices, have significantly higher V-scores, suggesting that these problems are much harder to solve with today’s classical computing methods.
The researchers also found that methods relying on neural networks and quantum circuits — two promising techniques for the future — performed quite well even when compared to established techniques. What this means is that, as quantum computing technology improves, we may be able to solve some of the hardest quantum problems out there.
The V-score gives researchers a powerful tool to measure progress in solving quantum problems, especially as quantum computing continues to develop. By pinpointing the hardest problems and the limitations of classical methods, the V-score could help direct future research efforts. For instance, industries that rely on quantum simulations, such as pharmaceuticals or energy, could use these insights to focus on problems where quantum computing could give them a competitive edge.
List of contributors
- EPFL Computational Quantum Science Lab
- Sorbonne Université
- University of Zurich
- Università di Trieste
- Flatiron Institute
- Vector Institute
- Goethe-Universität
- Collège de France
- CNRS École Polytechnique
- Université de Genève
- University of Waterloo
- Toyota Physical and Chemical Research Institute
- Waseda University
- Sophia University
- Paul Scherrer Institute (PSI)
- University of Zurich
- IBM Quantum
- Columbia University
- New York University
- Keio University
- Université Paris-Saclay
- University of Tokyo
- University of California Irvine
- International School for Advanced Studies (SISSA)
- Politecnico di Torino
- Chinese Academy of Sciences
- Max Planck Institute
- University of Chinese Academy of Sciences
- College of William and Mary
Swiss National Science Foundation (SNSF)
Simons Foundation
Deutsche Forschungsgemeinschaft (DFG)
European Union's Horizon 2020 research and innovation program
Japanese Ministry of Education, Culture, Sports, Science and Technology (MEXT)
RIKEN Center for Computational Science
Natural Sciences and Engineering Research Council (NSERC)
Shared Hierarchical Academic Research Computing Network (SHARCNET)
Compute Canada
Canadian Institute for Advanced Research (CIFAR)
Dian Wu, Riccardo Rossi, Filippo Vicentini, Nikita Astrakhantsev, Federico Becca, Xiaodong Cao, Juan Carrasquilla, Francesco Ferrari, Antoine Georges, Mohamed Hibat-Allah, Masatoshi Imada, Andreas M. Läuchli, Guglielmo Mazzola, Antonio Mezzacapo, Andrew Millis, Javier Robledo Moreno, Titus Neupert, Yusuke Nomura, Jannes Nys, Olivier Parcollet, Rico Pohle, Imelda Romero, Michael Schmid, J. Maxwell Silvester, Sandro Sorella, Luca F. Tocchio, Lei Wang, Steven R. White, Alexander Wietek, Qi Yang, Yiqi Yang, Shiwei Zhang, and Giuseppe Carleo. Variational Benchmarks for Quantum Many-Body Problems. Science 17 October 2024. DOI: 10.1126/science.adg9774