Nanopores and deep learning change disease diagnostics
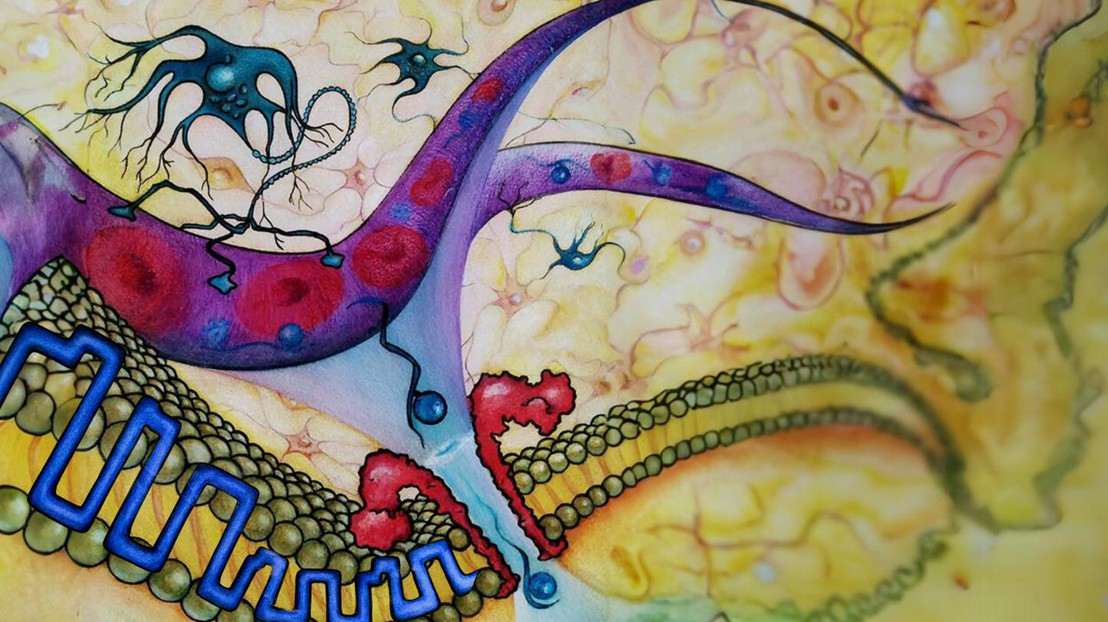
Deep learning assisted single-molecule detection of protein post-translational modifications with a biological nanopore. Credit: Andrea Vucicevic/EPFL
EPFL scientists unveil a groundbreaking method using biological nanopores and deep learning to detect protein modifications, offering new avenues in disease diagnostics.
Proteins, the workhorses of the cell, undergo various modifications after their synthesis. Because they can profoundly affect how a protein operates in the cell, these “post-translational modifications”, or PTMs, are key in numerous biological processes.
PTMs also serve as biomarkers for several diseases, which means that it is crucial that we can detect and analyze them accurately to avoid mistaken diagnoses. But traditional methods, however, are limited in sensitivity and specificity, especially when dealing with low concentrations of proteins and complex PTM patterns.
Now, scientists at EPFL have developed a novel method that combines the sensitivity of biological nanopores with the precision of deep learning. The innovative approach can transform how we detect and analyze PTMs.
The study was led by the bioengineering groups of Matteo Dal Peraro, Chan Cao and Hilal Lashuel at EPFL’s School of Life Sciences and is published in ACS Nano.
The new method centers on the use of a biological nanopore, specifically the pore-forming toxin aerolysin, to detect and distinguish peptides, the building blocks of proteins, with different PTMs. Dal Peraro’s group has previously worked with aerolysin-based nanopores to make high-res sensors of complex molecules and even read data encoded into synthetic macromolecules. This nanopore technology is sensitive enough to detect these peptides at picomolar concentrations, a significant improvement over existing techniques.
But how does the method work? As the peptides pass through the nanopore, they cause characteristic changes in the flow of ions across the nanopore – the so-called “ionic current”. Each type of PTM changes the peptide's structure in a unique way, leading to distinct signatures of currents; by recording these changes in current, the method can identify and differentiate between various PTMs on the peptides.
What makes this approach stand out even more is that it then uses deep-learning algorithms to analyze the complex data and precisely classify the peptides based on their PTM patterns. The model can confidently identify the characteristic current signatures of peptides and their PTM variants, providing a fast, automatic, and highly accurate way to classify them.
To test the approach, the researchers turned to the expertise of Lashuel, whose lab has pioneered the development of synthetic and chemical biology approaches to investigate the role of PTMs neurodegenerative diseases. “We demonstrated that we can exploit the sensing power of our nanopore to detect and discriminate various PTM forms of alpha-synuclein, one of the most sought-after biomarkers and targets for developing therapies to treat Parkinson’s,” says Chan Cao, the study’s leading author.
The scientists successfully showed that the nanopore method could detect and differentiate alpha-synuclein proteins with single or multiple PTMs, such as phosphorylation, nitration, and oxidation. “This ability to identify multiple modifications simultaneously is a game-changer,” says Lashuel. “It allows for more precise mapping of the PTM code of proteins at the single molecule levels and thus could help uncover new insights into the complex interplay and dynamics of PTMs in disease processes and their potential as disease biomarkers.”
This combination of nanopore sensing and advanced data analysis opens up new possibilities for understanding protein modifications at a level of detail previously unattainable. Nanopore technology can not only be used for PTM detection but also in biomarker discovery and diagnostics.
“We gave a first proof-of-principle that this approach can be used to detect these biomarkers in a mimic of a clinical sample, providing ground for developing single-molecule diagnostic tools for Parkinson’s disease,” says Dal Peraro. The team envisions that the method could be developed into a portable diagnostic device, offering a fast, cost-effective, and highly sensitive tool for medical and commercial use.
Other contributors
- University of Geneva
Swiss National Science Foundation
Horizon 2020
Peter and Traudl Engelhorn Foundation
Synapsis foundation
Chan Cao, Pedro Magalhães, Lucien Fabrice Krapp, Juan F. Bada Juarez, Simon Mayer, Verena Rukes, Anass Chiki, Hilal A. Lashuel, Matteo Dal Peraro. Deep learning-assisted single-molecule detection of protein post-translational modifications with a biological nanopore. ACS Nano 19 December 2023. DOI: 10.1021/acsnano.3c08623