Modeling Avalanches: “We're still in the kitchen.”
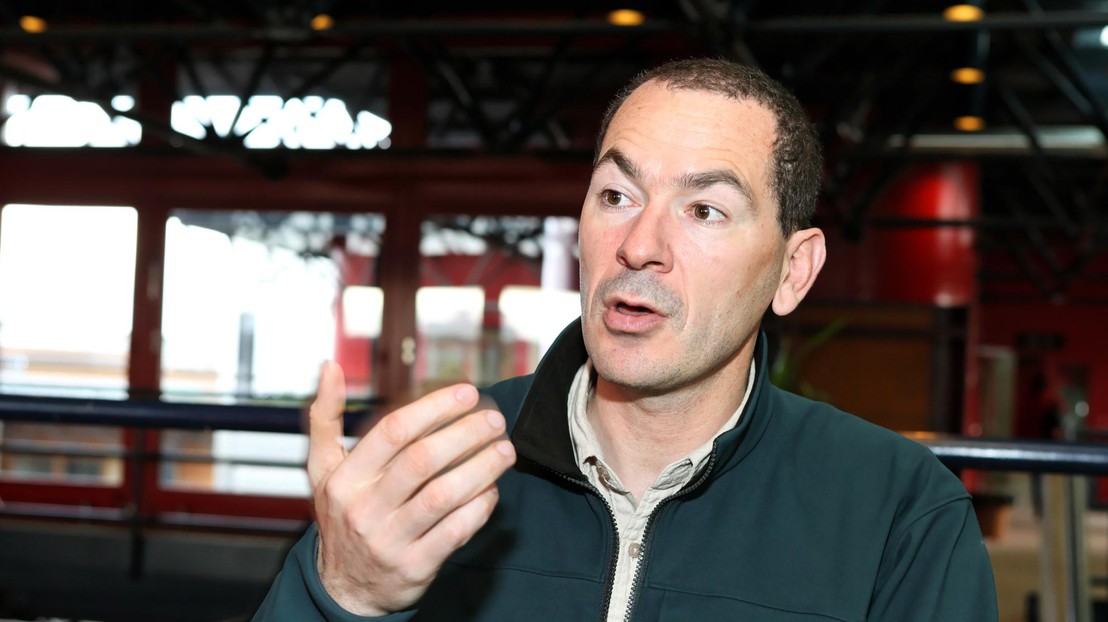
© Alain Herzog / EPFL
Christophe Ancey warns against relying blindly on the output of computational avalanche models to evaluate the safety of alpine areas. Recent events prove him right.
Christophe Ancey, head of EPFL’s Laboratory for Environmental Hydraulics, is an expert in modeling avalanches and other environmental flows. His research group develops complex numerical models to simulate avalanches, yet he remains convinced that models alone cannot entirely replace the keen eye and intuition of an expert. In a recent article, he showed that more sophisticated computational models of avalanches do not necessarily translate to better accuracy in their results. We met with him to discuss the difficulties in modeling and predicting avalanches.
On March 3rd, you were called on to provide your expert opinion on an avalanche that had come down a slope beneath a chairlift in Saint-François-Longchamp, France. What did you see when you arrived at the scene?
Christophe Ancey: I traveled to Saint-François-Longchamp the day after the avalanche had come down, as it was important to get a first-hand view of the site. At the bottom of the avalanche, the snow had accumulated six meters deep and two masts near the bottom chairlift station had been destroyed by the hydrostatic-like pressure exerted by the snow. As you can now see on youtube, the avalanche was a sliding avalanche. It had come down the slope so slowly that skiers had time to stop and film it!
Could this avalanche have been predicted?
By merely studying the slope, an experienced practitioner could have predicted the possibility of rare avalanche at that site and would have advised to build the chairlift station just outside the depression it was constructed in. But when the chairlift was planned 25 years ago, the risk was evaluated using methods of that time and deemed acceptable.
How can you evaluate avalanche risk?
In the beginning, avalanche risk was evaluated based on naturalist knowledge. Without any calculations, an expert could estimate the trajectory that an avalanche might take and wind up with the right conclusions, basing himself only on observations of the mountain. During the early twentieth century, the first mathematical models were developed; an avalanche was modeled as a sliding block, subject to the forces of gravity and friction. Though simple, this approach provided more quantitative insight than the naturalist approach. And in the 1970s the first computational avalanche models were developed, based on the hydraulic models used at the time. These models, which were popularized in the 1990s, yielded much more precise results than the previous approaches.
But the model output didn’t become more reliable...
Each time you zoom in and try to include more detail, you don’t just multiply problems by a factor, you raise them to a certain power. And there are several different classes of problems; cartographic ones, numerical ones, and problems related to the physics included in the model.
You recently authored an article with a surprising conclusion: for certain types of flows, the least detailed numerical models can yield the most accurate results.
Yes, for this article, we chose to work in the lab. We ran our experiments using Carbopol - basically hair gel - since it replicates many of the important properties of snow avalanches. We let the gel flow down an inclined plain and tracked it until it came to a halt. By comparing measurements of flow velocities, gel depth, and flow distance to predictions by three models of increasing complexity we found, intriguingly, that the crudest model gave the best results.
How would you explain that?
The numerical models we use were developed to study the dynamics of flows that were close to equilibrium, such as river flows on shallow slopes. In these situations, the force of gravity is balanced by the momentum flux and energy dissipation. In our case we are interested in studying flow behavior on steep slopes, where gravity and dissipation play a dominant role and for which part of the flow never reaches equilibrium. Due to their mathematical structure, the same equations that worked well on gentle slopes become difficult to solve numerically when certain terms such as gravity become large.
Wouldn’t you expect sophisticated models that resolve more of the physics to provide better results?
When you measure a noisy signal, such as the brightness of a flickering light bulb, one thing you can do is average it to eliminate some of the error. By adding more complexity to our models, we’re doing exactly the opposite, refining them more and more. When modeling so-called non-linear fluids such as hair gel or snow, small errors in one variable can propagate to other variables, strongly affecting their precision. So, the more you try to refine your model, and the more physics you try to include, the more you tend to increase these sources of errors.
Many extreme phenomena such as rogue waves seem to evade prediction. Will we ever be able to predict extreme avalanches?
Some extreme avalanches seem to follow different statistics than other events. With enough data, we should be able to quantify the probability of future extreme events statistically. But there is growing physical evidence that certain events differ radically from the others as they are able to modify the physical system in which they occur by, for example, switching their trajectory. Putting aside the scientific issues, there is also the question of what engineers and public authorities do with the model predictions - how they deal with risk. We may never be able to characterize the whole system and eliminate risk entirely at a reasonable cost, but we should strive to determine the probability of various scenarios. In essence, the question cannot simply be: Can it happen, but with what probability will it happen?
Pubilcation: Ancey, C., Andreini, N., Epely-Chauvin, G., Viscoplastic dambreak waves: review of simple computational approaches and comparison with experiments, Advances in Water Resources (2012), doi:10.1016/j.advwatres.2012.03.015