Machine learning helps retrace evolution of classical music
Researchers in EPFL’s Digital and Cognitive Musicology Lab in the College of Humanities used an unsupervised machine learning model to ‘listen to’ and categorize more than 13,000 pieces of Western classical music, revealing how modes – such as major and minor – have changed throughout history.
Many people may not be able to define what a minor mode is in music, but most would almost certainly recognize a piece played in a minor key. That’s because we intuitively differentiate the set of notes belonging to the minor scale – which tend to sound dark, tense, or sad – from those in the major scale, which more often connote happiness, strength, or lightness.
But throughout history, there have been periods when multiple other modes were used in addition to major and minor – or when no clear separation between modes could be found at all.
Understanding and visualizing these differences over time is what Digital and Cognitive Musicology Lab (DCML) researchers Daniel Harasim, Fabian Moss, Matthias Ramirez, and Martin Rohrmeier set out to do in a recent study, which has been published in the open-access journal Humanities and Social Sciences Communications. For their research, they developed a machine learning model to analyze more than 13,000 pieces of music from the 15th to the 19th centuries, spanning the Renaissance, Baroque, Classical, early Romantic, and late Romantic musical periods.
“We already knew that in the Renaissance [1400-1600], for example, there were more than two modes. But for periods following the Classical era [1750-1820], the distinction between the modes blurs together. We wanted to see if we could nail down these differences more concretely,” Harasim explains.
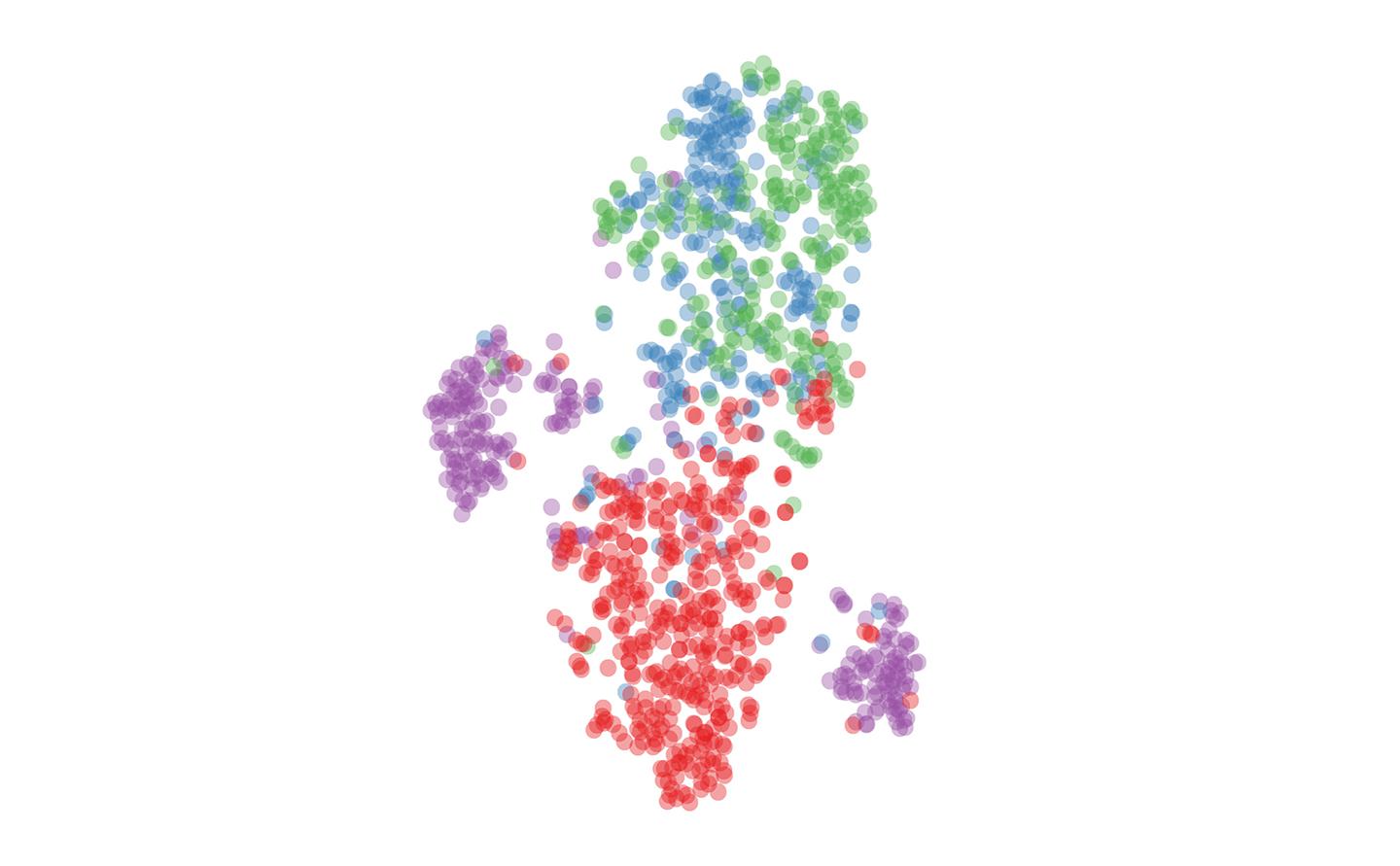
Machine listening (and learning)
The researchers used mathematical modeling to infer both the number and characteristics of modes in these five historical periods in Western classical music. Their work yielded novel data visualizations showing how musicians during the Renaissance period, like Giovanni Pierluigi da Palestrina, tended to use four modes, while the music of Baroque composers, like Johann Sebastian Bach, revolved around the major and minor modes. Interestingly, the researchers could identify no clear separation into modes of the complex music written by Late Romantic composers, like Franz Liszt.
Harasim explains that the DCML’s approach is unique because it is the first time that unlabeled data have been used to analyze modes. This means that the pieces of music in their dataset had not been previously categorized into modes by a human.
“We wanted to know what it would look like if we gave the computer the chance to analyze the data without introducing human bias. So, we applied unsupervised machine learning methods, in which the computer 'listens' to the music and figures out these modes on its own, without metadata labels.”
Although much more complex to execute, this “unsupervised” approach yielded especially interesting results which are, according to Harasim, more “cognitively plausible” with respect to how humans hear and interpret music.
“We know that musical structure can be very complex, and that musicians need years of training. But at the same time, humans learn about these structures unconsciously, just as a child learns a native language. That’s why we developed a simple model that reverse engineers this learning process, using a class of so-called Bayesian models that are used by cognitive scientists, so that we can also draw on their research.”
From class project to publication…and beyond
Harasim notes with satisfaction that this study has its roots in a class project that he and his co-authors Moss and Ramirez did together as students in EPFL professor Robert West’s course, Applied Data Analysis. He hopes to take the project even further by applying their approach to other musical questions and genres.
“For pieces within which modes change, it would be interesting to identify exactly at what point such changes occur. I would also like to apply the same methodology to jazz, which was the focus of my PhD dissertation, because the tonality in jazz is much richer than just two modes.”
Harasim, D., Moss, F.C., Ramirez, M., Rohrmeier, M. Exploring the foundations of tonality: statistical cognitive modeling of modes in the history of Western classical music. Humanit Soc Sci Commun 8, 5 (2021). https://doi.org/10.1057/s41599-020-00678-6
Data and code on Github: https://github.com/DCMLab/HistoryModes_DataCode