Low-Cost Deepfake Detection Paper Accepted by Electronic Imaging'24
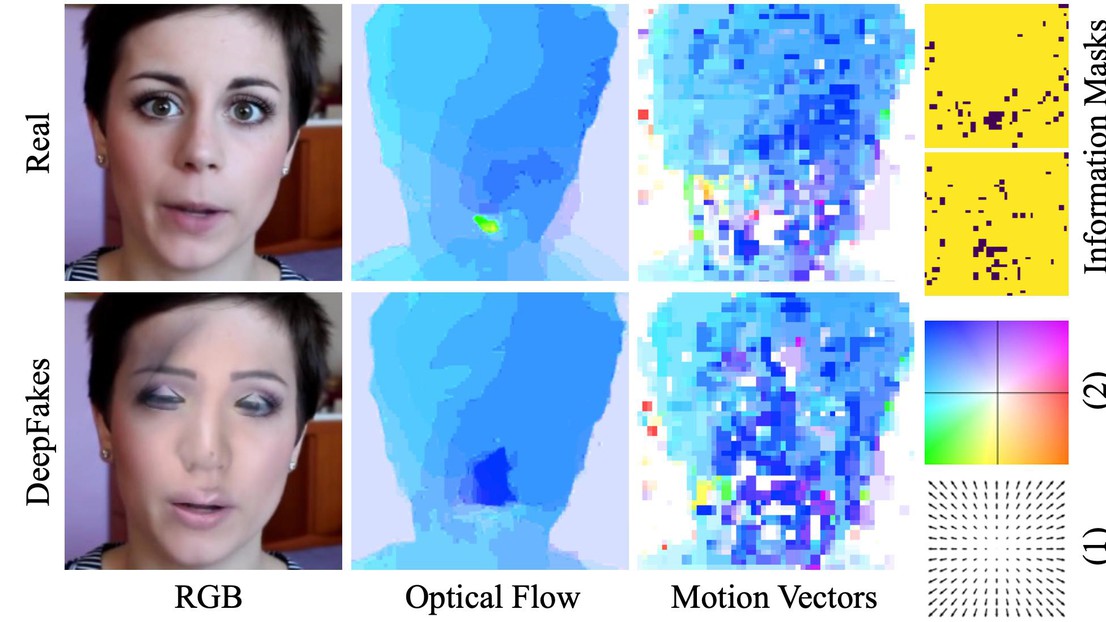
© 2023 EPFL
Our paper 'Efficient Temporally-Aware DeepFake Detection using H.264 Motion Vectors' was accepted by the conference Electronic Imaging 2024 for oral presentation. Check out a nice blog by Martin Anderson and Metaphysic.ai explaining it.
Video DeepFakes are fake media created with Deep Learning (DL) techniques that manipulate a person's expression or identity. Most current DeepFake detection methods analyze each frame independently but often overlook inconsistencies and unnatural movements between frames. Some newer methods employ optical flow models to capture this temporal aspect, but they are computationally expensive. However, some newer methods that use optical flow models to capture this temporal aspect are computationally expensive.
Our paper 'Efficient Temporally-Aware DeepFake Detection using H.264 Motion Vectors' proposes using the related but often ignored Motion Vectors (MVs) and Information Masks (IMs) from the H.264 video codec, to detect temporal inconsistencies in DeepFakes. Our experiments demonstrate this approach’s effectiveness and minimal computational costs compared to per-frame RGB-only methods. This could lead to new, real-time temporally-aware DeepFake detection methods for video calls and streaming.
Come and check out our live presentation in San Francisco at the Electronic Imaging conference on the 22.-25 January 2024. This paper is authored by IVRL members Peter Grönquist*, Yufan Ren*, Qingyi He, Alessio Verardo, and Sabine Süsstrunk, as our continued effort in DeepFakes detection research. For further information, see our IVRL’s participation in Singapore’s Trusted Media Challenge [link].
The paper: Efficient Temporally-Aware DeepFake Detection using H.264 Motion Vectors
Metaphysic.ai's post: Low-Cost Deepfake Video Detection With H.264 Motion Vectors