Katie Bouman, the scientist who reveals the invisible
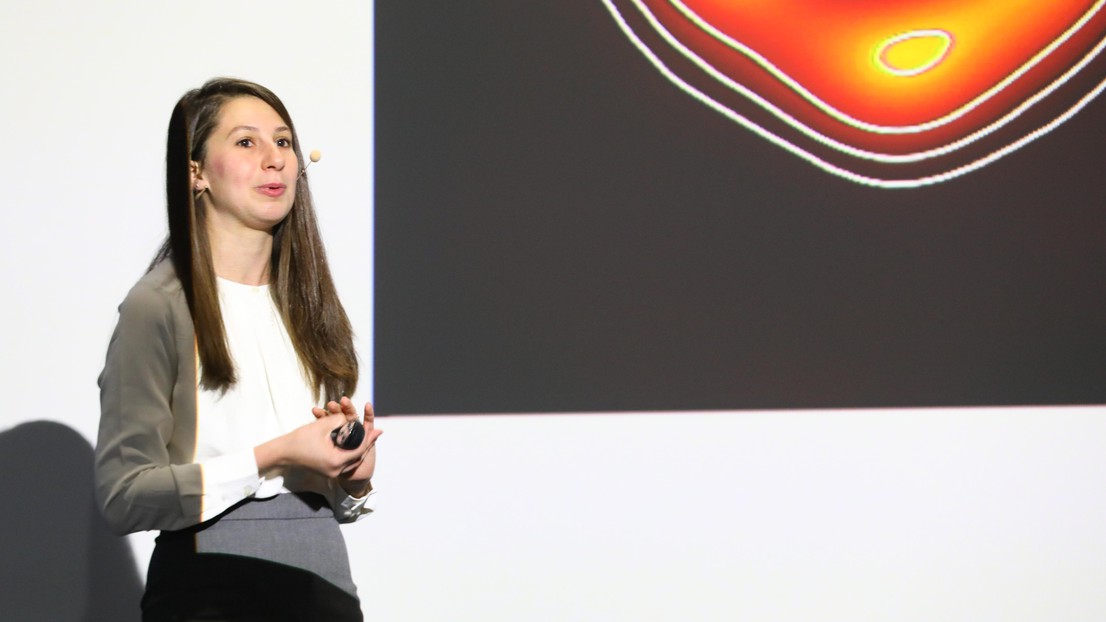
Katie Bouman presented her work during the EPFL Open Science Day. © EPFL / Murielle Gerber
The first-ever image of a black hole was unveiled to the public this past April. It was produced by a team of 200 scientists as part of the Event Horizon Telescope Project. Katie Bouman, an assistant professor at Caltech, was at the center of the action. On a recent visit to EPFL, she talked about computer science with President Martin Vetterli.
Katie Bouman was one of 200 scientists who helped create the first-ever image of a black hole, released this past April. The 30-year old researcher, who was recently named an assistant professor of computing and mathematical sciences at the California Institute of Technology (CalTech), came to speak at EPFL’s Open Science Day. Martin Vetterli took advantage of that opportunity to sit down with her and ask her a few questions about their field of shared interest.
- MV: “It’s pretty unusual for someone to become a star researcher at such a young age. How did you get into science?
KB: I was very interested in science as a kid. When I was in third grade, I remember looking under rocks all the time. And as I got older, I was constantly drawn to the different science topics covered in class. In sixth grade, I first got involved in science fair projects, and I continued doing that on and off through high school. Then, when I tried out research for the first time, I was thrilled: it was so different from a homework set, where you know there’s a solution even if it’s difficult to find. Research was a new way of thinking and problem-solving for me, and I found that very exciting.
- How did you get into computer science?
It wasn’t until high school, when a friend convinced me to take a computer science class. At first, I thought: “This isn’t very fun, what will I use it for?” But it opened up a totally new area for me! We didn’t solve any big problems or anything, but because I had done this class and learned this new computing language, I was offered a position the following summer to work in a lab at Purdue University, in my hometown, helping the graduate researchers with their projects. Some were working on imaging problems, such as in the field of forensics. It was so exciting to see how we could pull information out of images and use it to recover hidden properties about the world. From that point on, I got more and more interested in imaging and electrical engineering and eventually computer vision. My path has always been guided by a love of research and images, because I really like the fact that you can visualize what you’re working on.
- The black-hole imaging project was very cross-disciplinary. What was it like to work on this team?
It was quite a new experience, and I had a lot of fun! At that point, the team had already worked extensively on the instrumentation side and was just getting started on the imaging part. They really needed someone who could interpret the data and develop new ways of dealing with the challenges. I first heard about the project through a presentation and, actually, I understood pretty much nothing, it was like gibberish [laughter]. Still, I left that presentation thinking “I want to be part of this!”, because I could see that the tools needed for this project were so similar to the ones we were developing for other problems, like in medical imaging. When I joined the team, I didn’t know anything about black holes. But I was working with an amazing group of researchers. They kindly taught me everything I needed to know about radioastronomy, astrophysics, black holes, and so on. I also got to spend about a month and a half behind a telescope learning about the technical system. And I think that process was important, because when you’re developing an imaging method, you have to understand not only how problems arise during observations and make their way into the data you’re analyzing, but also what you can expect to find. In order to get the most out of the data, you need to learn as much as possible in the related fields. That’s why working in cross-disciplinary teams is so crucial now for many big science projects to go forward.
- Helping create the first image of a black hole is a major accomplishment so early in your career. What’s next for you as a young researcher at CalTech?
I’m really excited to do new things. For example, I’m starting to work on seismology – trying to image earthquakes – which is crucial for the California region. I’m very excited about this job because CalTech is a small school, which makes it easier to connect with people in other fields. I’m so fortunate to already be working with a lot of people in different areas. My ultimate goal is to think about how we can help scientists discover new things. We are so used to coming up with our own hypotheses and figuring out what we should observe to test them. But we can now develop machines to find trends and data that we might not see or be aware of as humans. I’m really interested in how to design machines that can tell us how to do our experiments, and what new wavelength or data domain to look at in order to discover something we would not otherwise see. In general, I’m very much into how we do data-driven scientific discovery.
- A couple of guys just won the Nobel Prize for discovering the first exoplanet 25 years ago. Initially, they didn’t believe their results and reran the experiment several times. Some things have changed since then, but we’re still dealing with noisy data, right?
Yes, when we first created the black-hole image, or at least the day we got the data, we entered it into an imaging script and a ring appeared. I actually went from being really excited to being mad at the colleagues who gave us the data – which I was convinced were fake. It just seemed too good to be true! Nothing ever works like that the first time. It was just so much more beautiful than any synthetic data we had created. It actually took weeks before I believed the data were real, and that our colleagues weren’t trying to trick us or test us.