IBM Research Award 2019 – Cosimo Aprile
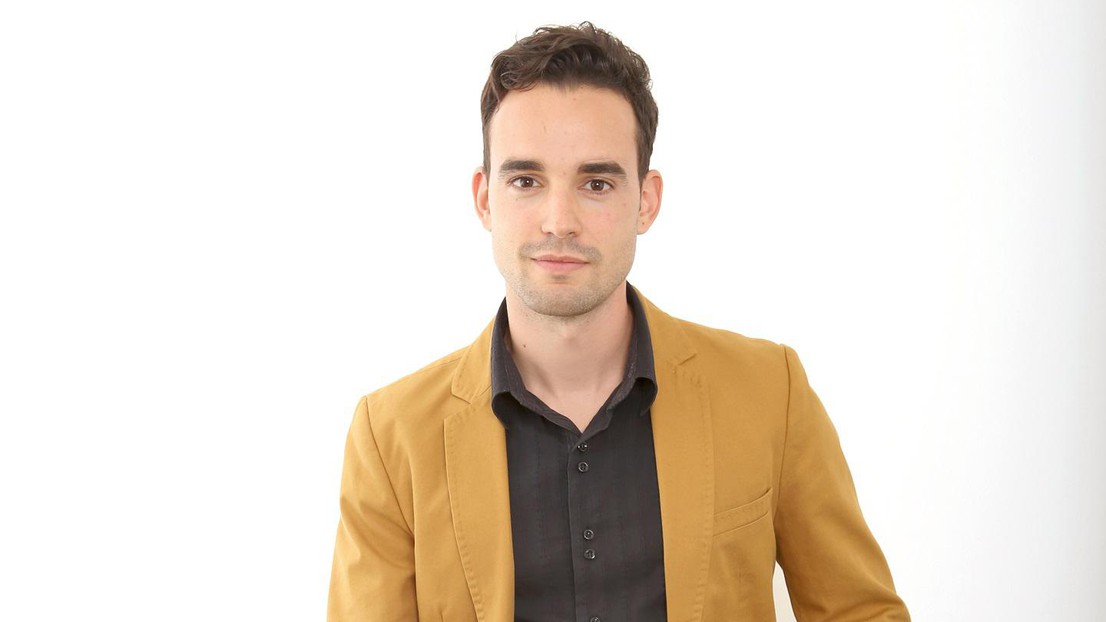
© 2019 EPFL - Cosime Aprile
Learning-Based Hardware Design for Data Acquisition Systems
EPFL thesis n°8693 (2018)
Thesis directors: Prof. V. Cevher, Prof. Y. Leblebici
"For his outstanding contributions in merging machine learning theory with circuit design towards energy efficient neural implants, as well as for developing new insights in boosting high-speed computer-to-computer communications."
This multidisciplinary research project aims to investigate the optimized information extraction from signals or data volumes and to develop tailored hardware implementations that trade-off the complexity of data acquisition with that of data processing, conceptually allowing radically new device designs.
This work demonstrates our contention in hardware prototypes, for two different fields of application as edge and big-data computing.
In the framework of edge-data computing, such as wearable and implantable ecosystems, the power budget is defined by the battery capacity, which generally limits the device performance and usability.
Based on our new mathematical foundations, we built different prototypes to get a neural signal acquisition chip that rigorously trades off its area, energy consumption, and the quality of its signal output, outperforming the state-of-the-art in all aspects.
In the framework of big-data and high-performance computation, such as in high-end servers application, the RF circuits meant to transmit data from chip-to-chip or chip-to-memory are defined by low power requirements.
In this work, we have developed a learning-based approach that allows a versatile receiver circuit, which not only copes with large channel attenuation but also implements novel crosstalk reduction techniques to allow single-ended multiple lines transmission, without sacrificing its overall bandwidth for a given area within the interconnect's data-path.