Hamiltonian Deep Neural Networks are Universal Approximators!
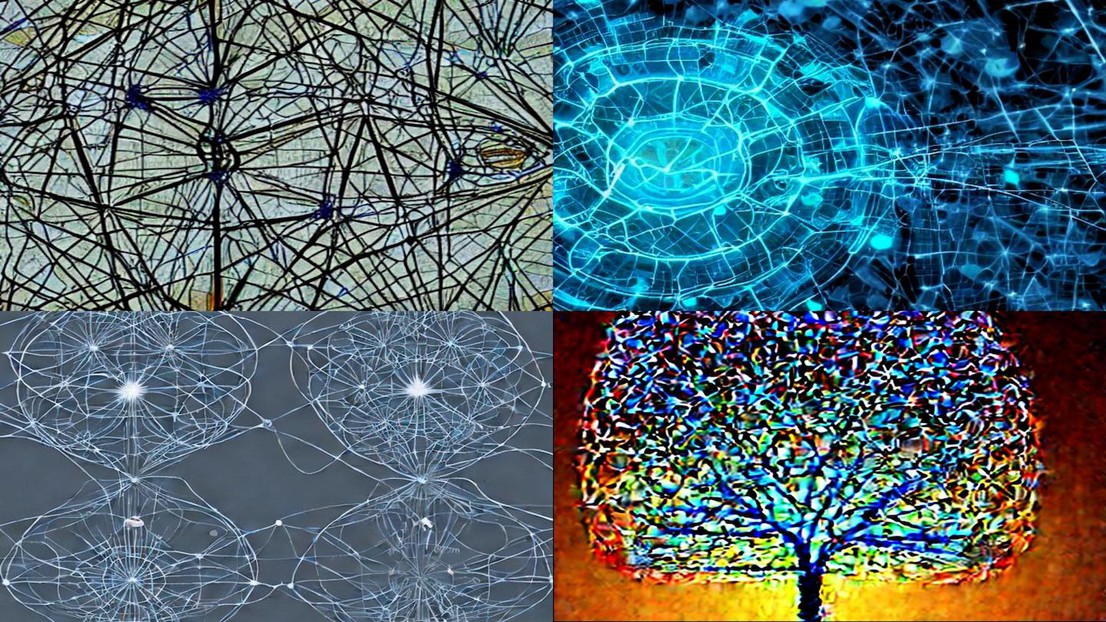
© 2023 EPFL
Our paper "Universal Approximation Property of Hamiltonian Deep Neural Networks" is accepted in L-CSS.
Our paper investigates the universal approximation capabilities of Hamiltonian Deep Neural Networks (HDNNs) that arise from the discretization of Hamiltonian Neural Ordinary Differential Equations.
Recently, it has been shown that HDNNs enjoy, by design, non-vanishing gradients, which provide numerical stability during training. However, although HDNNs have demonstrated state-of-the-art performance in several applications, a comprehensive study to quantify their expressivity is missing. In this regard, we provide a universal approximation theorem for HDNNs and prove that a portion of the flow of HDNNs can approximate arbitrary well any continuous function over a compact domain. This result provides a solid theoretical foundation for the practical use of HDNNs.
This research is supported by the Swiss National Science Foundation under the NCCR Automation (grant agreement 51NF40 180545).