ETHOS presents two papers at i3CE 2024
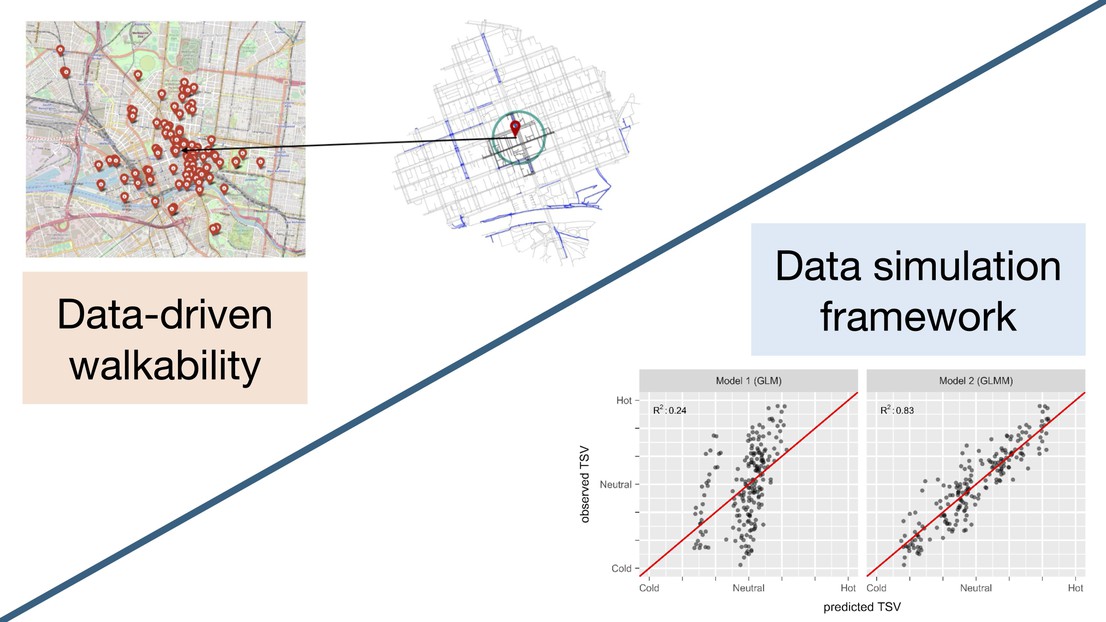
© ETHOS Lab 2024 EPFL
ETHOS presented two papers at the ASCE International Conference on Computing in Civil Engineering (i3CE), hosted by Carnegie Mellon University.
Kanaha Shoji presented our work on data-driven urban walkability. With an increasing urban population and a growing demand for sustainable mobility, there is a need to understand what drives walkability and walking behavior. Despite numerous studies exploring urban walkability, a notable challenge lies in substantiating these investigations with empirical data. In this paper, we address this research gap by leveraging open-source data on pedestrian counts, urban form features, and weather data. We use random forest regression to predict pedestrian counts using temporal, urban form, and weather as well as population and visual features, and we quantify the importance of each feature using the percent increase in mean square error when the feature is randomly permuted. We observe distinct temporal patterns in walking behavior and diverse contributions of urban form features. Ultimately, this work is a first step toward a data-driven and empirical understanding of the associations between walking behavior and the urban environment, which, in the end, will provide engineers, designers, and planners with improved tools for decision-making on advancing sustainable mobility.
Matteo Favero presented our work on a data simulation framework for statistical analysis in human-building interaction research. Designing and operating buildings that are safe, comfortable, and conducive to productivity is essential for promoting human well-being. Human-centred approaches are gaining popularity, especially in addressing factors that influence well-being and building performance (e.g., thermal comfort). Unfortunately, statistical practices for analysing thermal comfort data often rely on simplified assumptions, resulting in crucial aspects of data analysis being often absent or overlooked. A common phenomenon in thermal comfort research is ignoring the clustering of observations, and methods that do not account for clustering (e.g., single-level models) are often used. This study proposes a synthetic data simulation workflow to investigate the tangible effects of ignoring clustering in the analysis of thermal comfort data. The results show that a multilevel model is more effective for predictions and produces more reasonable inferences compared with a single-level model. Overall, the results are relevant for improving the development and interpretation of thermal comfort models. Ultimately, this research aims to bridge the gap between current practices in statistical analysis and building research to promote better inferences of complex human-building interactions.