ETHOS presents new research at BuildSys conference
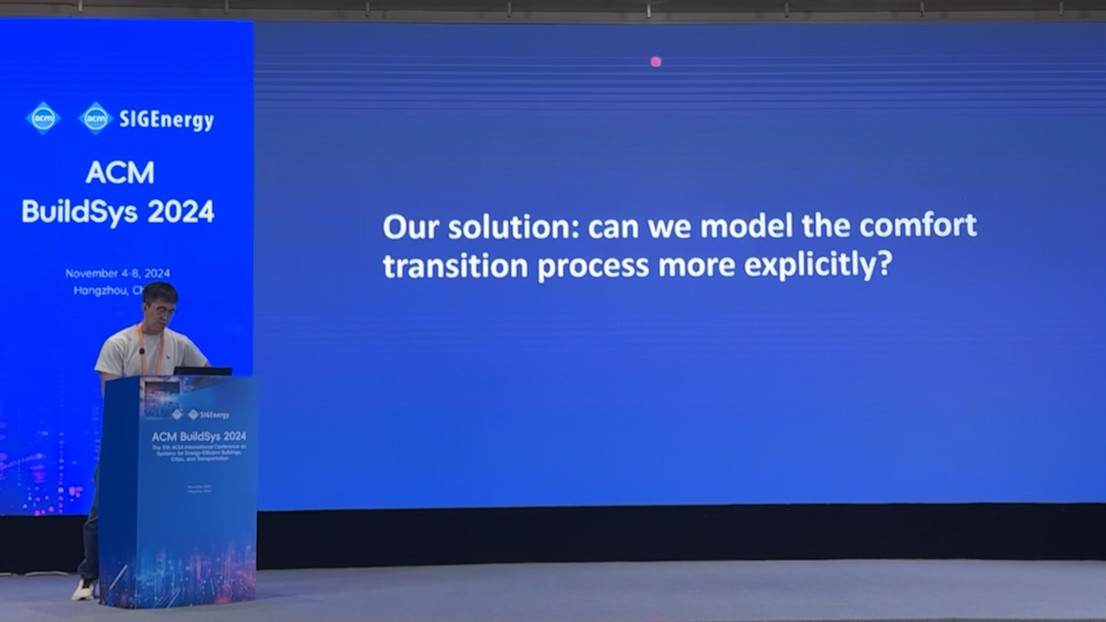
© 2024 Andrew Sonta EPFL
ETHOS presented two papers on occupant-centric energy and comfort modeling at the 2024 BuildSys conference, held in Hangzhou, China.
At the main conference, Yufei Zhang presented our work on data-driven modeling of personal comfort states in buildings. Recent advances in personalized sensing and comfort feedback have spurred the development of data-driven comfort models tailored to individual needs. However, because current models treat sequential comfort feedback independently, they are subject to unstable predictions and limited interpretability, hindering their deployment in building management. This study introduced a dynamic modeling framework that utilizes a Neural Ordinary Differential Equations-based Continuous-time Markov Chain to model the transitions in comfort states over time. Our modeling approach, developed through a field study utilizing smart glasses and mobile app feedback, tracks occupants' comfort transitions across daily activities and contexts. The results demonstrate that this model not only predicts comfort states more accurately and stably than conventional classification models but also uniquely provides a representation of how the hazards of state transitions are influenced by changing ambient and contextual conditions. This approach, therefore, offers a new perspective on personalized building control, where predictions of comfort transition hazards can preemptively suggest building management interventions to avoid occupants experiencing discomfort. In addition, insights into how environmental and contextual characteristics relate to these hazards can guide holistic management strategies that dynamically balance comfort with energy targets in response to the occupants' activities and contexts. The work was collaborative with the University of Fribourg, MIT, and the National University of Singapore.
At the conference's workshop on big data and machine learning for smart buildings and cities, Yufei Zhang also presented new work on occupant-centric energy flexibility. Building demand flexibility is essential for balancing the grid as renewable energy generation increases. However, the limited adoption of flexibility-enabling technologies underscores the need for scalable solutions to evaluate flexibility. Despite increasing availability of building data and scalable data-driven methods, these tools often fail to capture critical what-if scenarios for building operations, market conditions, and potential impacts on occupant comfort. To address these challenges, we propose a data-driven framework to assess HVAC-related demand flexibility in commercial buildings. Using only smart metering data, our approach integrates physics-based insights with data-driven techniques to deliver credible what-if scenarios and occupant-centric operation. Our tool has three main features: (1) generating representative scenarios of load profiles based on weather conditions and inferred occupancy level; (2) capturing general indoor temperature behavior to guide comfort-related decisions without precise measurements; and (3) introducing an occupant-centric flexibility envelope, explicitly quantifying trade-offs between power levels, duration, and occupant satisfaction in flexible operation. We showcase the implementation of this framework using a real-world office building dataset, demonstrating both its accuracy and adaptivity for demand flexibility evaluations. This work marks a significant step toward scalable, efficient, and credible data-driven flexibility assessments. It provides a versatile tool for building operators and market designers, delivering tailored solutions for individual buildings while scaling across large portfolios. This work was collaborative with the Hong Kong Polytechnic University.