EPFL papers @ ICML 2023
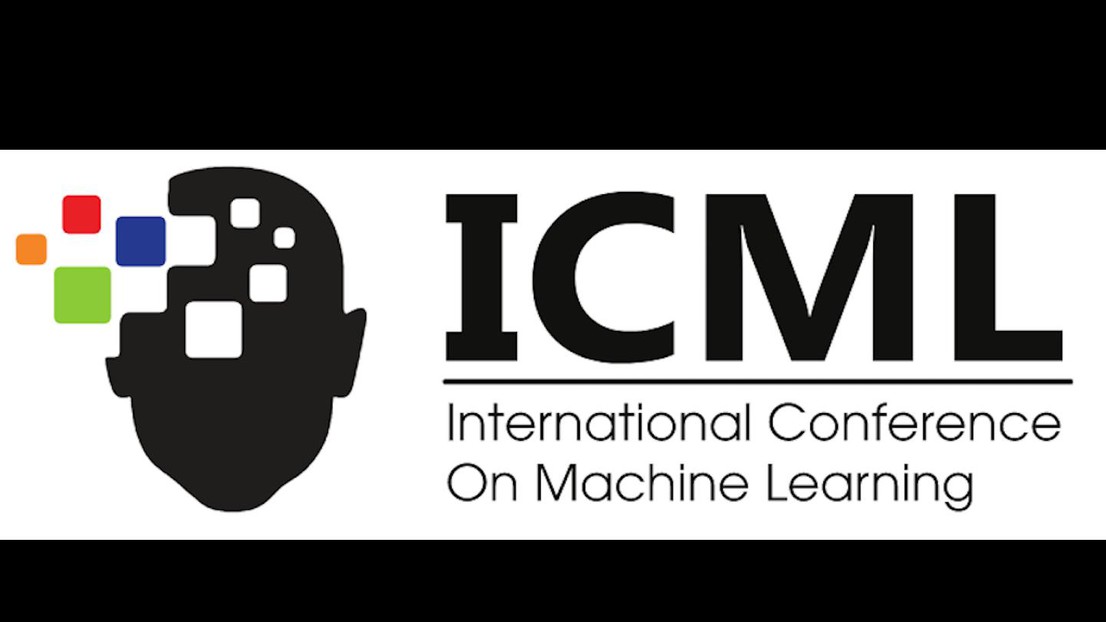
ICML logo © ICML
The following EPFL papers have been accepted to ICML 2023 (Fortieth International Conference on Machine Learning).
The conference will be held from July 23-29, 2023 in Honolulu, Hawaii, USA
ICML 2023
Below is a list of ICML 2023 papers with at least one EPFL author:
1. Provable benefits of general coverage conditions in efficient online reinforcement learning with function approximation by Fanghui Liu, Luca Viano, Volkan Cevher.
2. Benign Overfitting in Deep Neural Networks by Zhenyu Zhu, Fanghui Liu, Grigorios Chrysos, Francesco Locatello, Volkan Cevher.
3. Semi Bandit dynamics in Congestion Games: Convergence to Nash Equilibrium and No-Regret Guarantees by Ioannis Panageas, Efstratios Skoulakis, Luca Viano, Xiao Wang, Volkan Cevher.
4. When do Minimax-fair Learning and Empirical Risk Minimization Coincide? by Harvineet Singh, Matthäus Kleindessner, Volkan Cevher, Rumi Chunara, Chris Russell
5. On Pitfalls of Test-time Adaptation by Hao Zhao, Yuejiang Liu, Alexandre Alahi, Tao Lin
6. Are Gaussian data all you need? Extents and limits of universality in high-dimensional generalized linear estimation by Luca Pesce, Florent Krzakala, Bruno Loureiro, Ludovic Stephan
7. Optimal Learning of Deep Random Networks of Extensive-width by Hugo Cui, Florent Krzakala, Lenka Zdeborová
8. Deterministic equivalent and error universality of deep random features learning by Dominik Schröder, Hugo Cui, Daniil Dmitriev, Bruno Loureiro
9. Robust Collaborative Learning with Linear Gradient Overhead by Sadegh Farhadkhani, Rachid Guerraoui, Nirupam Gupta, Lê-Nguyên Hoang, Rafael Pinot, John Stephan
10. Distributed Learning with Curious and Adversarial Machines by Youssef Allouah, Rachid Guerraoui, Nirupam Gupta, Rafael Pinot, John Stephan
11. SGD with large step sizes learns sparse features by Maksym Andriushchenko, Aditya Varre, Loucas Pillaud-Vivien, Nicolas Flammarion
12. A modern look at the relationship between sharpness and generalization by Maksym Andriushchenko, Francesco Croce, Maximilian Müller, Matthias Hein, Nicolas Flammarion
13. What can be learnt with wide convolutional networks? by Francesco Cagnetta, Alessandro Favero, Matthieu Wyart
14. Dissecting the Effects of SGD Noise in Distinct Regimes of Deep Learning by Antonio Sclocchi, Mario Geiger, Matthieu Wyart
15. When does privileged information explain away label noise? by Guillermo Ortiz-Jimenez, Mark Collier, Ananat Nawalgaria, Alexander D'Amour, Jesse Berent, Rodolphe Jenatton, Effrosyni Kokiopoulou
16. Towards Stable and Efficient Adversarial Training againts $l_1$ Bounded Adversarial Attacks by Yulun Jiang*, Liu Chen*, Zhichao Huang, Mathieu Salzmann, Sabine Süsstrunk
17. Identifiability and generalizability in constrained inverse reinforcement learning by Andreas Schlaginhaufen, Maryam Kamgarpour
18. Revisiting Gradient Clipping: Stochastic bias and tight convergence guarantees by Anastasia Koloskova, Hadrien Hendrikx, Sebastian U. Stich
19. Second-order optimization with lazy Hessians by Nikita Doikov, El Mahdi Chayti, Martin Jaggi
20. Special Properties of Gradient Descent with Large Learning Rates by Amirkeivan Mohtashami, Martin Jaggi, Sebastian Stich
21. End-to-End Learning for Stochastic Optimization: A Bayesian Perspective by Yves Rychener, Daniel Kuhn and Tobias Sutter