EPFL Doctorate Award - 2023 - Arthur Jacot
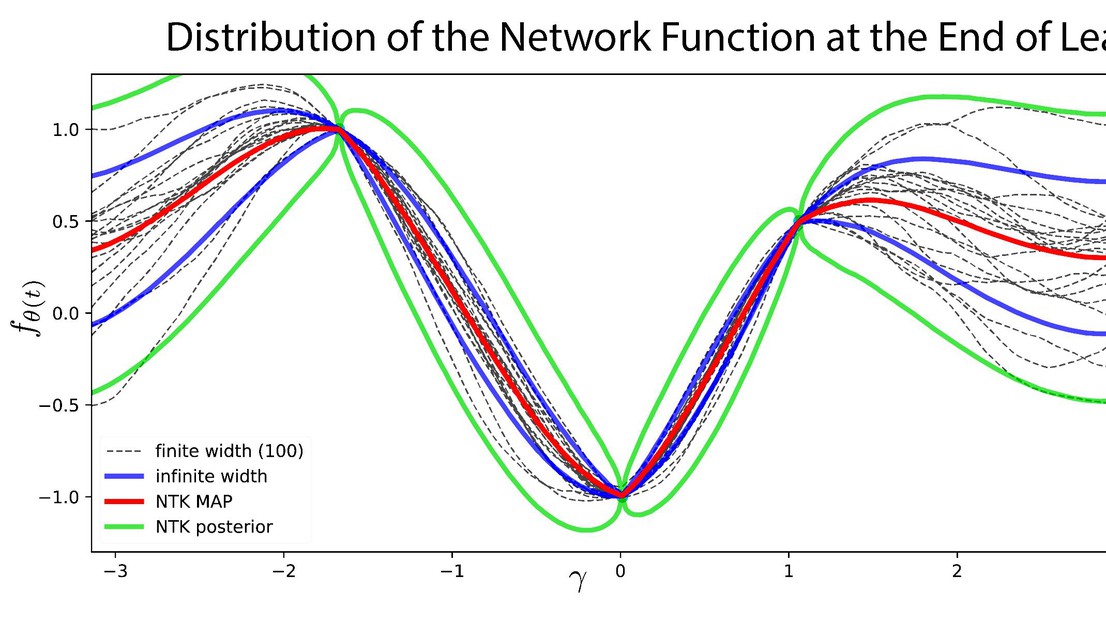
©
Theory of Deep Learning: Neural Tangent Kernel and Beyond
EPFL thesis n°9825
Thesis director: Prof. C. Hongler
For his ground-breaking contributions to the mathematical theory of Deep Learning, in particular the invention of the Neural Tangent Kernel, which have revolutionised our understanding of Artificial Neural Networks
Deep Neural Networks (DNNs) are at the forefront of today's AI revolution, but we still have not identified what allows them to learn tasks that stump other statistical models. Arthur Jacot's thesis made a significant step toward a mathematical theory of DNNs by introducing the Neural Tangent Kernel (NTK). The NTK approximates the nonlinear model of DNNs by a linear model. In the limit as the number of artificial neurons grows to infinity, this approximation becomes exact, yielding surprisingly simple training dynamics, and leading to the first precise description of the convergence and generalization of DNNs in terms of the spectrum of the NTK. By analyzing how different architecture choices affect the NTK, one can design and use DNNs in a principled manner, backed by theory.