EPFL doctorate Award 2018 – Julien Fageot
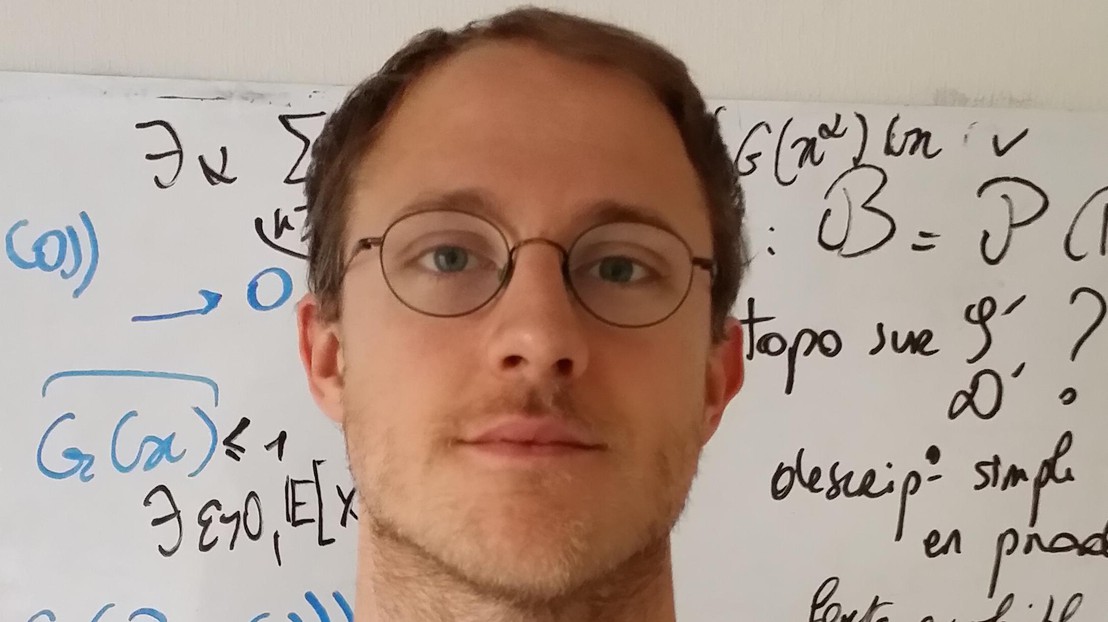
© 2018 EPFL
Gaussian versus Sparse Stochastic Processes: Construction, Regularity, Compressibility
EPFL thesis n°7657 (2017)
Thesis director: Prof. M. Unser
"For his fundamental contributions to the theory of sparse stochastic processes and their application to image and signal processing."
Signal processing is the science that studies, at an abstract level, the analysis and the interpretation of signals. Basic methods are based on a rigorous and elegant formalism, which includes frequency analysis and Gaussian-based statistical models. However, these methods have shown to be unable to catch an essential characteristic of naturally-occuring signals: their sparsity. A signal is sparse if it is possible to describe it efficiently with a limited amount of information. This observation brings many thrilling challenges, including to introduce mathematical models that are coherent with the sparsity.
In my thesis, I realized an in-depth study of a mathematical model for analog sparse signals. We see a signal as the realisation of a random process characterized by its unpredictable part, called the innovation, and a differential operator, which structures its spatio-temporal dependance. My work culminated with the rigorously quantification of the effective compressibility of a broad variety of random models, far beyond the classical Gaussian hypothesis. We now understand the reasons why Gaussian models are unable to capture the sparsity of signals, and why the non-Gaussian Lévy models are excellent candidates to model high compressibility. This theoretical work is moreover useful to develop new algorithms for the reconstruction of sparse signals.