Enhanced AI tracks neurons in moving animals
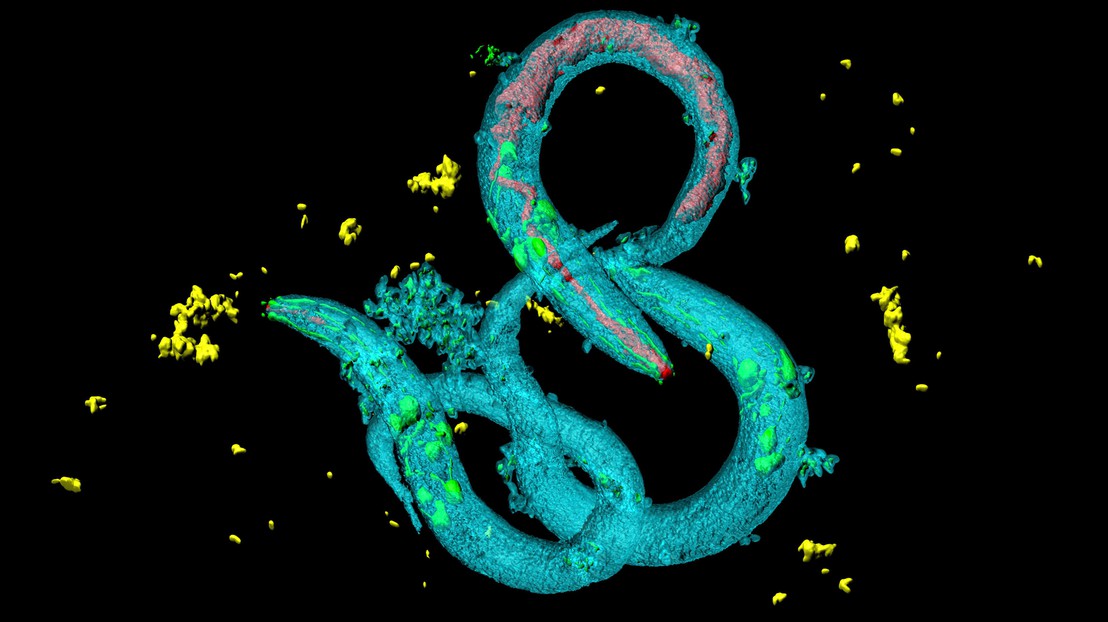
Caenorhabditis elegans © iStock (HeitiPaves)
EPFL and Harvard scientists have developed a pioneering new method that uses deep learning and a new technique called ‘targeted augmentation’ to track neurons in moving and deforming animals.
Recent advances allow imaging of neurons inside freely moving animals. However, to decode circuit activity, these imaged neurons must be computationally identified and tracked. This becomes particularly challenging when the brain itself moves and deforms inside an organism’s flexible body, e.g. in a worm. Until now, the scientific community has lacked the tools to address the problem.
Now, a team of scientists from EPFL and Harvard have developed a pioneering AI method to track neurons inside moving and deforming animals. The study, now published in Nature Methods, was led by Sahand Jamal Rahi at EPFL’s School of Basic Sciences.
The new method is based on a convolutional neural network (CNN), which is a type of AI that has been trained to recognize and understand patterns in images. This involves a process called “convolution”, which looks at small parts of the picture – like edges, colors, or shapes – at a time and then combines all that information together to make sense of it and to identify objects or patterns.
The problem is that to identify and track neurons during a movie of an animal’s brain, many images have to be labeled by hand because the animal appears very differently across time due to the many different body deformations. Given the diversity of the animal’s postures, generating a sufficient number of annotations manually to train a CNN can be daunting.
To address this, the researchers developed an enhanced CNN featuring ‘targeted augmentation’. The innovative technique automatically synthesizes reliable annotations for reference out of only a limited set of manual annotations. The result is that the CNN effectively learns the internal deformations of the brain and then uses them to create annotations for new postures, drastically reducing the need for manual annotation and double-checking.
The new method is versatile, being able to identify neurons whether they are represented in images as individual points or as 3D volumes. The researchers tested it on the roundworm Caenorhabditis elegans, whose 302 neurons have made it a popular model organism in neuroscience.
Using the enhanced CNN, the scientists measured activity in some of the worm’s interneurons (neurons that bridge signals between neurons). They found that they exhibit complex behaviors, for example changing their response patterns when exposed to different stimuli, such as periodic bursts of odors.
The team have made their CNN accessible, providing a user-friendly graphical user interface that integrates targeted augmentation, streamlining the process into a comprehensive pipeline, from manual annotation to final proofreading.
“By significantly reducing the manual effort required for neuron segmentation and tracking, the new method increases analysis throughput three times compared to full manual annotation,” says Rahi. “The breakthrough has the potential to accelerate research in brain imaging and deepen our understanding of neural circuits and behaviors.”
Other contributors
- Swiss Data Science Center
EPFL
Helmut-Horten Foundation
Swiss Data Science Center
Core Francisco Park, Mahsa, Barzegar Keshteli, Kseniia Korchagina, Ariane Delrocq, Vladislav Susoy, Corinne L. Jones, Aravinthan D. T. Samuel, Sahand Jamal Rahi. Automated neuron tracking inside moving and deforming animals using deep learning and targeted augmentation. Nature Methods 05 December 2023. DOI: 10.1038/s41592-023-02096-3