Emmanuel Abbé receives Frontier of Science Award
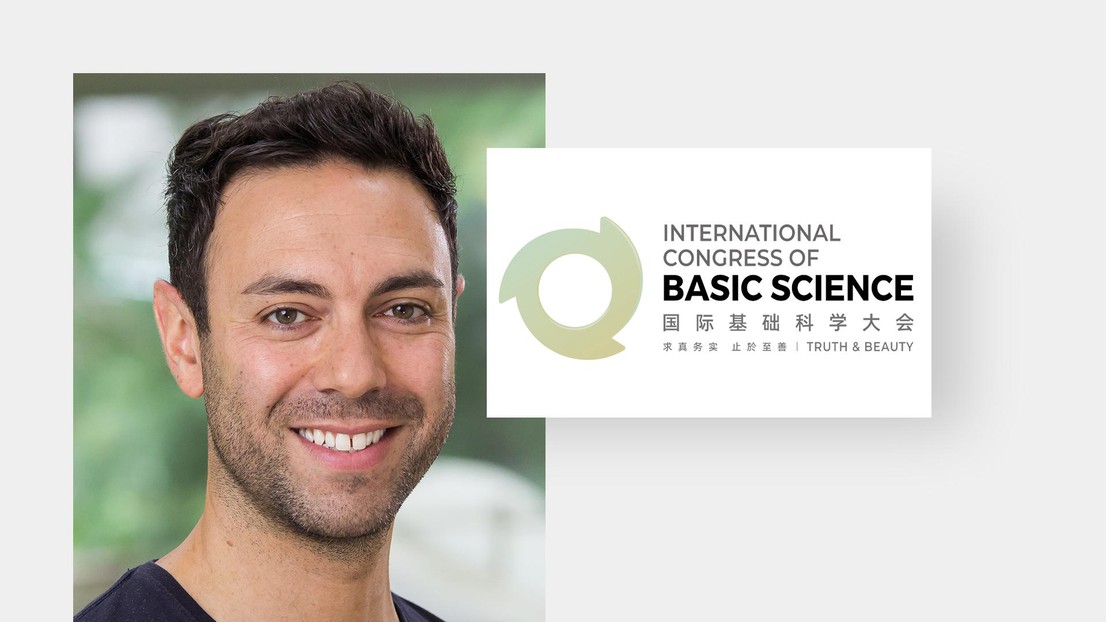
Emmanuel Abbé. Credit: EPFL
EPFL Professor Emmanuel Abbé has been honored with the 2024 Frontier of Science Award for his pioneering work on principal component analysis and spectral clustering.
The Frontier of Science Awards (FSA) are presented by the International Congress for Basic Science to honor top research achievements from the past five years that demonstrate exceptional scholarly value and originality.
Each awarded work must be published within the last five years, exhibit high scientific impact, and be evaluated and accepted by experts in its field.
Professor Emmanuel Abbé at EPFL has been awarded the 2024 FSA in the field of mathematics, category of statistics, for his work on Principal Component Analysis (PCA) and spectral clustering.
PCA is a mathematical tool used to simplify large sets of data by finding patterns and reducing the data to its most important features, making it easier to analyze and visualize. Spectral clustering is a method that groups data points into clusters based on their similarities by turning the data into a graph and finding connections between the points, helping to identify natural groups or patterns in the data.
The FSA recognizes Abbé’s 2022 paper, An ℓp Theory of PCA and Spectral Clustering, co-authored with Jianqing Fan (Princeton University) and Kaizheng Wang (Columbia University), and published in The Annals of Statistics.
ℓp perturbation theory is a mathematical method that helps to analyze how small changes or "noise" in data affect the results of a process, making it possible to improve the accuracy of statistical estimations even when the data is noisy or messy. The award-winning paper develops an ℓp perturbation theory for PCA that allows for more accurate analysis in conditions where traditional PCA struggles.
The results have far-reaching implications for machine learning and data analysis, particularly in high-noise, high-dimensional environments. The research introduces a novel perturbation theory that significantly improves PCA's ability to perform under noisy conditions, providing new insights into data dimensionality reduction.