Does one simple rule underlie learning in the brain?
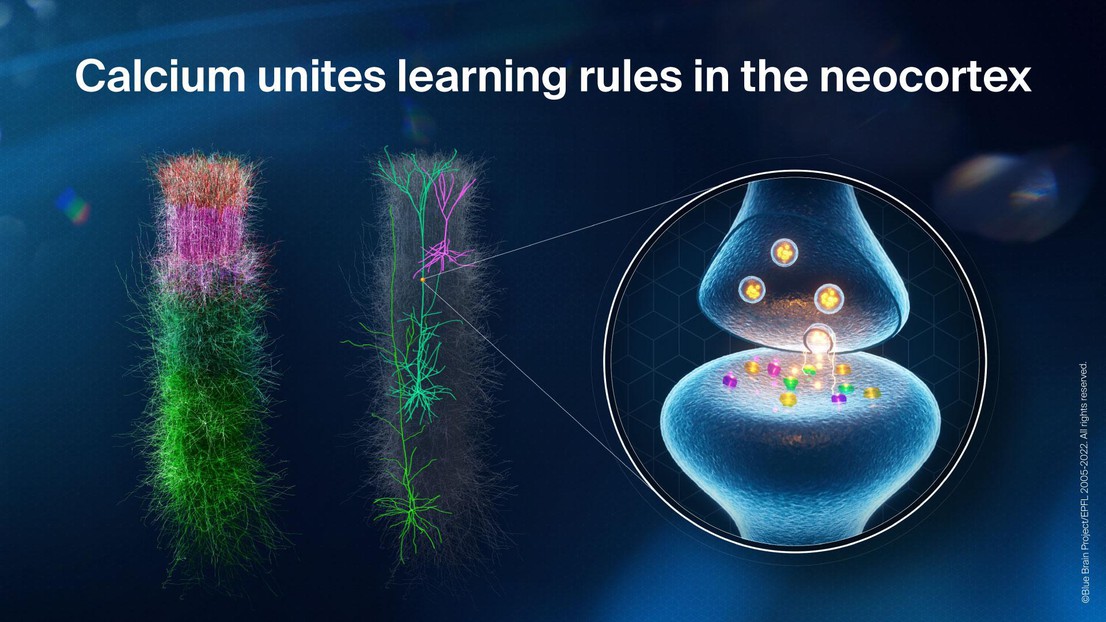
© 2022 EPFL
What underlies learning in the brain might be actually simpler than previously thought despite the brain being one of the most complex objects in the known universe. A collaboration of Scientists led by the EPFL Blue Brain Project has achieved a major advance in accurately simulating the synaptic changes thought to implement learning in the neocortex, opening the door to greater understanding of learning in the brain.
The brain is made up of billions of neurons that communicate with each other by forming trillions of synapses. These connection points between neurons are complex molecular machines that are constantly changing as a result of external stimuli and internal dynamics, a process commonly referred to as ‘synaptic plasticity’. In the neocortex, a key area associated with learning of high-level cognitive functions in mammals, Pyramidal Cells (PCs) account for 80%-90% of the neurons and are known to play a major role in learning. Despite their importance, the long-term dynamics of their synaptic changes have been experimentally characterized between only a few types of PCs, providing little coverage of the complex neural circuits they form, especially across the stereotypical cortical layers, which dictate how the diverse cortical regions interact. How then can we formulate a comprehensive view of the synaptic plasticity dynamics governing learning in neocortical circuits?
In a paper recently published in Nature Communications, a group of scientists from the EPFL Blue Brain Project, together with collaborators from Université de Montréal, Université de Paris, Hebrew University of Jerusalem, Instituto Cajal, and Harvard Medical School, introduce to the neuroscience community a model of synaptic plasticity for PCs in the neocortex based on data-constrained postsynaptic calcium dynamics. By comparing their results to the available experimental data, they show that their synaptic plasticity model can capture the varied plasticity dynamics of the diverse PCs making up the neocortical microcircuit. They achieve this with only one unified model parameter set, indicating the plasticity rules of the neocortex could be shared across pyramidal cell-types.
A complete picture – a full comprehensive model and in vivo prediction
What is unique about this study is that the group focused on finding a solution to the problem of parameterizing a model for the large set of heterogeneous synapses across the different PC connection types in the neocortex, given the sparse experimental constraints available. Moreover, the majority of these plasticity experiments were performed on brain slices in vitro, where the calcium dynamics driving synaptic transmission and plasticity are significantly altered compared to learning in the intact brain in vivo. This study had the particular aim of generalizing the plasticity predictions of the model to physiological calcium dynamics in vivo. Importantly, the study predicts qualitatively different plasticity dynamics from the reference experiments performed in vitro, which, if confirmed, could have profound implications for our understanding of plasticity and learning in the brain.
Lead Author, Giuseppe Chindemi explains, “In this study we designed a model of synaptic plasticity for one specific type of PCs, namely the thick tufted PCs of neocortical layer 5. We found that this model could be transplanted as-is to other PC types to reproduce the results of Long-Term Potentiation (LTP) and Long-Term Depression (LTD) experiments in the same cortical region. Our proposed model and fitting procedure offer a systematic method to predict plasticity outcomes for connections that have yet to be experimentally explored, and a flexible framework for studying cortical learning algorithms in
silico”, he concludes.
This has resulted in the first comprehensive null model for LTP/LTD between neocortical PC types in vivo, and an open source integrated modeling framework opening the door to exploring the input/output conditions relevant for plasticity in vivo, and ultimately the biological algorithms of learning in the neocortex.
Team-science and open-science accelerate progress
“What is exciting about this study”, comments Prof. Henry Markram, Founder and Director of the Blue Brain Project, “is that this is further confirmation for scientists that we can overcome gaps in experimental knowledge using a modelling approach when studying the brain. In addition, the model is open source, and here we have shared hundreds of plastic pyramidal cell connections of different types. Not only is it the most extensively validated plasticity model to date, but it also represents the most comprehensive prediction thus far of the differences between plasticity observed in a petri dish, and in an intact brain. This leap is made possible because of our collaborative team-science approach. Moreover, the community can take it further and develop their own versions by modifying or adding to it – this is open science, and it will accelerate progress”.
“It opens up a world of new directions for scientific inquiry into how we learn,” confirms co-senior author, Prof. Eilif Muller, who co-led the study at the Blue Brain Project prior to his appointment as Assistant Professor at the Université de Montréal, the CHU Ste-Justine Research Center, and the Québec Artificial Intelligence Institute (Mila). “Neurons are shaped like trees, and synapses are the leaves on their branches. Prior approaches to model plasticity have ignored this tree structure, but now we have the computational tools to test the idea that synaptic interactions on branches play a fundamental role in guiding learning in vivo. This has important implications for understanding the mechanisms of neurodevelopmental disorders such as autism and schizophrenia, but also for developing powerful new AI approaches inspired by neuroscience”.
_____________________
Citation - Chindemi, G., Abdellah, M., Amsalem, O., Benavides-Piccione, R., Delattre, V., Doron, M., Ecker, A., Jaquier, A. T., King, J., Kumbhar, P., Monney, C., Perin, R., Rössert, C., Tuncel, A. M., Van Geit, W., DeFelipe, J., Graupner, M., Segev, I., Markram, H., & Muller, E. B. (2022). A calcium-based plasticity model for predicting long-term potentiation and depression in the neocortex. Nature Communications, 13(1), 3038. https://doi.org/10.1038/s41467-022-30214-w
For more information, contact – Blue Brain Communications Manager Kate Mullins – [email protected]
Data and Code Availability
The synapse model, simulations and analysis code used in this work are publicly available on Zenodo at https://doi.org/10.5281/zenodo.5654788
Simulations can be reproduced using EModelRunner https://github.com/BlueBrain/EModelRunner - an open source Python package developed to run cell models provided by the Blue Brain Project portals.
More about the neocortical microcircuit model
In 2015, the Reconstruction and Simulation of Neocortical Microcircuitry was published in Cell, representing the most complete description of any neural microcircuit to date. The model comprises 163,271 compartmental neurons, based on morphological reconstructions of 17 pyramidal cell types across six layers in the rat somatosensory cortex. Extensively validated, it was used here to test the hypothesis that a calcium-based modelling approach can capture the synaptic plasticity behavior and diversity of Pyramidal Cells in the neocortex.
About the EPFL Blue Brain Project
The aim of the EPFL Blue Brain Project, a Swiss brain research initiative founded and directed by Professor Henry Markram, is to establish simulation neuroscience as a complementary approach alongside experimental, theoretical and clinical neuroscience to understanding the brain, by building the world’s first biologically detailed digital reconstructions and simulations of the mouse brain.
About the Authors
Led by the EPFL Blue Brain Project, the scientists involved in this study are affiliated with the following institutions:
Department of Neurobiology and the Edmond and Lily Safra Center for Brain Sciences, the Hebrew University of Jerusalem, Israel
Division of Endocrinology, Diabetes and Metabolism, Beth Israel Deaconess Medical Center, Harvard Medical School, Boston, USA
Instituto Cajal, Consejo Superior de Investigaciones Científicas, Madrid, Spain
Laboratorio Cajal de Circuitos Corticales, Centro de Tecnología Biomédica, Universidad Politécnica de Madrid, Spain
Laboratory of Neural Microcircuitry, École Polytechnique Fédérale de Lausanne, Switzerland
Université de Paris, SPPIN - Saints-Pères Paris Institute for the Neurosciences, CNRS, France
Department of Neurosciences, Faculty of Medicine, Université de Montréal, Canada
CHU Sainte-Justine Research Center, Montréal, Canada
Quebec Artificial Intelligence Institute (Mila), Canada
This study was supported by funding to the Blue Brain Project, a research center of the École polytechnique fédérale de Lausanne, from the Swiss government’s ETH Board of the Swiss Federal Institutes of Technology. E.B.M. received additional support from the CHU Sainte-Justine Research Center (CHUSJRC), the Institute for Data Valorization (IVADO), Fonds de Recherche du Québec - Santé (FRQS), the Canada CIFAR AI Chairs Program, the Quebec Institute for Artificial Intelligence (Mila), and Google. R.B.P. and J.DF. received support from the Spanish “Ministerio de Ciencia e Innovación” (grant PGC2018-094307-B-I00). M.D. and I.S. were supported by a grant from the ETH domain for the Blue Brain Project, the Gatsby Charitable Foundation and the Drahi Family Foundation.