DigitALL: Innovation at EPFL for explainable and equitable AI
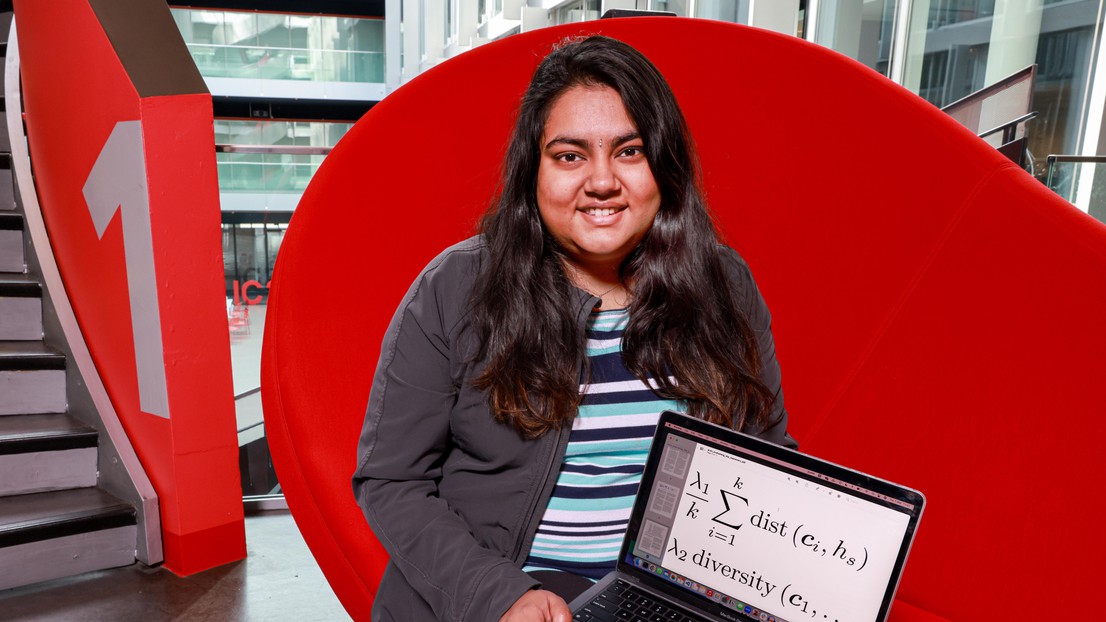
Vinitra Swamy © Alain Herzog / 2023 EPFL
It’s International Women’s Day 2023 and this year the focus is on innovation, technological change and education in the digital age for gender equality. New research from EPFL’s School of Computer and Communication Sciences aims to break gender and other biases.
In 1597 during the Enlightenment, in his work Meditationes Sacrae, the British philosopher Francis Bacon coined the phrase ‘knowledge is power’. Four hundred years on, never has it been more important to have the knowledge and skills to address some of the core challenges faced by humanity today.
Education is key to gaining this knowledge and as we celebrate International Women’s Day, with a spotlight on education in the digital age, how can we ensure educational equality, free from the known bias contained within the machine learning algorithms that drive artificial intelligence?
PhD student Vinitra Swamy, in the Machine Learning for Education Laboratory led by Assistant Professor Tanja Käser, works on neural networks, pervasive as the general form of artificial intelligence models that we see everywhere and behind, for example, ChatGPT.
“One key objective of my research with machine learning is educational equality for all. When you look under the hood at what these models learn, you see human biases reflected from the data, leading to strong inequalities like man is to doctor as woman is to nurse. Mitigating this bias, especially at younger formative stages while students have developing minds and when these kinds of impressions last a long time, is so crucial,” she explained.
Following the explosion in digital education during the COVID-19 pandemic, Swamy is trying to increase course completion rates and address the problems of dropout for students undertaking Mass Online Open Courses (MOOCS), and when using education portals like Moodle, Coursera or EdX.
Specifically, Swamy and her colleagues have trained models to predict student success early in the course to make sure students get the most effective, unbiased, and personalized help they need to improve learning outcomes.
“We look at time series clickstreams of people interacting with education portals to make predictions. The patterns of students pausing or playing a video, when they post a question on the forum, when they answer a question on a quiz – all of these help us predict how to intervene for struggling students to get them back on track,” Swamy said.
“The key problem with using neural networks on human-centric data is that they don't explain their decisions. The models can differentiate whether a picture is a cat or a dog very easily, but when asked to explain why it thinks the picture is a cat or a dog… that’s not inherent to the model design. It’s necessary to add attention layers at the front of the model or use post-hoc explainability methods to work out what models identify as meaningful.”
As the predictions generated by the models, and subsequent interventions, are all about helping students succeed, it’s important to understand why the models predict whether a student will pass or fail – whether the factors are completely arbitrary or not. Swamy used five state-of-the-art explainability models on top of the same neural networks, for the same students, and found that they all disagreed on the explanation for success or failure. This, she says, “is very concerning. If the methods you use to interpret the model are all giving different reasons for predictions of failure, which one can you trust?”
“If we know the model is making a prediction on student success based on demographic or socioeconomic attributes, that's clearly worrying. However if all the explainers are systematically biased in saying what the models find important, that's actually even more scary, because what you're trusting to explain what the model is doing is biased also. Our initial research shows that there is pervasive disagreement in these explainers and our follow-on work aims to build trust around these explainers with human experts in the loop.”
All machine learning models come with sources of underlying bias and there are a lot of stages of the pipeline where this can enter: data selection bias, annotation bias, modeling bias, and downstream human bias. Swamy believes that for computer scientists it is critical to be aware of this issue and as well as how to address it.
“While I was at Microsoft AI, our team saw a lot of clients asking for toolkits to measure bias, choosing to use interpretable models, and understanding that responsible AI was important. In industry and research, we've seen a general uptick in interest for responsibility when it comes to creating these models and measuring and mitigating bias,” Swamy said.
For women and other minorities, Swamy believes machine learning for education can create a level playing field both in terms of educational and gender inequality and minority disparity, so long as attention is paid to all the places that the technology could go wrong.
“The education industry is primed for AI personalization, individualized homework assignments, auto-grading, and the kinds of techniques that we already see happening in the research world. Once those models make their way to the real world with real consequences, this issue of bias becomes a million times more important. It's an ongoing issue.”