DeepBreath: Using deep learning to identify respiratory disease
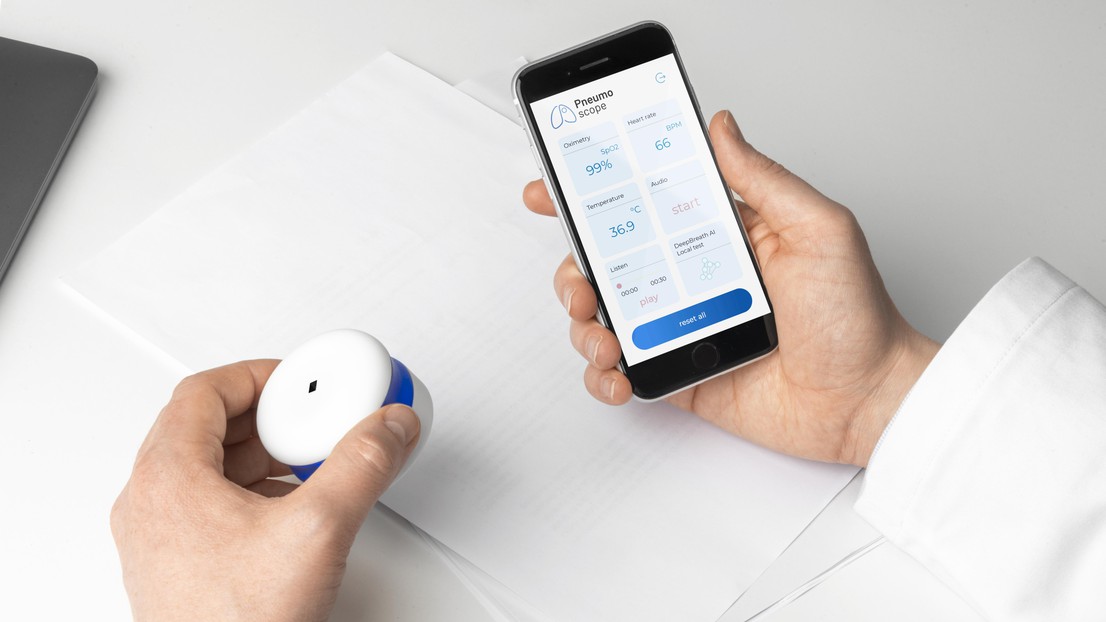
The Pneumoscope © Cyrille Verdon / Renaud Defrancesco BUREAU 141 / EPFL 2023
A new AI algorithm developed at EPFL and University Hospital Geneva (HUG) will power an intelligent stethoscope - Pneumoscope - with the potential to improve the management of respiratory disease in low-resource and remote settings.
As air passes through the labyrinth of small passageways in our lungs, it makes a distinctive whooshing sound. When these passageways are constricted with asthmatic inflammation, or get clogged up with the infectious secretions of bronchitis, the sound changes in characteristic ways. Screening for these diagnostic signatures using a stethoscope applied to the chest, a procedure called auscultation, has become an inescapable element of almost every health check-up.
Yet, despite two centuries of experience with stethoscopes, the interpretation of auscultation is still highly subjective, where one doctor the next will hear something different. Indeed, depending on where you are in the world, a single sound can be variously described as sizzling, popping candy, Velcro, frying rice, and more. The accuracy is further affected by the level of experience of the health worker and their specialization.
These complications make it an ideal challenge for deep learning, which has the potential to discriminate audio patterns more objectively. Deep learning has already been shown to augment human perception in the interpretation of a range of complex medical exams, such as X-rays and MRI scans.
Now, a new study published in Nature Digital Medicine from EPFL’s intelligent Global Health research group (iGH) based in the Machine Learning and Optimization Laboratory, a hub of interdisciplinary AI specialists in the School of Computer and Communication Sciences, described their AI algorithm, DeepBreath, that shows the potential of automated interpretation in the diagnosis of respiratory disease.
“What makes this study particularly unique is the diversity and rigorous collection of the auscultation sound bank,” said the senior author of the study, Dr Mary-Anne Hartley, a medical doctor and biomedical data scientist who heads iGH. Almost six-hundred pediatric outpatients were recruited across five countries - Switzerland, Brazil, Senegal, Cameroon, and Morocco. The breath sounds were recorded on patients under the age of fifteen presenting with the three most common types of respiratory disease - radiographically confirmed pneumonia and clinically diagnosed bronchiolitis, and asthma.
“Respiratory disease is the number one cause of preventable death in this age group,” explained Professor Alain Gervaix, Head of the Department of Pediatric Medicine at HUG, and founder of Onescope: the startup that will bring this intelligent stethoscope that integrates the DeepBreath algorithm to the market. “This work is a perfect example of a successful collaboration between HUG and EPFL, between clinical studies and basic science. The DeepBreath-powered Pneumoscope is a breakthrough innovation for the diagnosis and management of respiratory diseases,” he continued.
Dr Hartley’s team is leading the AI development for Onescope and she is particularly excited by the potential of the tool in low-resource and remote settings. “Reusable, consumable-free diagnostic tools like this intelligent stethoscope have the unique advantage of guaranteed sustainability,” she explained, adding “AI tools also have the potential to continually improve themselves and I am hopeful that we could expand the algorithm to other respiratory diseases and populations with further data.”
DeepBreath is trained on patients from Switzerland and Brazil and then validated on recordings from Senegal, Cameroon, and Morocco, giving insight into the geographic generalizability of the tool. “You can imagine that there are many differences between emergency rooms in Switzerland, Cameroon, and Senegal,” said Dr Hartley and lists examples, “the soundscape of background noise, the way the clinician holds the stethoscope that is recording the sound, the epidemiology, and the local protocols for diagnosis.”
With enough data, an algorithm should be robust to these nuances and find the signal among the noise. DeepBreath maintained an impressive performance across diverse sites despite the small number of patients, which indicates the potential to improve even further with more data.
A particularly unique contribution of the study was the inclusion of methods that aimed to demystify the inner workings of the algorithm’s “black box”. The authors were able to demonstrate that the model was indeed using the breath cycle to make its predictions and show which parts of it were most important. Proving that the algorithm actually uses the breath sounds, instead of “cheating” by using the biased signatures in background noise, is a critical gap in the current literature and can degrade confidence in the algorithm.
The multidisciplinary team is working to prepare the algorithm for real-world use in their intelligent stethoscope, Pneumoscope. A major next task is to repeat the study on more patients using recordings from this newly developed digital stethoscope, which also records temperature and blood oxygenation. “Combining these signals together will likely improve the predictions even further,” predicts Dr. Hartley.
Dr Hartley’s team of students involved in developing DeepBreath includes Julien Heitmann, Jonathan Doenz, Julianne Dervaux, Daniel Hinjos Garcia and Giorgio Mannarini, who all completed their master theses on the project.