Daniel Kuhn winner of the 2024 Farkas Prize
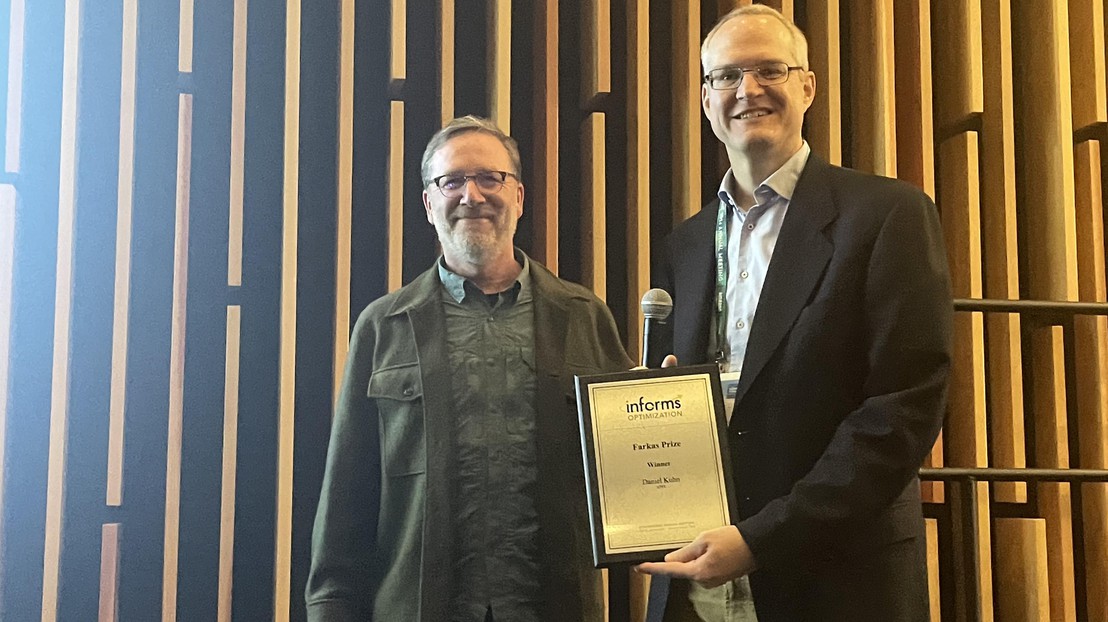
© 2024 EPFL
The Farkas Prize of the INFORMS Optimization Society was established in 2006 and is awarded annually at the INFORMS Fall National Meeting to a mid-career researcher for outstanding contributions to the field of optimization, over the course of their career. The 2024 Farkas Prize was awarded to Daniel Kuhn.
Although it has a long history, the “modern era” of distributionally robust optimization began in the early 2000s, and it has been shaped to a considerable extent by Kuhn’s seminal works on moment-based as well as data-driven ambiguity sets, in particular, Kuhn’s work on “Distributionally Robust Convex Optimization”, which provides a general framework that unifies much of the prior work in the field and characterizes precisely when distributionally robust optimization problems are (not) tractable. The work has become a standard reference in the discipline, and many researchers have extended it to incorporate new types of risk measures, novel characterizations of uncertainty as well as different constraint architectures. In his paper “Data-Driven Distributionally Robust Optimization Using the Wasserstein Metric: Performance Guarantees and Tractable Reformulations” (for which Kuhn received the 2020 INFORMS Frederick W. Lanchester Prize), Kuhn has shown that data-driven optimization problems over Wasserstein balls are efficiently solvable.
Multi-stage robust optimization seeks optimal decisions when information about the uncertain problem parameters is acquired sequentially, and today’s decisions need to account for the fact that future decisions benefit from information that is unavailable today. In his seminal paper “Primal and Dual Linear Decision Rules in Stochastic and Robust Optimization”, Kuhn offers one of the first theoretical analyses of the performance of affine decision rules, a popular approximation scheme going back to the 60s, and thus answers a question first raised by S.
Garstka and R. Wets in 1974, and subsequently reiterated by A. Ben-Tal, L. El Ghaoui and A. Nemirovski in their book on Robust Optimization in 2009. In particular, Kuhn demonstrated that the (typically suboptimal) affine decision rule approximation can be applied not only to the multi-stage robust optimization problem at hand, but also to its dual, thus offering instance-specific optimality bounds that are often remarkably tight. Kuhn’s bounds have subsequently been used by various researchers in domains such as finance, energy, and operations management. Later, Kuhn pioneered similar performance guarantees for robust optimization problems with discrete decisions (where duality-based schemes would fail due to the non-convexity) as well as Markov decision processes with uncertain transition kernels.
Kuhn is the current Editor-in-Chief for Mathematical Programming. He was named an IFORS distinguished lecturer in 2023, and he has delivered many plenary talks at major conferences. Kuhn was elected as an INFORMS Fellow in 2022, and he won the Wilhelm Bessel Research Award in 2021.