Congratulations to Dr. Yves Rychener for obtaining his PhD!
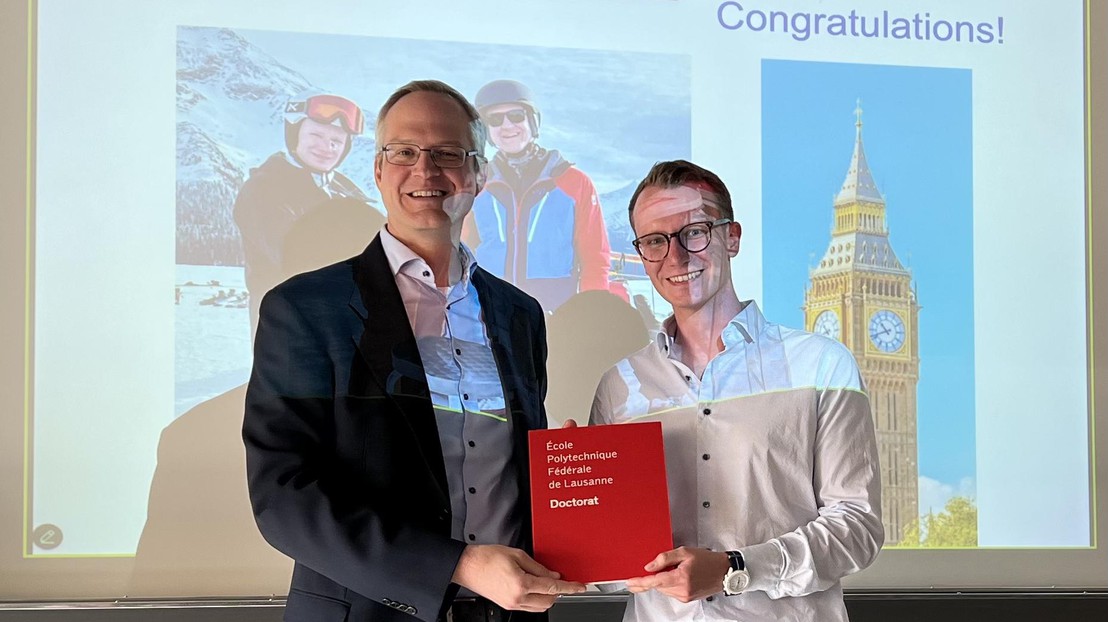
© 2025 EPFL
Dr. Yves Rychener obtained his PhD in April 2025. His dissertation, supervised by Prof. Daniel Kuhn, is entitled "Machine Learning in Decision-Making Systems: Fairness, Robustness, and Data Bias".
Abstract:
Machine learning is increasingly integrated with decision making by powering automated systems and informing human decision makers in applications such as CV screening, portfolio allocation, loan risk assessment, and insurance approval. In this thesis, I explore the challenges that arise when combining machine learning and decision-making, with a particular emphasis on issues like data bias, robustness, fairness, heterogeneous data sources, and decision-aware modeling. In each chapter, I examine these aspects from a different perspective and propose custom algorithms to address them. The first part focuses on decision-aware training, where the prediction model is optimized based on the final decision's outcome. It shows that the minimizer of the end-to-end learning problem corresponds to predictions that lead to the Bayes action. The second part then shifts focus towards addressing issues that arise when the outputs of prediction systems affect human lives and fairness is of interest. The first chapter on fairness investigates group fairness in a centralized setting and proposes a fairness regularization term with corresponding unbiased gradient estimates. This approach simplifies the construction of convergence rates and generalization bounds, offering a scalable method to guarantee fairness in real-world applications where group fairness is critical. Another issue with data related to humans is that they often cannot be transferred to a central server due to privacy considerations.
The second chapter on fairness extends the fair learning approach from the preceding chapter to the federated learning setting, where different clients hold different parts of the dataset. Based on a function-tracking scheme, it proposes a group-fairness regularizer on the global level, which can be combined with most existing federated learning algorithms and requires limited computational and communication overhead. The final part then focuses on decision-making. It proposes an approach for combining information from different (biased) reference distributions to make decisions on a target domain of interest. By intersecting optimal transport-balls around the different reference distributions, the decision maker can optimize over the worst-case distribution which is consistent with all reference distributions.