Congratulations to Dr. Bahar Taşkesen for obtaining her PhD!
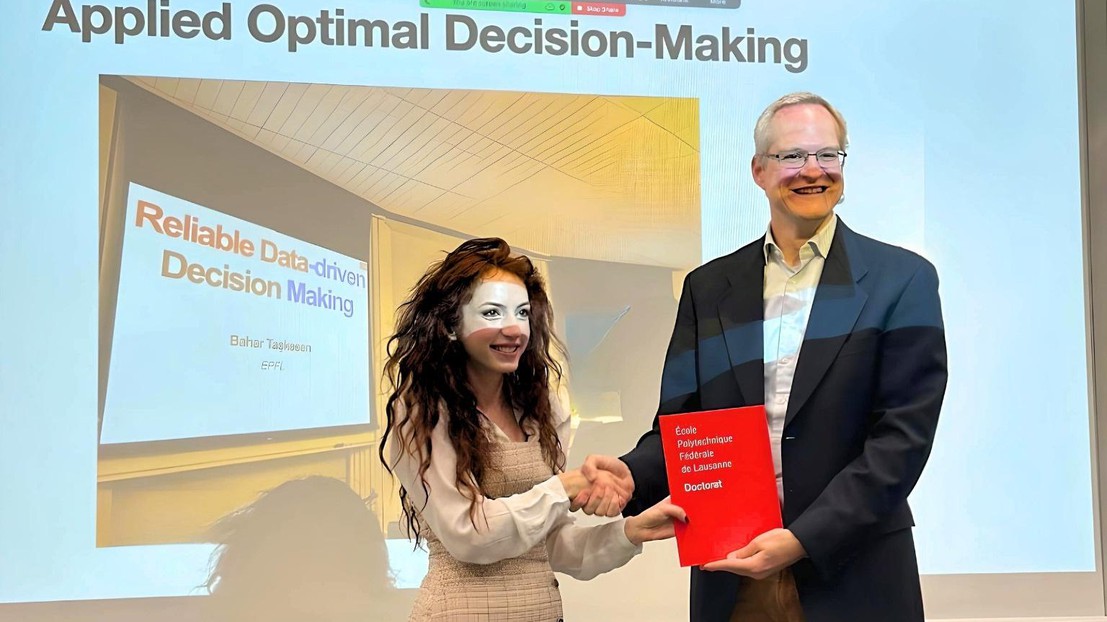
Bahar Taşkesen & Daniel Kuhn © 2024 EPFL
Dr. Bahar Taşkesen obtained her PhD in June 2024. Her dissertation, supervised by Prof. Daniel Kuhn, is entitled "Reliable data-driven decision-making through optimal transport".
Abstract:
Decision-making permeates every aspect of human and societal development, from individuals' daily choices to the complex decisions made by communities and institutions. Central to effective decision-making is the discipline of optimization, which seeks the best choice from a set of alternatives based on specific criteria. This thesis focuses on optimization problems fueled by the ever-growing abundance of data. In an era where data is ubiquitous, machine learning algorithms offer unprecedented potential to enhance decision-making across diverse sectors such as healthcare, finance, and technology. The enthusiastic adoption of machine learning in various sectors has necessitated a more cautious approach upon realizing that the reliability of these systems in complex real-world situations is not always guaranteed. At the heart of this investigation is the ambition to design algorithms equipped to make reliable data-driven decisions. This entails addressing the challenges of ensuring robust performance outside training environments, incorporating fairness measures when needed, and achieving decision interpretability while maintaining computational efficiency. Attempting to satisfy all these desires simultaneously is a formidable task, given the challenges in the data collection phase and modeling. In its most comprehensive form, our objective in this thesis entails modeling, developing tools for, and auditing data-driven decision-making systems based on data generated by an unknown mechanism.
The common theme shared within the lines of works in this thesis is the use of optimal transport. Thus, the first part of this thesis introduces the optimal transport problem, studies its computational complexity, and proposes numerical solutions. The rest of the thesis explores two interrelated learning paradigms: static decision-making, in which decisions have no immediate impact on the data used in training, and dynamic decision-making, in which decisions actively influence the data acquisition process. The third chapter then investigates the development of estimators in scenarios marked by data scarcity in the target domain despite abundant data in a related source domain. Utilizing optimal transport, we propose robust estimators that capitalize on source data while accommodating the sparse target data. In the fourth chapter, we focus on creating fair and robust models. We introduce a distributionally robust logistic regression model with an unfairness penalty, which helps to prevent discrimination based on sensitive attributes such as gender or ethnicity. This model is tractable when an optimal transport-based ambiguity set is utilized. While it is important to train fair models, it is equally crucial to rigorously examine machine learning models before deploying them in practice. In the fifth chapter, we use ideas from the optimal transport theory and propose a statistical test for detecting unfair classifiers. The sixth chapter extends linear quadratic Gaussian control problems to their distributionally robust counterparts using an optimal transport-based ambiguity set, offering structural insights that aid in the efficient design of numerical solutions.