Congratulation to Dr. Sohey Massoudi
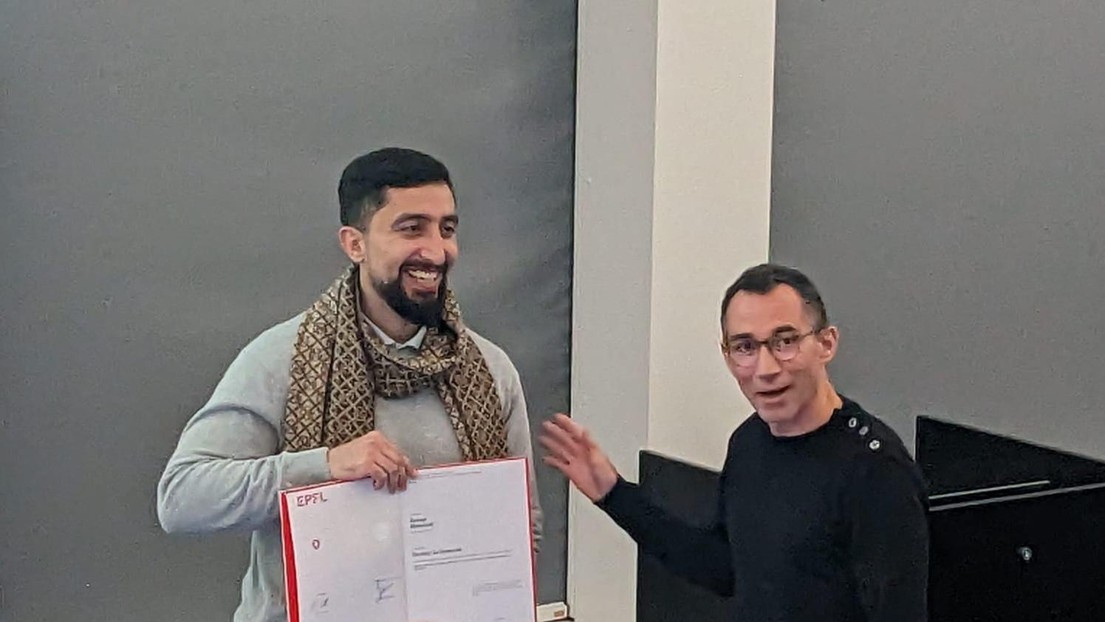
© 2024 LAMD EPFL
On November 15th, Dr. Soheyl Massoudi successfully defended his PhD thesis entitled "Empowering turbocompressor design with AI: a unified approach to robustness and all-at-once optimization" at the Neuchatel associated campus of EPFL.
During his Ph.D. at EPFL's Laboratory for Applied Mechanical Design (LAMD), Soheyl Massoudi developed a groundbreaking approach to designing gas-bearing supported turbocompressors, which are essential for technologies like heat pumps and fuel cells. These high-speed machines, spinning at hundreds of thousands of RPM, are highly sensitive to minor imperfections that can lead to efficiency losses or system failure. Traditional methods typically optimize each component—such as bearings, rotor, and impeller—independently and under ideal conditions, often neglecting real-world manufacturing deviations. To address this, Soheyl introduced robustness metrics as key objectives and constraints within his optimization framework, ensuring the resulting designs are resilient against manufacturing variations. Furthermore, his all-at-once optimization approach considers the coupling between subsystems, resulting in a system that is not only globally efficient and fully functional but also inherently more robust.
To achieve this, Soheyl harnessed advanced artificial intelligence techniques, using ensemble artificial neural networks and genetic algorithms to model the entire system efficiently. These AI models, trained on high-fidelity simulation data, enable rapid design predictions without extensive computational resources. Additionally, Soheyl’s unique approach of training these models on dimensionless groups improved their accuracy, allowing them to generalize effectively across different designs and power ranges.
One of Soheyl’s major contributions is the creation of three key tools for turbocompressor design: ARMID (Automated Robust Modular Integrated Design), ParaturboCAD for 3D model generation, and DARTS-NETGAB for real-time simulation and parameter adjustments. These tools streamline the design process, making it possible to explore a wide range of designs quickly and accurately. Experimental testing on a custom-built rig validated these AI-generated designs, confirming their performance in real-world conditions.
Beyond his research, Soheyl contributed to teaching, mentored multiple student projects, and supported the lab’s IT infrastructure, enhancing LAMD’s educational and technical resources. His work, presented at major conferences and published in top journals, offers a robust, AI-driven framework that improves the reliability and efficiency of turbocompressors, with potential applications in various industries.