Computational Quantum Science Lab at the APS Global Physics Summit
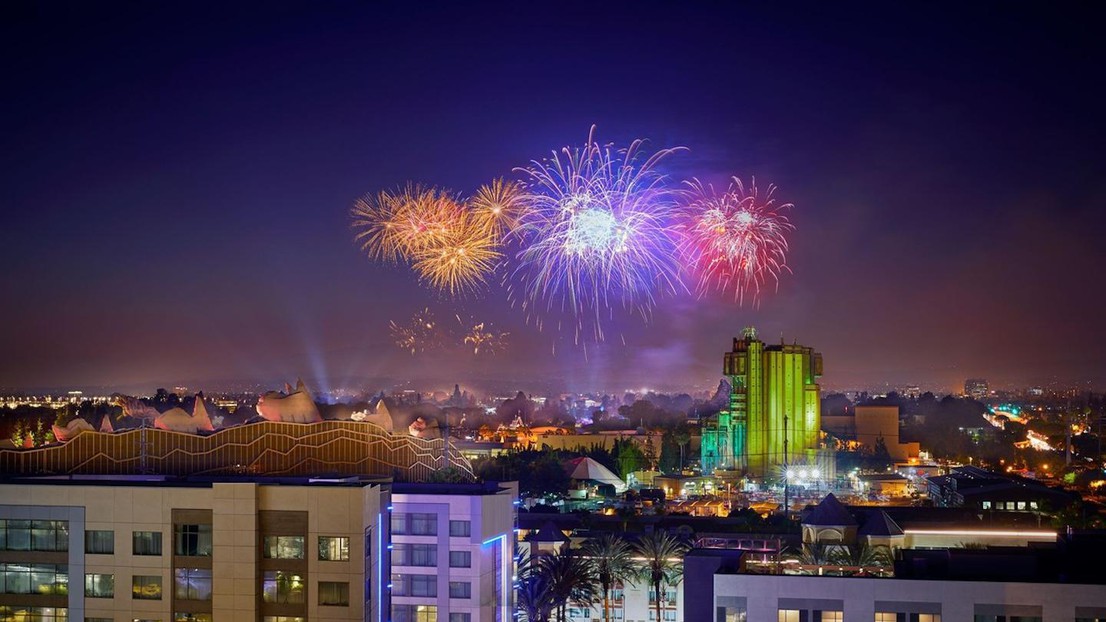
© 2025 EPFL
At this year's APS Global Physics Summit in Anaheim, the Computational Quantum Science Lab showcased several contributions, spanning quantum dynamics, neural-network methodologies, topological quantum systems, and quantum chemistry. Eight among lab members and collaborators delivered presentations reflecting both theoretical ingenuity and practical advancements, offering insights into the lab’s innovative approach at the intersection of quantum computing and machine learning.
Presented below are this year's contributions, highlighting innovative results and novel approaches by our lab researchers.
• Correcting and Extending Trotterized Quantum Dynamics with a Quantum-Classical Hybrid Ansatz
Introducing a quantum-classical hybrid method to refine Trotterized quantum dynamics, reducing computational errors and broadening simulation capabilities.
(Presenter: Gian Gentinetta)
• Neural Network Representations of Fermionic Ground and Excited States
Demonstrating the effectiveness of neural-network architectures in accurately capturing complex fermionic states, with important implications for strongly correlated electronic systems.
(Presenter: Jane M Kim)
• Non-Stabilizerness with Neural Quantum States
Proposing a novel neural-network-based framework to quantify non-stabilizer quantum states, providing critical insights into resource assessment for quantum computations.
(Presenter: Alessandro Sinibaldi)
• A Single Wave Function for Multiple Systems: Neural-Network Quantum States as Foundation Models
Presenting a unified neural-network quantum-state architecture that enables efficient modeling across diverse quantum systems, paving the way for versatile simulation protocols.
(Presenter: Luciano L Viteritti)
• Improved Second Quantized Neural Quantum States for Molecules
Advancing neural-network quantum states in second quantization to significantly enhance computational precision in molecular simulations and chemical property predictions.
(Presenter: Clemens Giuliani)
• Grassmannian Variational Monte Carlo for Simultaneous Multiple State Optimization
Introducing a new Grassmannian geometry-inspired variational Monte Carlo approach that efficiently optimizes multiple quantum states simultaneously, ensuring increased computational robustness.
(Presenter: Douglas G Hendry)
• Predicting Topological Entanglement Entropy in a Rydberg Analog Simulator
Establishing a neural-network-based methodology to predict topological entanglement entropy, enriching our understanding of quantum correlations in Rydberg atom simulators.
(Presenter: Linda Mauron)
• Phase Diagram of Helium-4 in Two Dimensions Using Neural Quantum States
Employing advanced neural quantum-state methods to accurately chart quantum phase transitions in two-dimensional helium-4, offering new insights into this fundamental quantum system.
(Presenter: David Linteau)
The wide-ranging topics covered in these presentations underscore the lab’s continued dedication to pushing the boundaries of computational quantum science, fostering innovative techniques, and addressing some of the most pressing challenges in quantum physics today.