Chen Liu's paper accepted to NeurIPS 2020
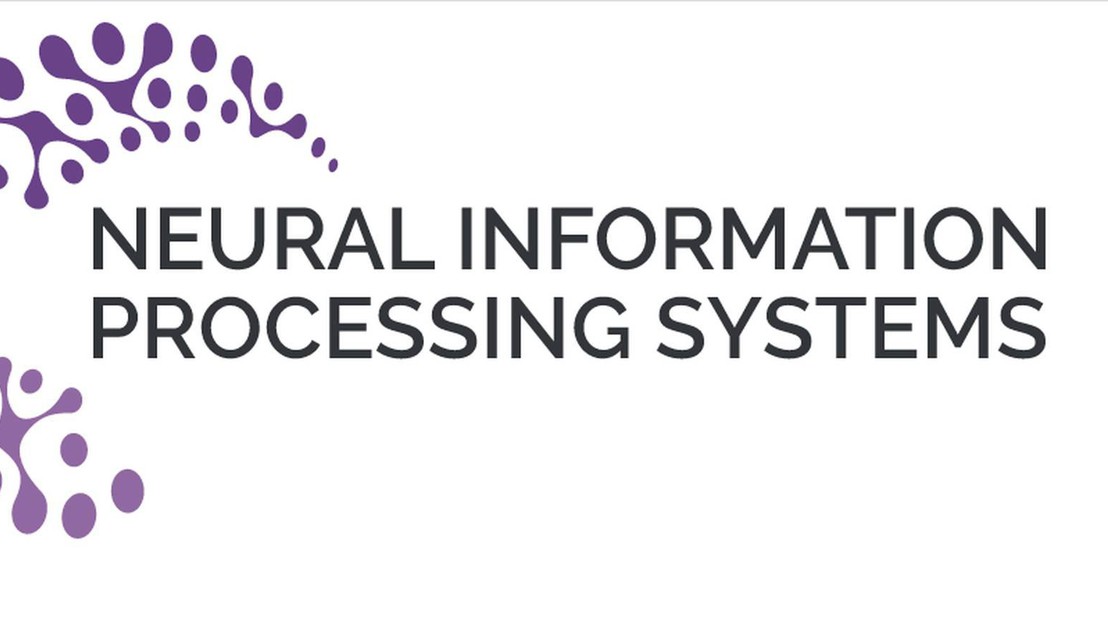
© 2020 Neurips
The paper “On the Loss Landscape of Adversarial Training: Identifying Challenges and How to Overcome Them” is accepted to NeurIPS 2020. IVRL member Chen Liu will present this work at the 34th Conference on Neural Information Processing Systems, which will be held online between 6th and 12th December 2020.
Authors:
Chen Liu, Mathieu Salzmann, Tao Lin, Ryota Tomioka, Sabine Süsstrunk
Abstract:
We analyze the influence of adversarial training on the loss landscape of machine learning models. To this end, we first provide analytical studies of the properties of adversarial loss functions under different adversarial budgets. We then demonstrate that the adversarial loss landscape is less favorable to optimization, due to increased curvature and more scattered gradients. Our conclusions are validated by numerical analyses, which show that training under large adversarial budgets impede the escape from suboptimal random initialization, cause non-vanishing gradients and make the model find sharper minima. Based on these observations, we show that a periodic adversarial scheduling (PAS) strategy can effectively overcome these challenges, yielding better results than vanilla adversarial training while being much less sensitive to the choice of learning rate.
Arxiv link of the paper:
https://arxiv.org/abs/2006.08403
Github page of the work: