Chemical data management: an open way forward
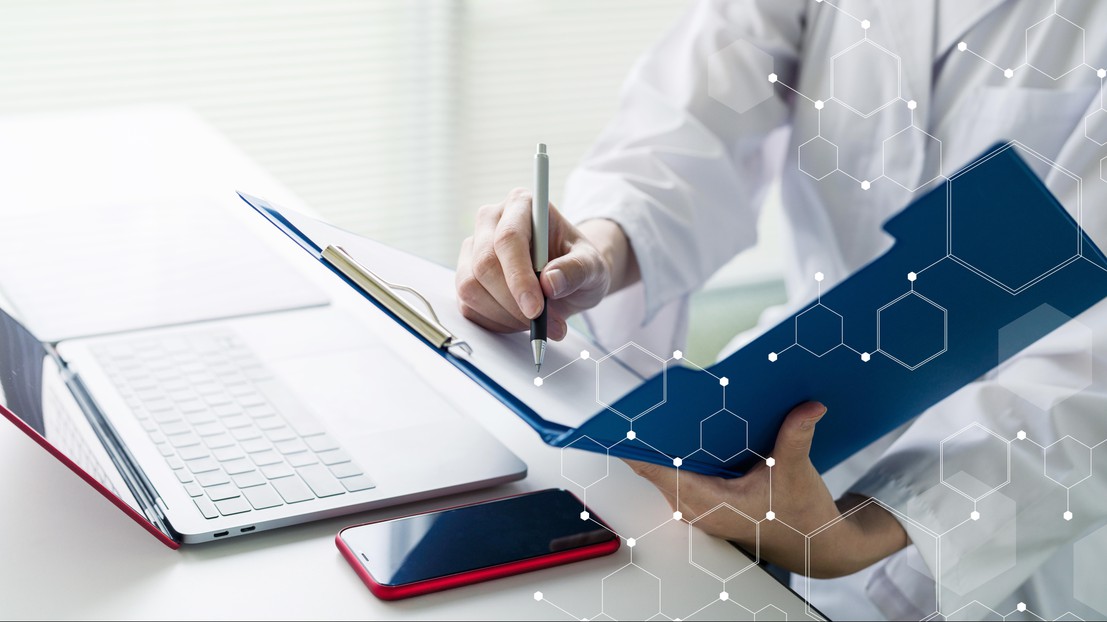
iStock
Writing in Nature Chemistry, three EPFL scientists propose an open platform for managing the vast amounts of diverse data produced in chemical research. Based on principles of accessibility, collaboration and efficiency, the proposed platform could be spearheaded by EPFL.
One of the most challenging aspects of modern chemistry is managing data. For example, when synthesizing a new compound, scientists will go through multiple attempts of trial-and-error to find the right conditions for the reaction, generating in the process massive amounts of raw data. Such data is of incredible value, as, like humans, machine-learning algorithms can learn much from failed and partially successful experiments.
The current practice is, however, to publish only the most successful experiments, since no human can meaningfully process the massive amounts of failed ones. But AI has changed this; it is exactly what these machine-learning methods can do provided the data are stored in a machine-actionable format for anyone to use.
“For a long time, we needed to compress information due to the limited page count in printed journal articles,” says Professor Berend Smit, who directs the Laboratory of Molecular Simulation at EPFL Valais Wallis. “Nowadays, many journals do not even have printed editions anymore; however, chemists still struggle with reproducibility problems because journal articles are missing crucial details. Researchers ‘waste’ time and resources replicating ‘failed’ experiments of authors and struggle to build on top of published results as raw data are rarely published.”
But volume is not the only problem here; data diversity is another: research groups use different tools like Electronic Lab Notebook software, which store data in proprietary formats that are sometimes incompatible with each other. This lack of standardization makes it nearly impossible for groups to share data.
Now, Smit, with Luc Patiny and Kevin Jablonka at EPFL, have published a perspective in Nature Chemistry presenting an open platform for the entire chemistry workflow: from the inception of a project to its publication.
The scientists envision the platform as “seamlessly” integrating three crucial steps: data collection, data processing, and data publication – all with minimal cost to researchers. The guiding principle is that data should be FAIR: easily findable, accessible, interoperable, and re-usable. “At the moment of data collection, the data will be automatically converted into a standard FAIR format, making it possible to automatically publish all ‘failed’ and partially successful experiments together with the most successful experiment,” says Smit.
But the authors go a step further, proposing that data should also be machine-actionable. “We are seeing more and more data-science studies in chemistry,” says Jablonka. “Indeed, recent results in machine learning try to tackle some of the problems chemists believe are unsolvable. For instance, our group has made enormous progress in predicting optimal reaction conditions using machine-learning models. But those models would be much more valuable if they could also learn reaction conditions that fail, but otherwise, they remain biased because only the successful conditions are published.”
Finally, the authors propose five concrete steps that the field must take to create a FAIR data-management plan:
- The chemistry community should embrace its own existing standards and solutions.
- Journals need to make deposition of reusable raw data, where community standards exist, mandatory.
- We need to embrace the publication of “failed” experiments.
- Electronic Lab Notebooks that do not allow exporting all data into an open machine-actionable form should be avoided.
- Data-intensive research must enter our curricula.
“We think there is no need to invent new file formats or technologies,” says Patiny. “In principle, all the technology is there, and we need to embrace existing technologies and make them interoperable.”
The authors also point out that just storing data in any electronic lab notebook – the current trend – does not necessarily mean that humans and machines can reuse the data. Rather, the data must be structured and published in a standardized format, and they also must contain enough context to enable data-driven actions.
“Our perspective offers a vision of what we think are the key components to bridge the gap between data and machine learning for core problems in chemistry,” says Smit. “We also provide an open science solution in which EPFL can take the lead.”
European Union’s Horizon 2020
Swiss National Science Foundation (SNSF) – NCCR MARVEL
Kevin Maik Jablonka, Luc Patiny, Berend Smit. Making the collective knowledge of chemistry open and machine-actionable. Nature Chemistry 04 April 2022. DOI: 10.1038/s41557-022-00910-7