Brain models draw closer to real-life neurons
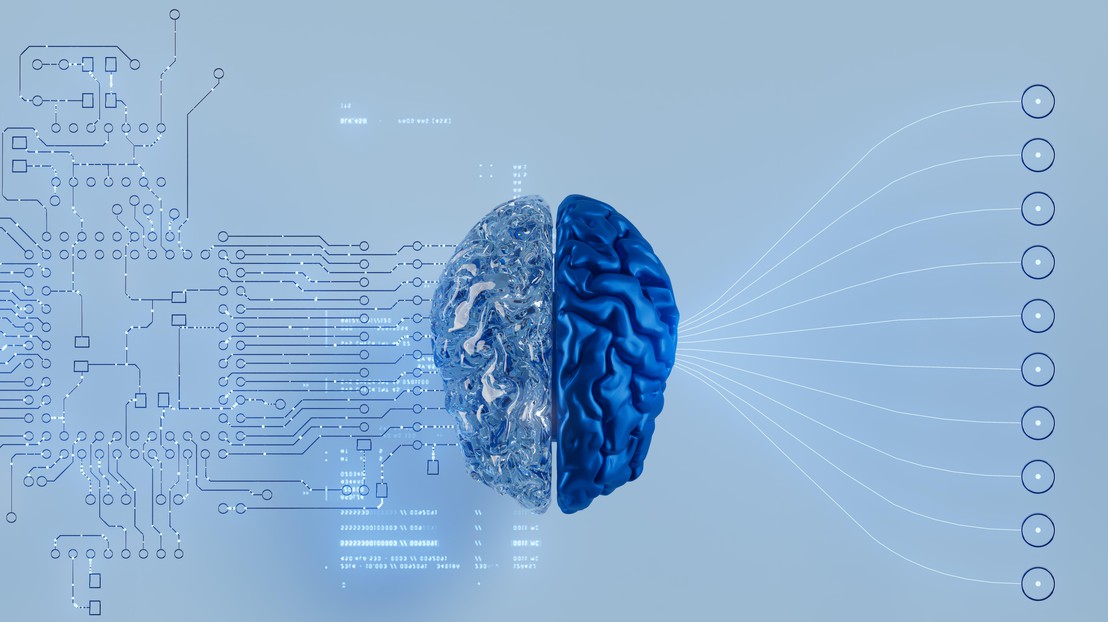
© EPFL/iStock (Just_Super)
Researchers at EPFL have shown how rough, biological spiking neural networks can mimic the behavior of brain models called recurrent neural networks. The findings challenge traditional assumptions and open new doors in computational neuroscience and even AI.
Every thought, memory, or action starts with a burst of electricity in your brain: neurons firing signals to each other. Neuroscientists often simplify this complex activity into mathematical models to study how the brain works.
The problem is that these models are much smoother than the noisy, unpredictable signals of real neurons. So, for decades, neuroscience has grappled with a key question: How do biological networks, governed by noisy, spike-based signals, produce robust and coherent dynamics?
Despite popular ideas, brains don’t actually work like computers. Instead of steady streams of information, they rely on "spikes"—quick bursts of electrical activity. Modeling this spiking activity is incredibly challenging because it looks random, noisy, and doesn’t follow clean patterns.
To make it easier, scientists have relied on simplified models called recurrent neural networks (RNNs). These models use continuous signals to mimic how neurons communicate, and they’re great for running simulations or powering artificial intelligence.
But there’s a catch: RNNs don’t look or behave much like real neurons, which makes them less useful for understanding how the brain itself works. Past attempts to align spiking neural networks (SNNs), which are closer to real neurons, with RNNs relied on shortcuts: Scientists had to either duplicate neurons to smooth out noise or make unrealistic assumptions, e.g. that neurons fire electrical signals non-stop. These workarounds produce some useful results, but they weren’t biologically realistic.
Biology matches theory
Three researchers at EPFL, Valentin Schmutz, Johanni Brea, and Wulfram Gerstner, have now shown that large populations of spiking neurons can naturally mimic the smooth behavior of RNNs — and they don’t need duplicates or unrealistic assumptions to do so.
Instead, their study shows that as networks of spiking neurons grow larger and more diverse, their activity stabilizes on its own. This means that even though individual neurons are noisy and unpredictable, their combined activity creates stable patterns that look like the smooth dynamics of RNNs.
The researchers started by building a network of SNNs with random connections—no two neurons received the exact same inputs, a feature called “duplicate-free.” Using a mix of mathematical proofs and computer simulations, they showed how the network stabilizes despite this randomness and diversity.
They tapped into a phenomenon called the "concentration of measure," which explains how, in large systems, random components can average out to create order. Imagine throwing a hundred coins in the air: each coin is random, but the overall result will hover close to half heads, half tails. Similarly, in these large spiking networks, the randomness of individual neurons balances out to create predictable, smooth activity.
The study revealed two important things. First, that large networks of spiking neurons don’t need duplicates or artificial smoothing to act like RNNs. Their natural dynamics are enough to create stable, rate-like patterns.
Second, the bigger the network, the closer it gets to behaving like an RNN. The researchers even calculated how fast this convergence happens, using mathematical models that matched their simulations.
This means that SNNs—closer to how the brain actually works—can match the performance of RNNs, which are widely used in AI and computational neuroscience.
The discovery could change how we think about neural networks in both biology and AI, by helping us design more energy-efficient systems that work like the brain, using spikes instead of continuous signals.
It also opens up a better way for neuroscientists to model real brain activity without relying on artificial assumptions, and sheds light on one of the field’s big debates: do brains compute using spikes, rates, or both? The study suggests that spikes might be enough to produce the smooth dynamics we see in brain activity.
Other contributors
University College London
Swiss National Science Foundation (SNSF)
Royal Society Newton International Fellowship
Valentin Schmutz, Johanni Brea, Wulfram Gerstner. Emergent Rate-Based Dynamics in Duplicate-Free Populations of Spiking Neurons. Physical Review Letters, Vol. 134, 018401 (06 January 2025). DOI: 10.1103/PhysRevLett.134.018401