Blue Brain uses deep learning to align complex brain datasets
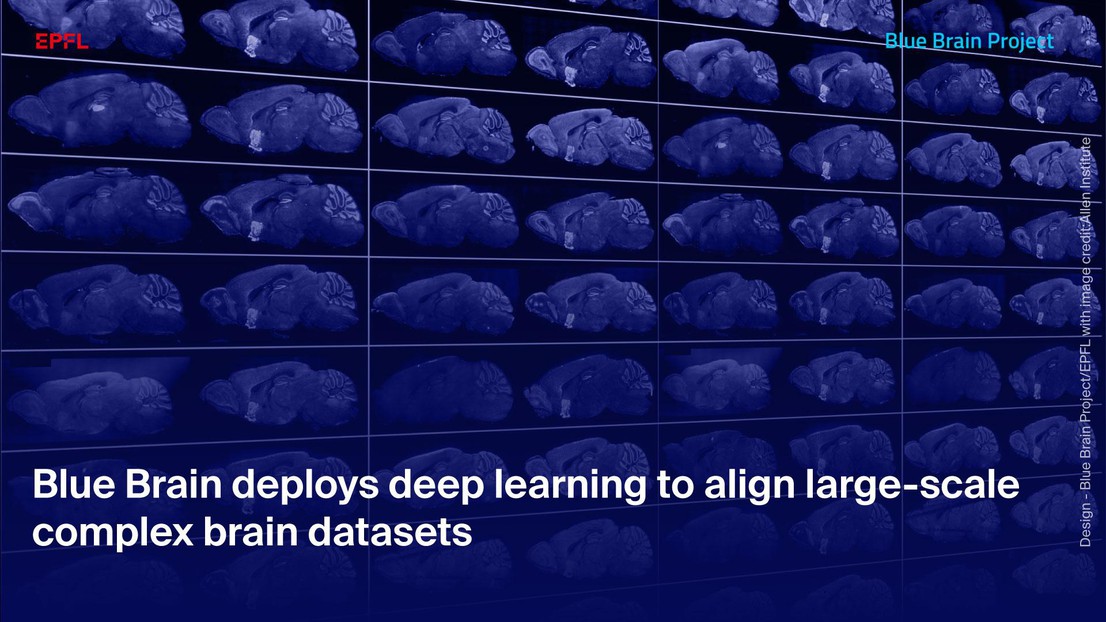
© 2021 EPFL
Atlases of the brain are an essential tool for neuroscientific research as they make it possible to see diverse and multimodal data in the same reference frame. This is particularly the case for the EPFL Blue Brain Project where our work requires the acquisition and integration of high quality maps of data of the rodent brain. As those data sets are acquired from different animals, the integration thereof requires a registration step, i.e. bringing individual datasets in spatial overlap.
Blue Brain’s Machine Learning team has developed dedicated open source tools to enable both our and other scientists to align large-scale complex brain datasets, specifically spatial gene expression data of the rodent brain with a reference atlas. While computer-aided alignment software exists, accurate alignments typically require numerous manual corrections by the human expert - a process that is very time-consuming, non-reproducible, and cannot scale to the rapidly growing data of spatial gene expression.
The approach followed by Blue Brain uses machine learning to automate this registration process and doing away with the need for corrections by the human expert. It was tested on data from the Allen Mouse Brain Atlas from the Allen Institute for Brain Science, hosting a rich database of gene expression images, Nissl volumes, and reference atlases. Our results show an acceleration and improvement in accuracy over previous methods.
A new deep learning framework for multimodal gene registration
Given the fundamental importance of acquiring and integrating high quality maps of gene expression in the rodent brain, the Blue Brain Project has developed a deep learning framework for aligning multimodal gene expressions to a reference volume. The complex task of predicting a transformation that maps a moving image to a target reference is known as ‘image registration’, but in our case this is made even more challenging by the multimodality of gene expressions with respect to reference Nissl stains and by several artifacts like air bubbles and tears in the brain tissue samples.
Therefore, the Blue Brain Machine Learning team has developed dedicated tools to enable both our and other scientists to better leverage the Allen Institute’s data and use them for their own projects.
Atlas Alignment is a Blue Brain framework to perform multimodal image registration onto a reference volume. It includes both traditional and supervised deep learning models and enables the user to tailor their data. This tool efficiently leverages labels provided by a trained human expert to accurately register multimodal 2D coronal section images showing gene expression stains.
We also developed Atlas Download Tools to enable users to search, download, and prepare atlas data prior to using Atlas Alignment. This can be downloading single section images and/or entire datasets, as well as the corresponding metadata. It can also further pre-process the image data to place it in the standard reference space.
Everyone working with brain atlas data can use and benefit from these open source and easy to install tools. Atlas Alignment can also be used to fix any misalignment between ISH stains and Nissl stains within the Blue Brain Cell Atlas.
We tested our tools on the Allen Mouse Brain Atlas, comprising whole brain Nissl and gene expression stains, and our results showed that our framework and design of the loss function result in highly accurate and smooth predictions. Our model proved to be able to generalize to unseen gene expressions and coronal sections, outperforming traditional intensity-based approaches in aligning complex brain structures.
We demonstrated with two different experiments that our proposed approach produces accurate predictions that generalize well to unseen gene expressions and coronal sections. This is particularly significant given the high variability of shape and appearance across stains and sections. We benchmarked our results against the state-of-the art method SyN, and our results showed that our model is significantly faster and it achieves higher accuracy in most cases.
From a qualitative point of view, our analysis shows that our model is able to predict deformation fields that are very close to the ground truth annotations provided by a human expert, even in the case of slices affected by artifacts such as air bubbles and tears. Indeed, during the training phase, our model is presented with samples including various kinds of anomalies, and therefore it learns how to predict a deformation field in a correct way, as opposed to intensity-based approaches.
“Being able to do the registration of different gene expression data sets automatically with an accuracy comparable to a human expert is a big win. We can now readily ingest the ground truth data from our colleagues at the Allen Institute at the best possible accuracy,” explains Blue Brain Computing Director, Prof. Felix Schürmann. “And since we made it open source, others can use and build on these tools for open science.”
“Looking ahead for the Blue Brain,” discloses Francesco Casalegno, Leader of Blue Brain’s Machine Learning team, “We will be applying our approach to new datasets. One specific example is to investigate the alignment of volumes to a reference volume consisting of detailed brain region annotations, instead of Nissl stains.”
------------------------
For more information, please contact Blue Brain Communications Manager – Kate Mullins
Read the paper - Krepl, J., Casalegno, F., Delattre, E., Erö, C., Lu, H., Keller, D., Rodarie, D., Markram, H., & Schürmann, F. (2021). Supervised learning with perceptual similarity for multimodal gene expression registration of a mouse brain atlas. Frontiers in Neuroinformatics, 15, 37. https://doi.org/10.3389/fninf.2021.691918
For the complete suite of Deep Atlas tools created by the Blue Brain Project and to see what is coming soon, go to - https://github.com/BlueBrain/Deep-Atlas
This study was supported by funding to the Blue Brain Project, a research center of the Ecole polytechnique fédérale de Lausanne (EPFL), from the Swiss government’s ETH Board of the Swiss Federal Institutes of Technology.