Alexis Goujon wins EEDE Thesis Award
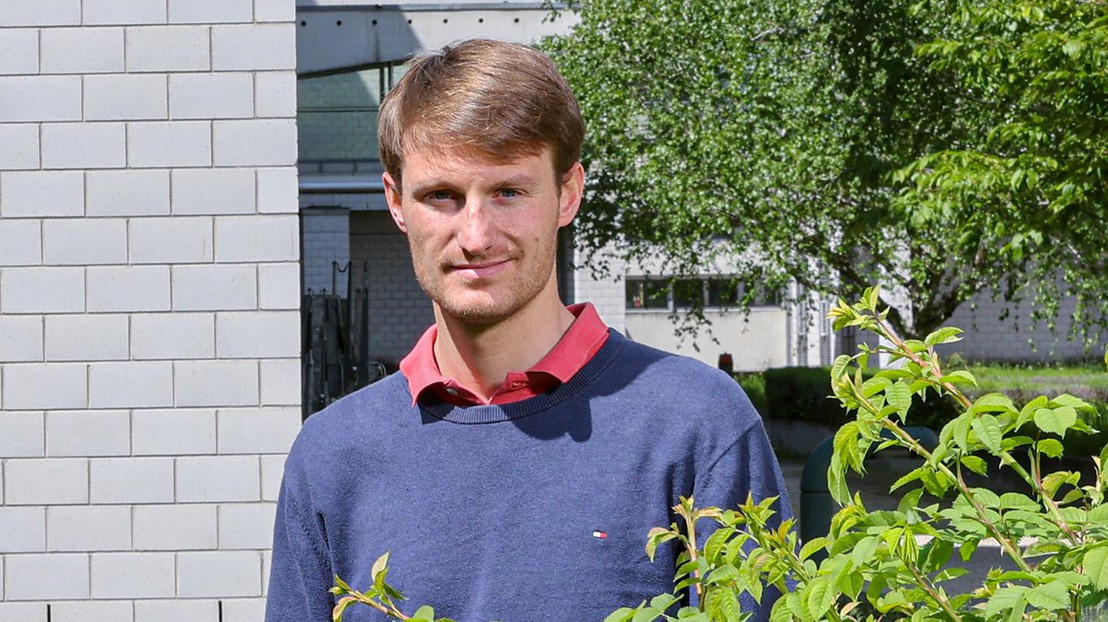
Alexis Goujon © 2022 Alain Herzog
Alexis Goujon has received the Electrical Engineering Doctoral program (EDEE) Thesis Award for his outstanding research on improving the trustworthiness of deep learning methods for medical image reconstruction while maintaining performance. Mingxiang Gao, Francesco Gerini, Lakmal Buddika Meegahapola, and Veronica Lily Ravano also received EDEE Thesis Distinctions.
Alexis Goujon's prize-winning thesis, entitled "Towards Trustworthy Deep Learning for Image Reconstruction", was completed under the supervision of Michaël Unser of the Biomedical Imaging Group in the School of Engineering. The work focuses on the trustworthiness of the output of deep learning models. For example, unexpected behaviors are sometimes encountered in sensitive applications, such as hallucinations in medical image reconstruction. Goujon's thesis addresses these concerns via the design of expressive, stable, and interpretable spline-based parameterizations across various contexts.
The annual EDEE Thesis Award honors an “outstanding and remarkable” PhD thesis work in the field of electrical engineering from an EDEE student. Each year, EDEE Thesis Distinctions are also granted to a selection of very high quality theses, in order to highlight the doctoral candidates’ research work and their scientific merit. For each doctoral program, nominated graduates are selected on the basis of their oral examination. Then the program committee evaluates the nominees and rewards the best 8%. The EDEE Distinction 8% for 2024 has been awarded to Mingxiang Gao, Francesco Gerini, Lakmal Buddika Meegahapola, and Veronica Lily Ravano.