A new tool for tracing the family trees of cells
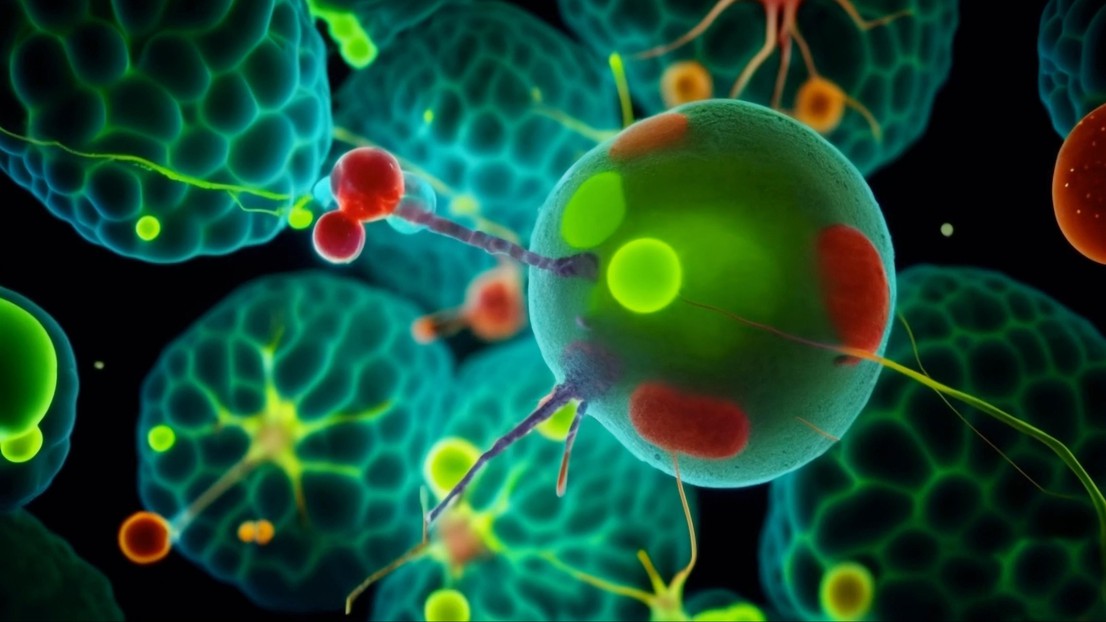
© EPFL/iStock (photobank kiev)
EPFL researchers have developed GEMLI, a pioneering tool that could democratize and vastly improve how we study the journey of cells from their embryonic state through to specialized roles in the body, as well as their changes in cancer and other diseases.
In the intricate dance of life, where cells multiply and diversify to form the different parts of organisms, understanding each cell's origin can be crucial. This is what biologists refer to as “cell lineage” – a family tree, but for cells. Just as you can trace your ancestry back to your grandparents and beyond, scientists can trace how cells divide and evolve from a single "parent" cell into various "offspring" cells, each with its own role in the body.
Tracing cell lineages helps us understand how complex organisms, like humans, can develop from a single fertilized egg into beings with trillions of specialized cells, and how disruptions in this process can lead to diseases like cancer. However, the field has faced some significant hurdles, mostly because lineage- tracing requires complex and labor-intensive techniques.
Introducing GEMLI
Now, scientists led by Almut Eisele and David Suter at EPFL, have developed a computational tool that can work out the lineage relationships between cells without the need for specialized experimental lineage-tracing methods.
The tool, Gene Expression Memory-based Lineage Inference (GEMLI), requires only single-cell RNA sequencing (scRNA-seq) data, a widely used technique that captures “snapshots” of the genes that are being expressed by an individual cell at any given time.
GEMLI capitalizes on the fascinating phenomenon of gene expression memory. Just like you might remember a recipe after making it several times, some genes maintain the intensity at which they are expressed over several cell generations. So by leveraging these "memory genes" in scRNA-seq datasets, GEMLI can piece together the lineage relationships between different cells, effectively reconstructing their family tree based solely on gene expression patterns.
The scientists rigorously tested GEMLI across various cell types and conditions, including embryonic stem cells, fibroblasts, blood cells, intestinal cells, and various cancer cell types, both in vitro and in vivo. In all the tests, GEMLI proved to be both robust and versatile.
Cell lineage identification by GEMLI, by small group of cells (left) or larger lineages (right)
GEMLI identifies cell lineages in primary human tumors
The team also applied GEMLI to primary human breast cancer samples, where alternative lineage identification methods cannot be used. “GEMLI works best at reconstructing small to medium-sized lineages (about 30-50 cells), allowing to zoom into branching points during cancer progression,” says David Suter. “By identifying cells at the transition point from an in situ to an invasive phenotype, one can recover genes that potentially drive cancer progression.
In summary, GEMLI works by identifying and leveraging memory genes within a vast sea of genetic information, using them as breadcrumbs to trace the lineage of cells. By analyzing the subtle nuances in gene expression, GEMLI reveals how cells relate to each other.
GEMLI does not require specialized equipment or any changes to standard laboratory practices, is freely available at https://github.com/UPSUTER/GEMLI, and allows lineage identification from virtually any standard scRNA-seq dataset. “We are excited about GEMLI’s potential in leveraging the large number of publicly-available human cancer scRNA-seq datasets to dissect how other types of cancers switch to an invasive phenotype,” says Suter.
Other contributors
Karolinska Institute
Swiss National Science Foundation
Novartis Foundation for Medical-Biological Research
OE och Edla Johanssons Research Foundation
The Swedish Research Council
Knut and Alice Wallenberg Foundation
Ragnar Söderberg Foundation
A.S. Eisele, M. Tarbier, A.A. Dormann, V. Pelechano, D.M. Suter. Gene-expression memory-based prediction of cell lineages from scRNA-seq datasets. Nature Communications 29 March 2024. DOI: 10.1038/s41467-024-47158-y