A new tool for protein sequence generation and design
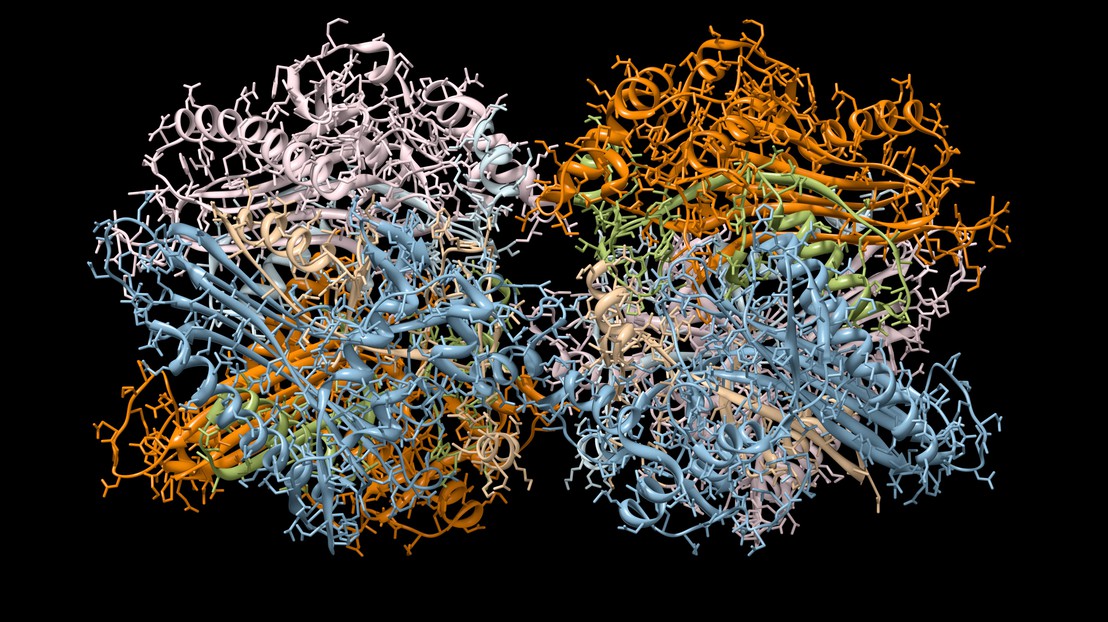
iStock photos
EPFL researchers have developed a new technique that uses a protein language model for generating protein sequences with comparable properties to natural sequences. The method outperforms traditional models and offers promising potential for protein design.
Designing new proteins with specific structure and function is a highly important goal of bioengineering, but the vast size of protein sequence space makes the search for new proteins difficult. However, a new study by the group of Anne-Florence Bitbol at EPFL's School of Life Sciences has found that a deep-learning neural network, MSA Transformer, could be a promising solution.
Developed in 2021, MSA Transformer works in a similar way to natural language processing, used by the now famous ChatGPT. The team, composed of Damiano Sgarbossa, Umberto Lupo, and Anne-Florence Bitbol, proposed and tested an “iterative method”, which relies on the ability of the model to predict missing or masked parts of a sequence based on the surrounding context.
The team found that through this approach, MSA Transformer can be used for generating new protein sequences from given protein “families” (groups of proteins with similar sequences), with similar properties to natural sequences.
In fact, protein sequences generated from large families with many homologs had better or similar properties than sequences generated by Potts models. “A Potts model is an entirely different type of generative model not based on natural language processing or deep learning, which was recently experimentally validated,” explains Bitbol. “Our new MSA Transformer-based approach allowed us to generate proteins even from small families, where Potts models perform poorly.”
The MSA Transformer reproduces the higher-order statistics and the distribution of sequences in natural data more accurately than other models, which makes it a strong candidate for protein sequence generation and protein design.
“This work can lead to the development of new proteins with specific structures and functions; such approaches will hopefully enable important medical applications in the future,” says Bitbol. “The potential of the MSA Transformer as a strong candidate for protein design provides exciting new possibilities for the field of bioengineering.”
The study is published in eLife, whose editors commented: “This important study proposes a method to sample novel sequences from a protein language model that could have exciting applications in protein sequence design. The claims are supported by a solid benchmarking of the designed sequences in terms of quality, novelty and diversity.”
European Research Council (ERC) (Horizon 2020)
Damiano Sgarbossa, Umberto Lupo, Anne-Florence Bitbol. Generative power of a protein language model trained on multiple sequence alignments. eLife 03 February 2023. DOI: 10.7554/eLife.79854