A New Tool For Optimizing Delinquent Debt Collection Strategies
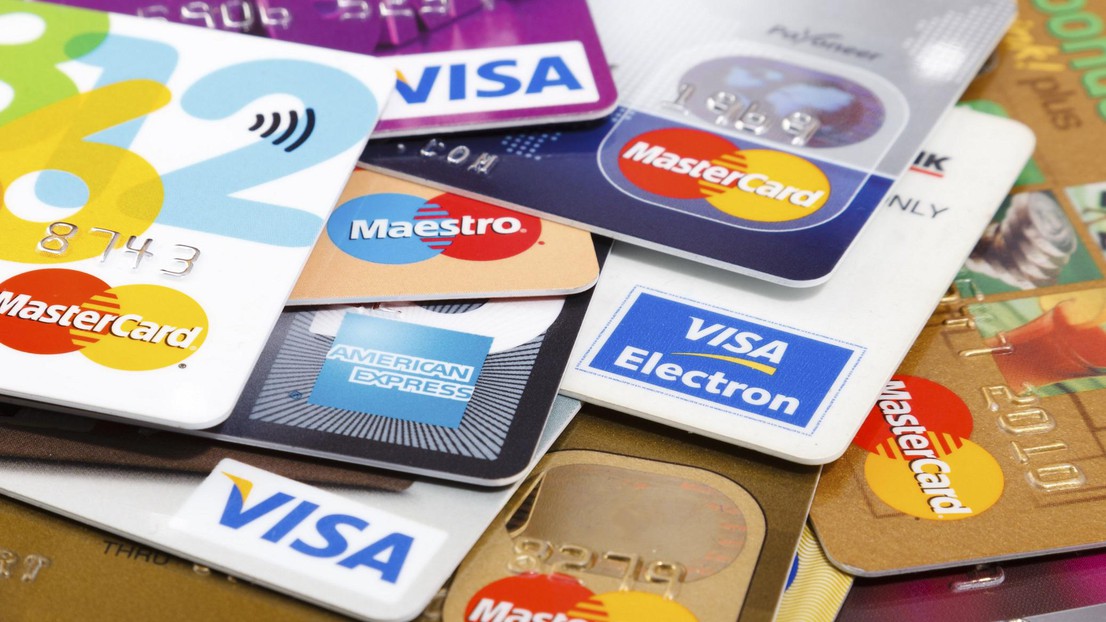
© istock.com
Traditional methods of scoring outstanding credit accounts and the associated collection strategies are relatively ineffective. Thomas Weber and his research colleagues Naveed Chehrazi and Peter W. Glynn have created a new Dynamic Collectability Scoring methodology, and further refined the model to provide a way for organizations to optimize delinquent debt collection strategies.
Collecting outstanding unsecured consumer debt is a major challenge for many financial institutions. The size of the challenge is clear from the statistics. In the US in 2017, for example, aggregate revolving consumer credit totalled over $1 trillion. An average delinquency rate of 2.2% (according to the Federal Reserve Bank in 2018), means that the pool of delinquent debt was over $20 billion, highlighting the exposure of issuing banks to nonperforming loans, and the importance of maximizing collection yields.
Traditionally, many financial institutions have offloaded the unsecured consumer debt problem to investors that specialize in debt, or outsourced the outstanding debt problem to credit collection firms. Even so, despite the use of increasingly sophisticated techniques, optimal strategies for collecting outstanding payment remain elusive.
Dynamic Collectability Scoring
This is where the work of academic Professor Thomas Weber at EPFL and his colleagues is proving invaluable, building on earlier work to construct a Dynamic Collectability Scoring (the DCS) methodology - a more effective approach to dealing with credit card delinquency. The DCS is founded on a mathematical construct called a self-exciting point process, where the occurrence of past events makes the occurrence of future events - in this case debt repayments - more probable, at least in the short run. Its use in finance is comparatively recent and in the context of credit collection Weber and his colleagues are pioneering developments.
Banks traditionally assign pre-computed scores to outstanding accounts that attempt to reflect a range of factors affecting collection, such as: account specific data about the debtor such as legal status, other loans and credit lines, credit rating (FICO score), repayment history and demographic information; and macroeconomic indicators including interest and inflation rates, and general economic performance. However, such static scoring methods are often poor predictors of repayment behavior, with Weber's dynamic DCS model proving far more effective.
Identifying the optimal collection strategy
One challenge for the banks, debt investors, and collection agencies is determining the optimal strategy, the actions and level of resources that should be utilized in chasing a particular outstanding debt as a function of the current account state. Further work on the DCS open box model allows organizations to determine the optimal collection actions to deploy at any given state of the account (in terms of its balance and payment intensity – the frequency of payments) along the debt collection journey, over time.
Using the model it is possible to find interventions that will produce the optimal repayment intensity, factoring in the relative cost of different account-treatment actions. An optimal collection strategy can be visualized in an (intensity, balance) - space, which contains the account state, and that is split into an action region and a continuation region. Once the account enters the action region it is optimal for a collection action to start so as to increase the likelihood of repayment, whereas in the continuation region it is best for the collector to wait. This policy minimizes the expected total loss (in terms of lost outstanding balance and collection cost). It also enables the collector of a debt to gauge whether an outstanding account is below a "minimally actionable balance" – a point at which best not to apply any costly account-treatment actions, such as engaging lawyers, for example.
To implement the DCS model organizations will go through a model identification process, running the model depending on the type of actions they are going to use, determining how sensitive the debtor is to those actions, then recalibrating the model as they proceed. They might, for example, test against three months of data, applying what they believe is the optimal policy, then recalibrating against the graph using the model. It is normal for organizations to use existing data to train the model and test the model's predicted outcomes against actual outcomes.
Deployed correctly the model provides a policy to minimize the total loss accrued from any outstanding payment, providing a higher return and lower variance of that return compared with not using the model. The model can even be used to validate the use of AI to facilitate the collection of payments.
The work of Weber and his colleagues has a number of important implications beyond debt collection. There are regulatory implications, for example, as regulators will have a more accurate means of determining the value of non-performing loans, and thus a bank's capital reserves. The model also allows financial institutions to value portfolios of non-performing loans more accurately, which in turn has implications for both the sale of such portfolios and valuation of the financial institution.
And the techniques used by Weber and built into the model are not just useful in the context of finance. Any series of events or phenomenon which exhibits self-exciting point process behavior (seismology is just one of many non-finance related fields) can utilize the model. Theoretically they would be able to determine the best strategies to bring about a series of events in the optimal way. The most immediate application, however, is likely to be enabling organizations to enhance their balance sheets by optimizing their collection of outstanding payments.